Pedestrian Crossing Action Recognition and Trajectory Prediction with 3D Human Keypoints
2023 IEEE INTERNATIONAL CONFERENCE ON ROBOTICS AND AUTOMATION, ICRA(2023)
摘要
Accurate understanding and prediction of human behaviors are critical prerequisites for autonomous vehicles, especially in highly dynamic and interactive scenarios such as intersections in dense urban areas. In this work, we aim at identifying crossing pedestrians and predicting their future trajectories. To achieve these goals, we not only need the context information of road geometry and other traffic participants but also need fine-grained information of the human pose, motion and activity, which can be inferred from human keypoints. In this paper, we propose a novel multi-task learning framework for pedestrian crossing action recognition and trajectory prediction, which utilizes 3D human keypoints extracted from raw sensor data to capture rich information on human pose and activity. Moreover, we propose to apply two auxiliary tasks and contrastive learning to enable auxiliary supervisions to improve the learned keypoints representation, which further enhances the performance of major tasks. We validate our approach on a large-scale in-house dataset, as well as a public benchmark dataset, and show that our approach achieves state-of-the-art performance on a wide range of evaluation metrics. The effectiveness of each model component is validated in a detailed ablation study.
更多查看译文
AI 理解论文
溯源树
样例
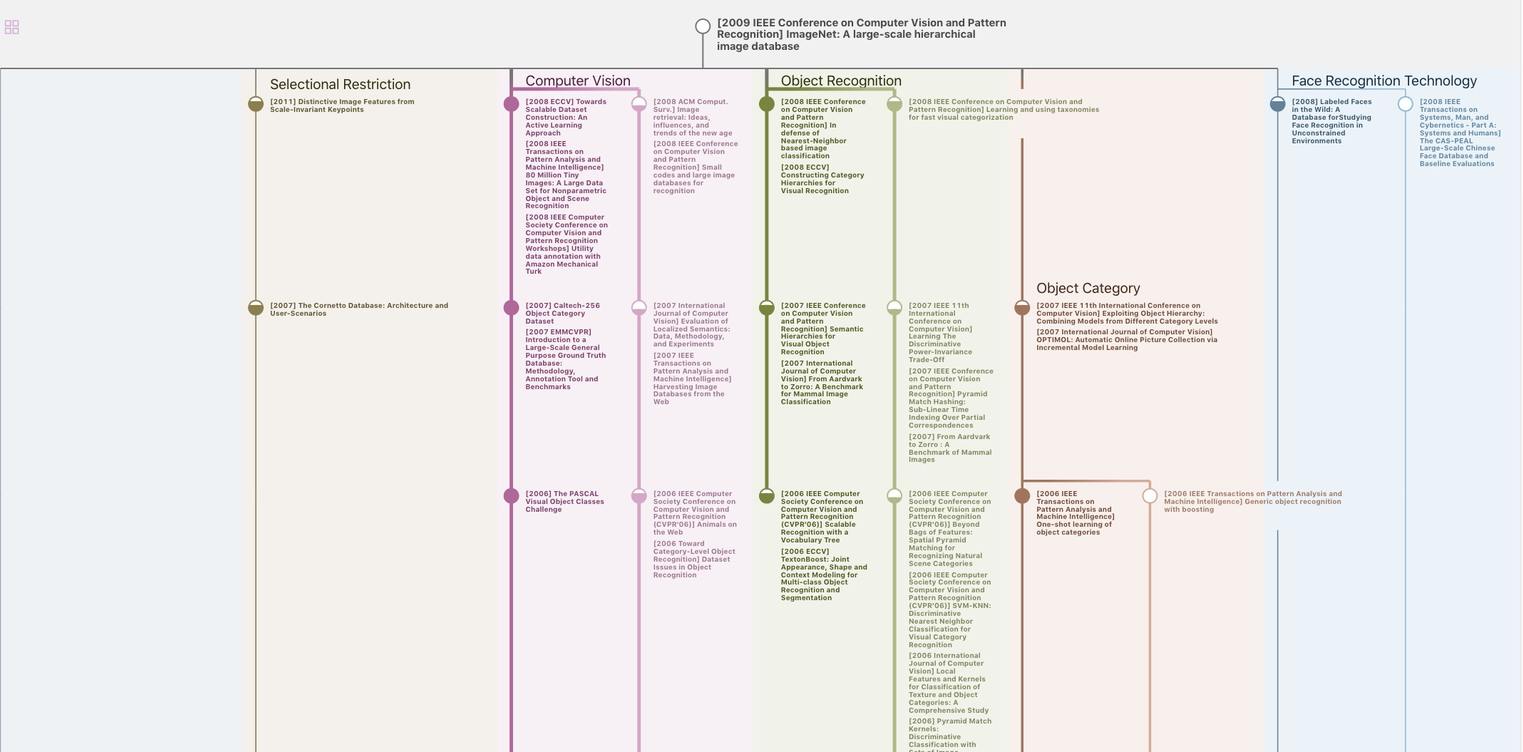
生成溯源树,研究论文发展脉络
Chat Paper
正在生成论文摘要