Generating live commentary for marine traffic scenarios based on multi-model learning.
Comput. Commun.(2023)
摘要
The Internet of Things (IoT), which allows for seamless communication and interaction with an array of smart devices, has rapidly become an indispensable part of our daily lives. Maritime transportation is one of the primary modes of transportation that heavily relies on Internet of Things (IoT) technologies to obtain crucial information such as atmospheric, oceanographic, navigation, and state data. By utilizing these IoT technologies, marine traffic scenarios can be constructed to obtain a comprehensive understanding of the traffic flow. Having a thorough understanding of marine traffic scenarios is crucial for improving the efficiency and safety of maritime transportation. Due to the time lag and data incompleteness inherent in marine traffic broadcasting and manual marine monitoring systems, it can be challenging to rely on them entirely. Meanwhile, the required resource to analyze marine traffic scenarios can be enormous. To address these challenges,we propose an approach based on multi-model learning for the automatic interpretation of marine traffic scenarios and developed an innovative dataset of multi-model marine traffic scenarios. Specifically, we have aligned maritime meteorological data with existing marine video data to create a comprehensive dataset. This dataset includes both raw data and manually created professional annotations to address the lack of benchmark datasets for multi-modal marine traffic scenarios. In addition to the dataset, we have developed a text generation model based on a multi-modal Transformer architecture. This model extracts the characteristics of multi-scale objects from visual marine frames by a parallel convolution mechanism, converts meteorological indexes into post-numeric codes, and combines the coding results of each mode through a multi-head self-attention mechanism to form fine-grained interpretation text. To train our text generation model for marine traffic scenarios, the threshold cross-entropy loss function is used to compensate the class imbalance. Our experimental results demonstrate the effectiveness of our approach, showing that our model can efficiently generate accurate and informative descriptions of maritime activity.
更多查看译文
关键词
Commentary generation, Multi-model, Spatio-temporal, Internet of Things, Marine traffic scenarios
AI 理解论文
溯源树
样例
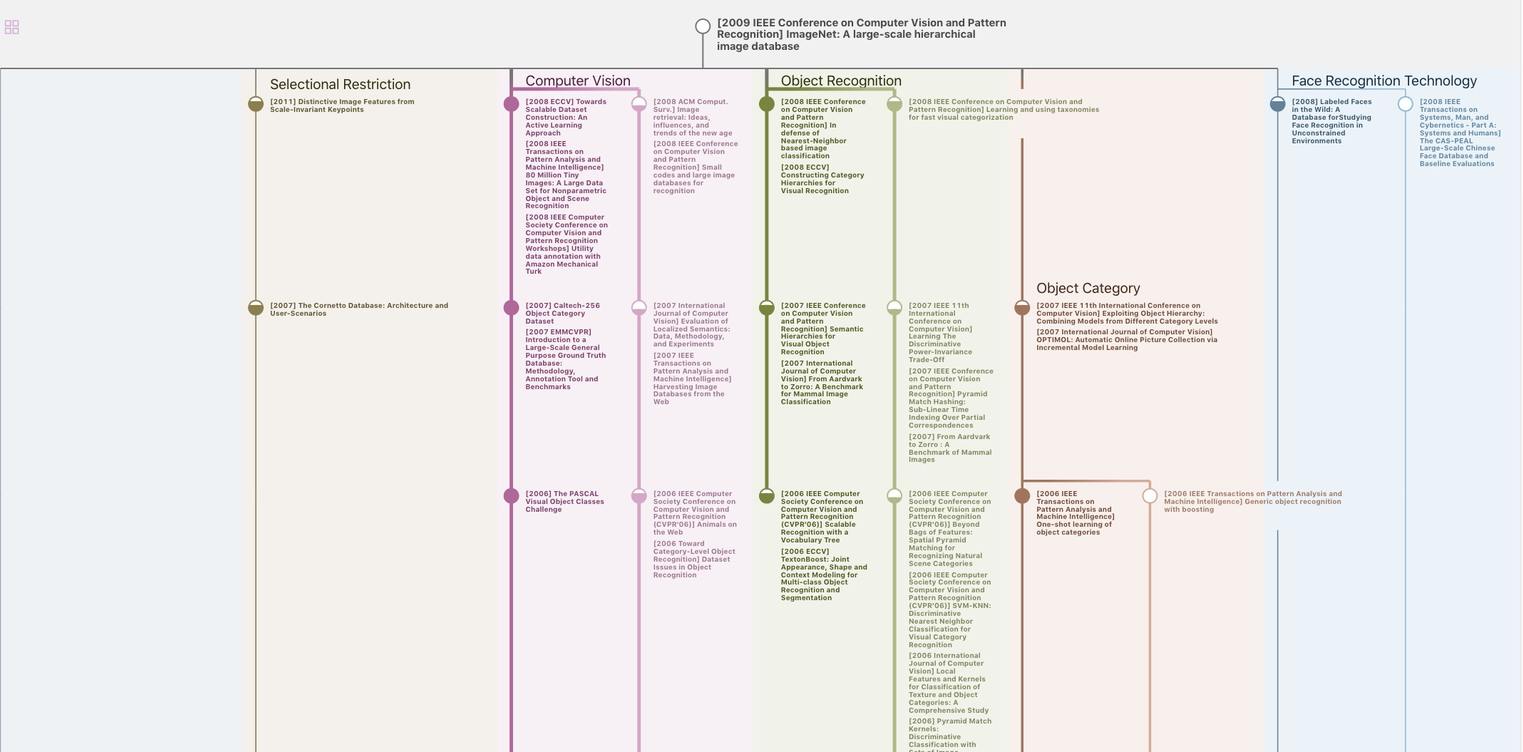
生成溯源树,研究论文发展脉络
Chat Paper
正在生成论文摘要