A Convolutional Neural Network for Parameter Estimation of the Bi-GTD Model
IEEE Transactions on Antennas and Propagation(2023)
摘要
In this article, a novel parameter estimation method based on a convolutional neural network (CNN) is proposed to extract the geometrical features of radar objects. The CNN’s design is inspired by the inversion process of a physically relevant model, called the geometrical theory of diffraction (GTD) model, whose bistatic form can be used to describe the bistatic scattering response from the target in the netted radar system. This model-inspired inversion method can automatically compensate for phase errors between multiple signal channels and obtain better parameter estimation performance than traditional methods, such as the orthogonal matching pursuit (OMP), the estimation of signal parameters via rotational invariance techniques (ESPRIT), and the multiple signal classification (MUSIC). The experimental results not only verify the validity of the proposed intelligent inversion method but also demonstrate the interpretability and generalization ability of the CNN, whose architecture is designed based on mathematical derivation.
更多查看译文
关键词
parameter estimation,convolutional neural network,model,bi-gtd
AI 理解论文
溯源树
样例
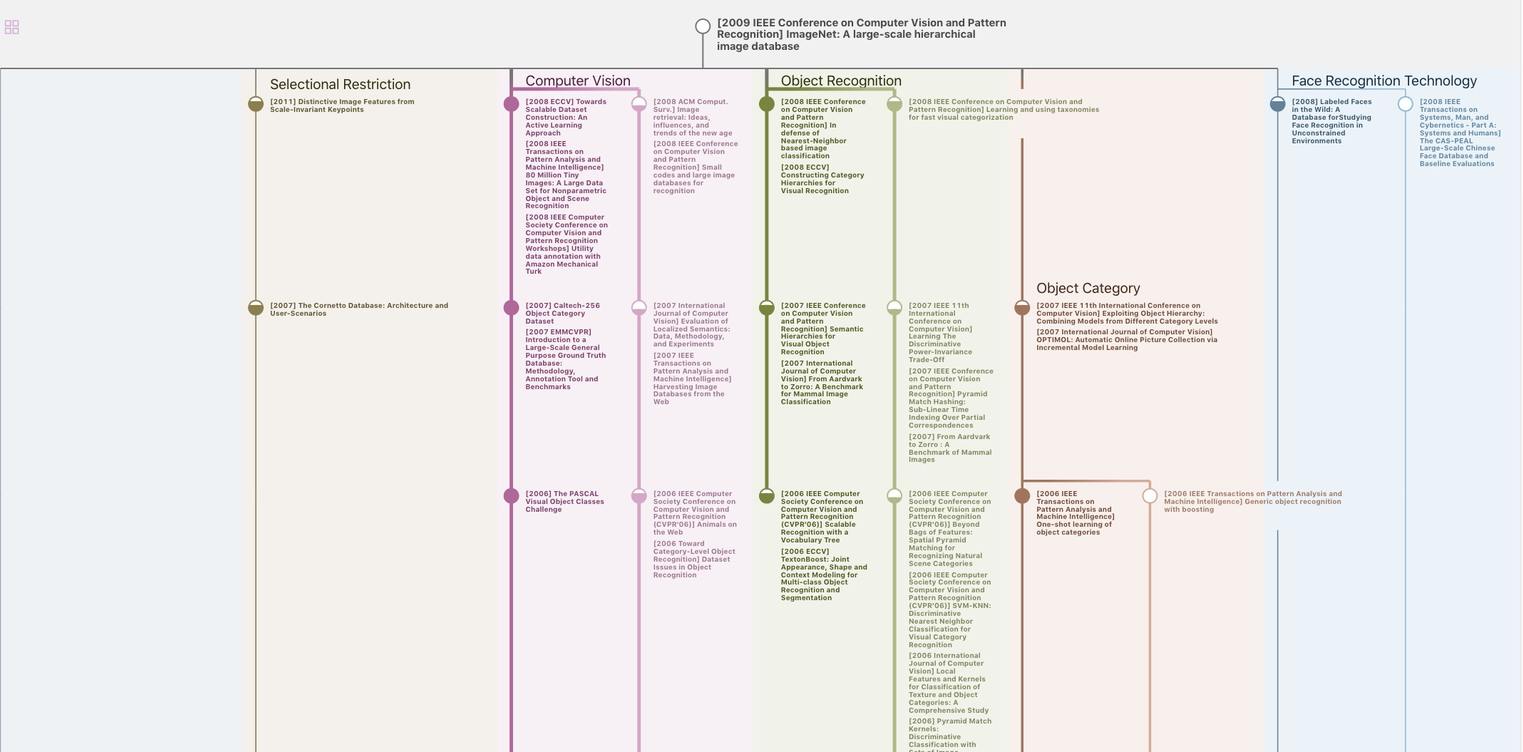
生成溯源树,研究论文发展脉络
Chat Paper
正在生成论文摘要