MBAHIL: Design of a Multimodal Hybrid Bioinspired Model for Augmentation of Hyperspectral Imagery via Iterative Learning for Continuous Efficiency Enhancements
IEEE Access(2023)
摘要
The augmentation of hyperspectral images requires the design of high-density feature analysis & band-fusion models that can generate multimodal imagery from limited information sets. The feature analysis models use deep learning operations to maximize inter-class variance while minimizing inter-class variance levels for efficient classification operations. When combined with intelligent band-fusion methods, such models allow the augmentation model to enhance its classification efficiency under different use cases. Existing band-fusion-based augmentation models for hyperspectral images do not incorporate continuous efficiency enhancements and showcase higher complexity levels. Furthermore, these models can't be scaled for more varied use cases because their use is restricted to specific image types. To overcome these issues, we designed a novel multimodal hybrid bioinspired model for the augmentation of hyperspectral imagery via iterative learning for continuous efficiency enhancements. The proposed model initially represents input images into Fourier, Laplacian, Cosine, multimodal Wavelet, Mellin, and Z-Transform domains, which will assist in describing the images in multimodal domains. These transformed image sets are passed through a convolutional filter to extract windowed feature sets. A Grey Wolf Optimizer (GWO) is used to identify high inter-class variance features from the extracted image sets, which assists in selecting transformed images that can help improve hyperspectral classification performance. The selected hyperspectral images are fused via a Bacterial Foraging Optimization (BFO) model, which assists in reducing intra-class variance levels. The final set of selected images is intelligently augmented via Particle Swarm Optimization (PSO), which performs rotation, zooming, shifting, and brightness variation operations selectively. These augmented images are classified via a customized VGGNet-19-based Convolutional Neural Network (CNN) classifier that assists in continuously estimating accuracy levels for different application scenarios. Based on these accuracy levels, the model is reconfigured via hyperparameter tuning of GWO and PSO optimizers. Due to combining these models and incremental accuracy optimizations, the proposed model has improved its hyperspectral classification accuracy by 10.6% and precision by 10.4%, as compared to standard deep learning-based augmentation techniques.
更多查看译文
关键词
Hyperspectral imaging,Biological system modeling,Convolutional neural networks,Feature extraction,Data models,Generative adversarial networks,Complexity theory,Particle swarm optimization,Bacterial foraging optimization,grey wolf optimizer,hyperspectral images,particle swarm optimization,VGGNet-19
AI 理解论文
溯源树
样例
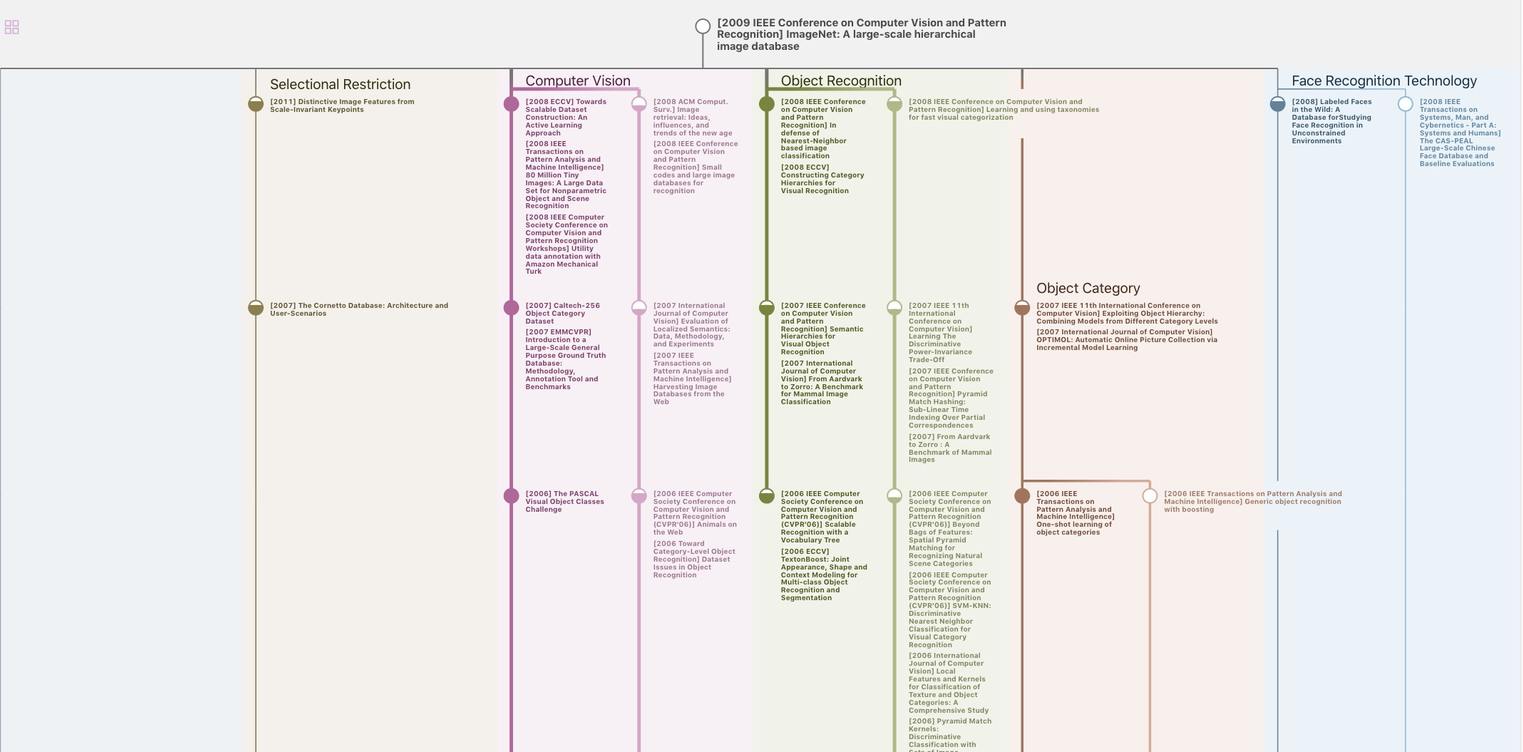
生成溯源树,研究论文发展脉络
Chat Paper
正在生成论文摘要