Poster: Lightweight Features Sharing for Real-Time Object Detection in Cooperative Driving
2023 IEEE Vehicular Networking Conference (VNC)(2023)
摘要
In model partitioning for real-time object detection, part of the model is deployed on a vehicle, and the remaining layers are processed in the cloud. Model partitioning requires transmitting intermediate features to the cloud, which can be problematic, given that the latency requirements are strict. This paper addresses this issue by demonstrating a lightweight feature-sharing strategy while investigating a trade-off between detection quality and latency. We report details on layer partitioning, such as which layers to split in order to achieve the desired accuracy.
更多查看译文
关键词
Real-time object detection, Neural network quantization, model compression, V2X Communication
AI 理解论文
溯源树
样例
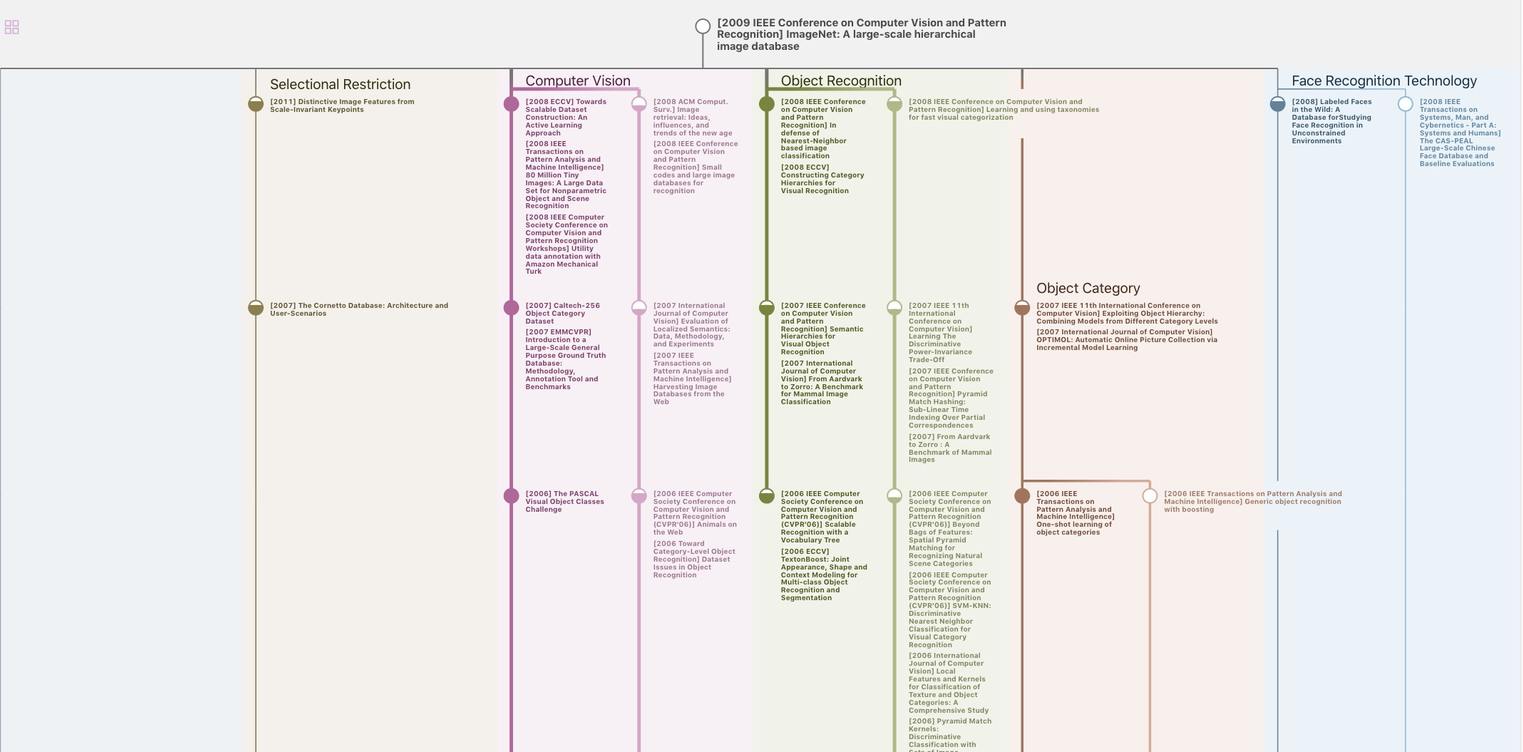
生成溯源树,研究论文发展脉络
Chat Paper
正在生成论文摘要