Learning Local Similarity with Spatial Interrelations on Content-Based Image Retrieval
IEICE Trans. Inf. Syst.(2023)
摘要
Convolutional Neural Networks (CNNs) have recently demonstrated outstanding performance in image retrieval tasks. Local convolutional features extracted by CNNs, in particular, show exceptional capability in discrimination. Recent research in this field has concentrated on pooling methods that incorporate local features into global features and assess the global similarity of two images. However, the pooling methods sacrifice the image's local region information and spatial relationships, which are precisely known as the keys to the robustness against occlusion and viewpoint changes. In this paper, instead of pooling methods, we propose an alternative method based on local similarity, determined by directly using local convolutional features. Specifically, we first define three forms of local similarity tensors (LSTs), which take into account information about local regions as well as spatial relationships between them. We then construct a similarity CNN model (SCNN) based on LSTs to assess the similarity between the query and gallery images. The ideal configuration of our method is sought through thorough experiments from three perspectives: local region size, local region content, and spatial relationships between local regions. The experimental results on a modified open dataset (where query images are limited to occluded ones) confirm that the proposed method outperforms the pooling methods because of robustness enhancement. Furthermore, testing on three public retrieval datasets shows that combining LSTs with conventional pooling methods achieves the best results.
更多查看译文
关键词
image retrieval,convolutional neural network,deep local features,local similarity,4D convolution,spatial correlation
AI 理解论文
溯源树
样例
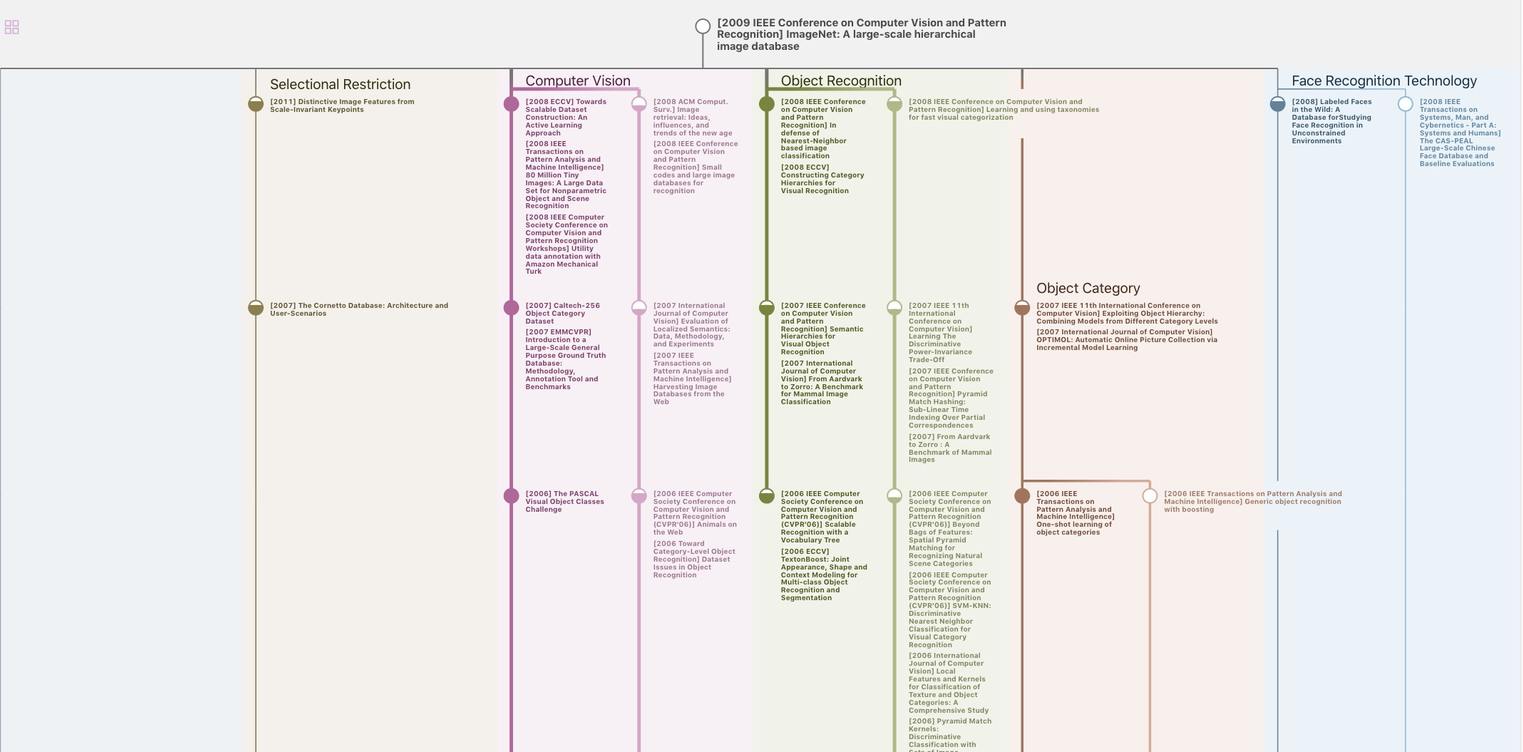
生成溯源树,研究论文发展脉络
Chat Paper
正在生成论文摘要