Latent-Space Disentanglement with Untrained Generator Networks for the Isolation of Different Motion Types in Video Data.
SSVM(2023)
摘要
Isolating different types of motion in video data is a highly relevant problem in video analysis. Applications can be found, for example, in dynamic medical or biological imaging, where the analysis and further processing of the dynamics of interest is often complicated by additional, unwanted dynamics, such as motion of the measurement subject. In this work, it is empirically shown that a representation of video data via untrained generator networks, together with a specific technique for latent space disentanglement that uses minimal, one-dimensional information on some of the underlying dynamics, allows to efficiently isolate different, highly non-linear motion types. In particular, such a representation allows to freeze any selection of motion types, and to obtain accurate independent representations of other dynamics of interest. Obtaining such a representation does not require any pre-training on a training data set, i.e., all parameters of the generator network are learned directly from a single video.
更多查看译文
关键词
untrained generator networks,different motion types,latent-space latent-space
AI 理解论文
溯源树
样例
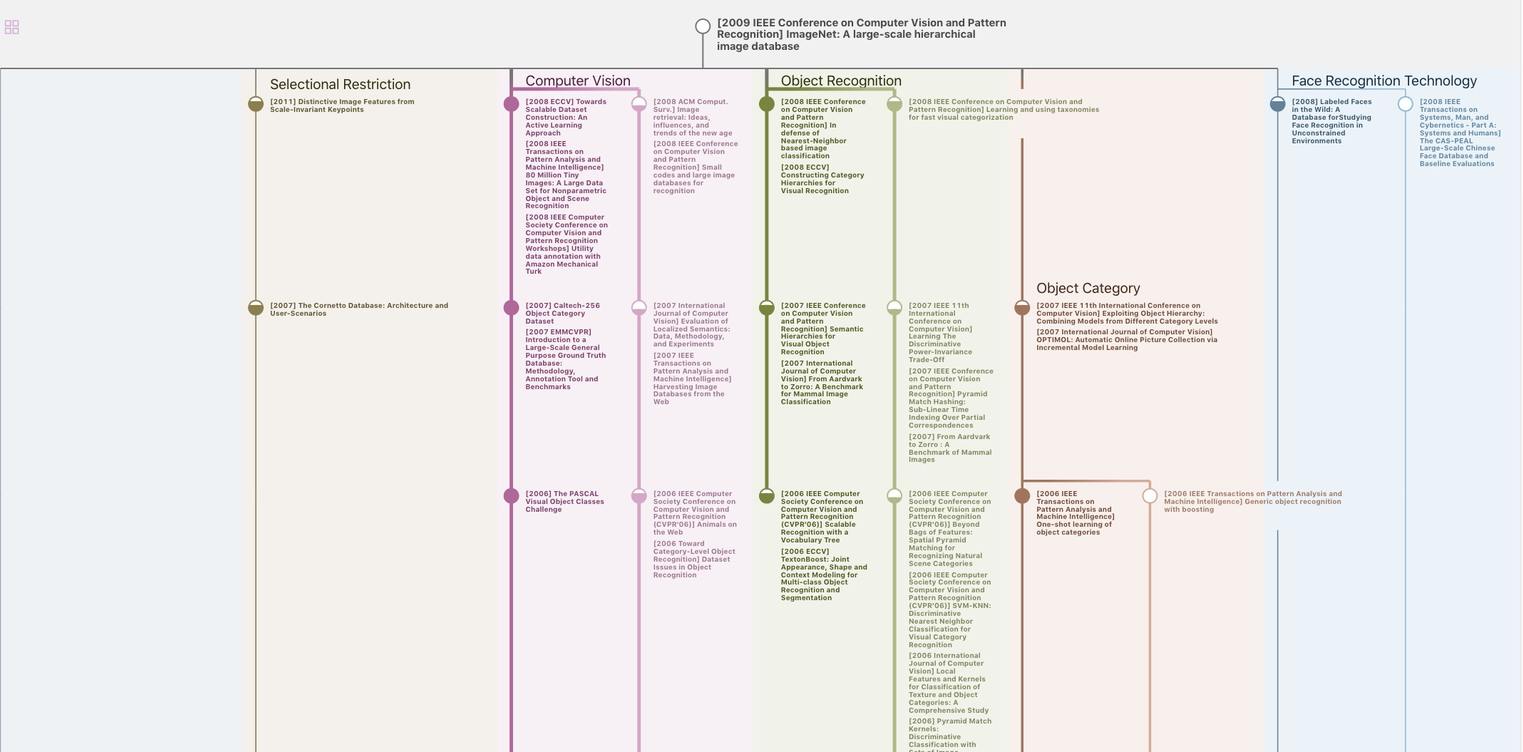
生成溯源树,研究论文发展脉络
Chat Paper
正在生成论文摘要