Detecting Coronavirus (COVID -19) Disease Cues from Chest Radiography Images.
ICVGIP(2022)
摘要
This paper proposes a deep learning-based approach to detect COVID-19 infections in lung tissues from chest Computed Tomography (CT) images. A two-stage classification model is designed to identify the infection from CT scans of COVID-19 and Community Acquired Pneumonia (CAP) patients. The proposed neural model named, Residual C-NiN uses a modified convolutional neural network (CNN) with residual connections and a Network-in-Network (NiN) architecture for COVID-19 and CAP detection. The model is trained with the Signal Processing Grand Challenge (SPGC) 2021 COVID dataset. The proposed neural model achieves a slice-level classification accuracy of 93.54% on chest CT images and patient-level classification accuracy of 86.59% with class-wise sensitivity of 92.72%, 55.55%, and 95.83% for COVID-19, CAP, and Normal classes, respectively. Experimental results show the benefit of adding NiN and residual connections in the proposed neural architecture. Experiments conducted on the dataset show significant improvement over the existing state-of-the-art methods reported in the literature.
更多查看译文
AI 理解论文
溯源树
样例
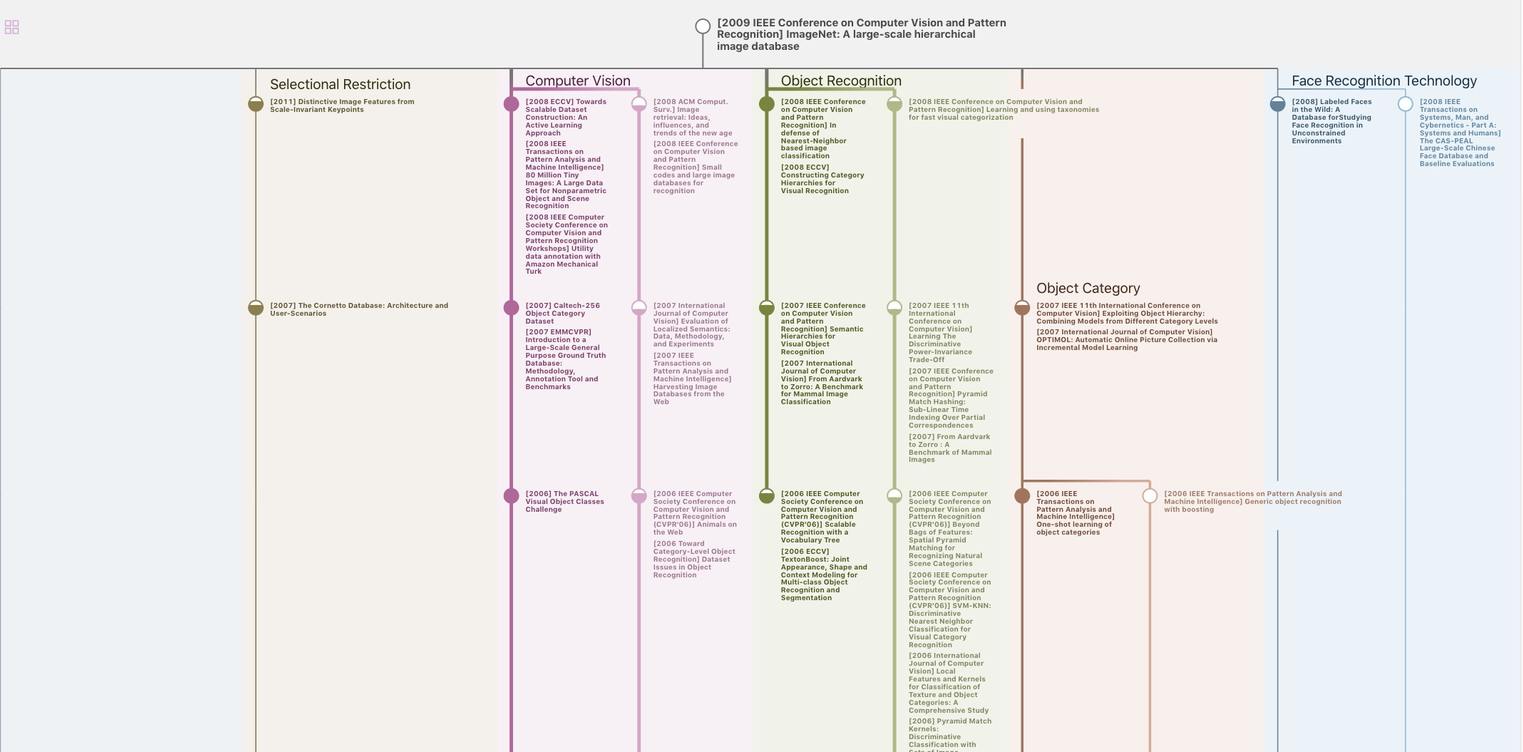
生成溯源树,研究论文发展脉络
Chat Paper
正在生成论文摘要