DWT-CompCNN: deep image classification network for high throughput JPEG 2000 compressed documents
CoRR(2023)
摘要
For any digital application with document images such as retrieval, the classification of document images becomes an essential stage. Conventionally for the purpose, the full versions of the documents, that is the uncompressed document images make the input dataset, which poses a threat due to the big volume required to accommodate the full versions of the documents. Therefore, it would be novel, if the same classification task could be accomplished directly (with some partial decompression) with the compressed representation of documents in order to make the whole process computationally more efficient. In this research work, a novel deep learning model—DWT-CompCNN—is proposed for classification of documents that are compressed using High Throughput JPEG 2000 (HTJ2K) algorithm. The proposed DWT-CompCNN comprises of five convolutional layers with filter sizes of 16, 32, 64, 128, and 256 consecutively for each increasing layer to improve learning from the wavelet coefficients extracted from the compressed images. Experiments are performed on two benchmark datasets, Tobacco-3482 and RVL-CDIP, which demonstrate that the proposed model is time and space efficient, and also achieves a better classification accuracy in compressed domain.
更多查看译文
关键词
DWT-CompCNN,Document classification,Compressed document version,JPEG 2000,Discrete wavelet transform,Deep learning in compressed domain
AI 理解论文
溯源树
样例
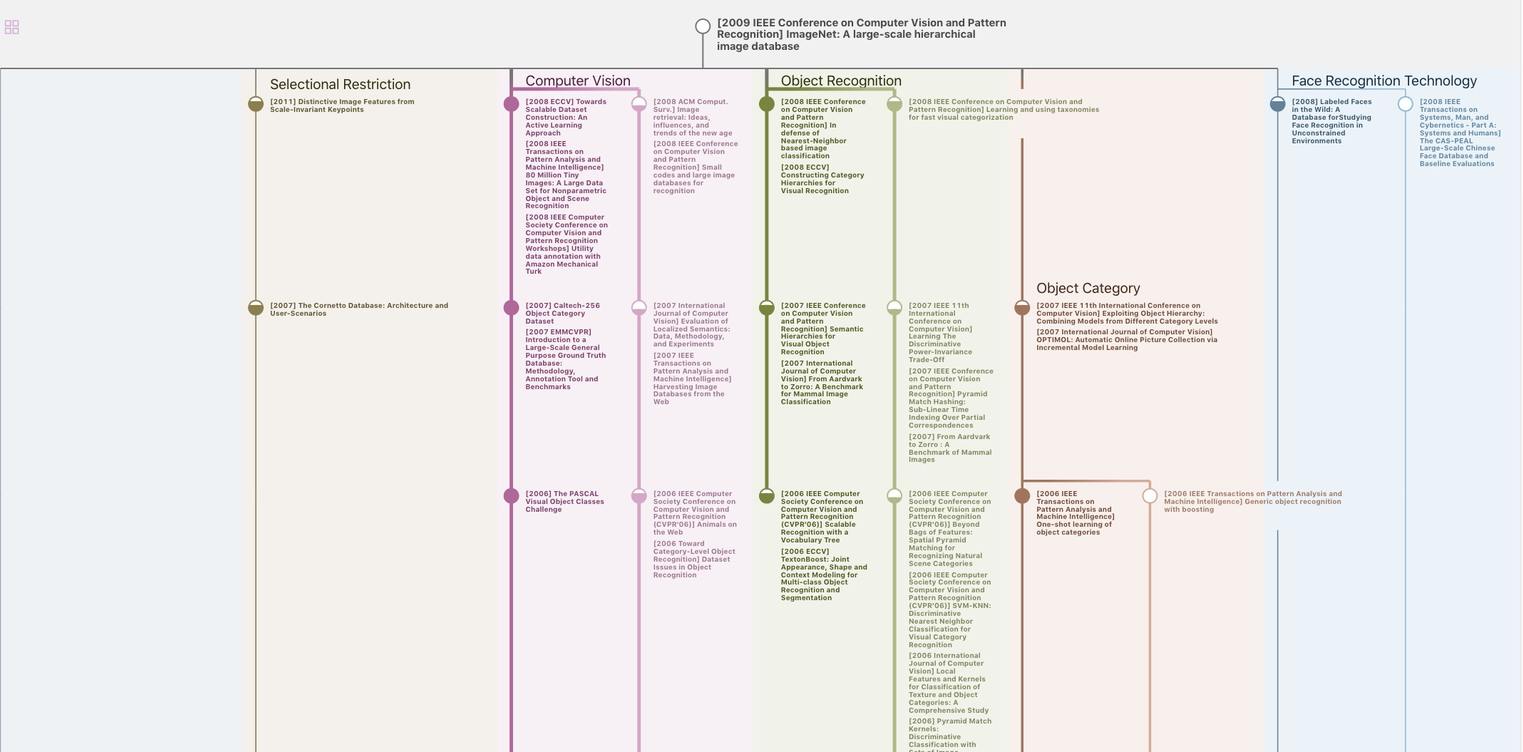
生成溯源树,研究论文发展脉络
Chat Paper
正在生成论文摘要