Data augmentation to improve syndromic detection from emergency department notes
ACSW(2023)
摘要
Using natural language processing algorithms requires a large amount of annotated data, which is difficult to obtain in clinical settings. In this work we aimed to develop and evaluate data augmentation methods to enhance the performance of classification tasks implemented in a medical domain. Three text augmentation methodswere evaluated for generating febrile convulsion-like emergency department (ED) presentations. These were synthetic text generation, domain-specific data augmentation, and task-agnostic data augmentation techniques. We used a transformer-based classifier, first establishing a baseline by training with non-augmented data, then training on each set of augmented data to compare the results with the baseline. The synthetic text generation augmentation method was clearly superior, with the f-score improving by 12% compared with the baseline. The domain-specific approach improved the score by 6%, while the task-agnostic augmentation method failed to enhance classification performance. Our study shows that data augmentation methods can be used for generating artificial ED data to enhance classification of critical syndromes, thereby assisting syndromic surveillance to contribute to improving public health.
更多查看译文
关键词
Chief complaints, triage notes, emergency, epidemic intelligence, data augmentation, syndromic surveillance
AI 理解论文
溯源树
样例
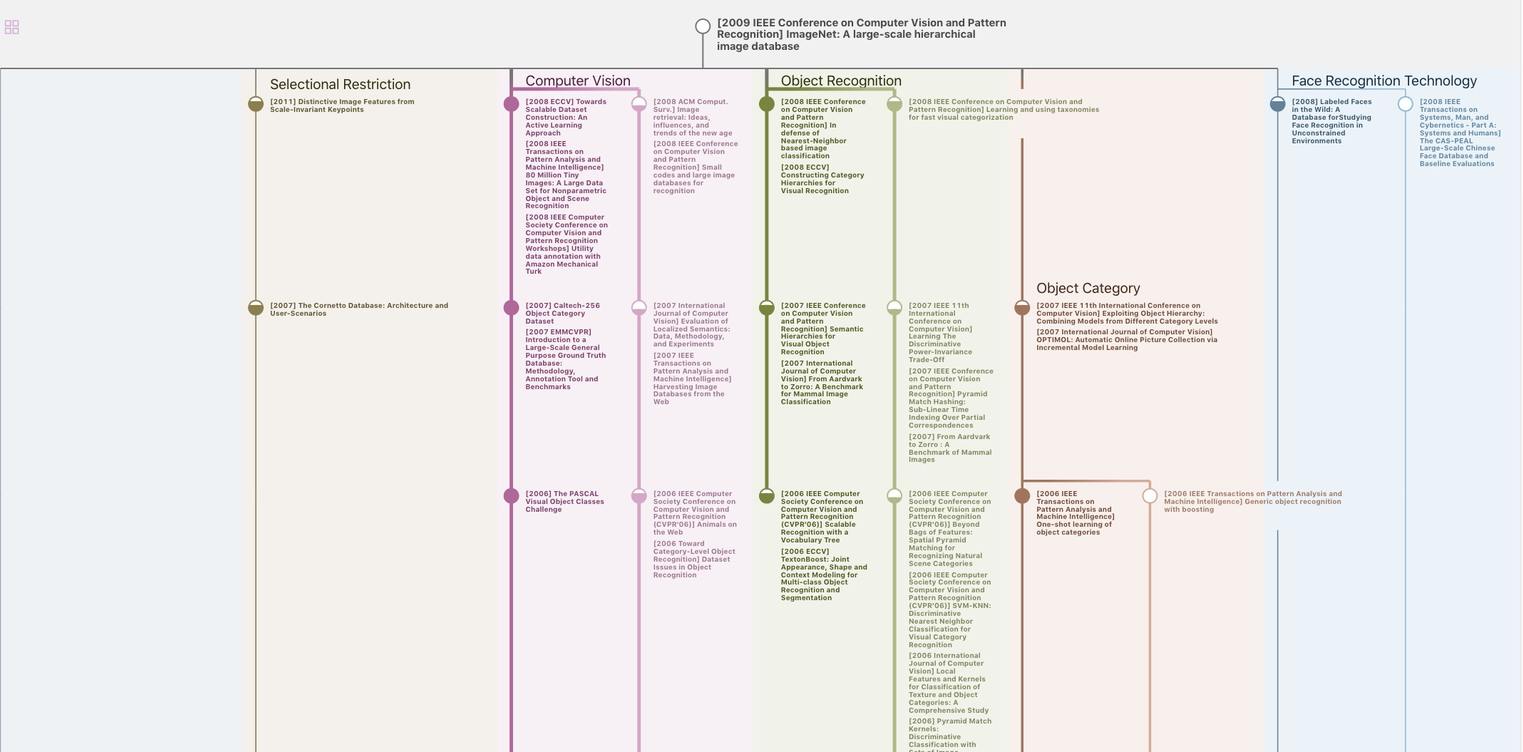
生成溯源树,研究论文发展脉络
Chat Paper
正在生成论文摘要