Semantic-Aware Region Loss for Land-Cover Classification
IEEE J. Sel. Top. Appl. Earth Obs. Remote. Sens.(2023)
摘要
Integrating superpixel segmentation into convolutional neural networks is known to be effective in enhancing the accuracy of land-cover classification. However, most of existing methods accomplish such integration by focusing on the development of new network architectures, which suffer from several flaws: conflicts between general superpixels and semantic labels introduce noise into the training, especially at object boundaries; absence of training guidance for superpixels leads to ineffective regional feature learning; and unnecessary superpixel segmentation in the testing stage not only increases the computational burden but also incurs jagged edges. In this study, we propose a novel semantic-aware region (SARI) loss to guide the effective learning of regional features with superpixels for accurate land-cover classification. The key idea of the proposed method is to reduce the feature variance inside and between homogeneous superpixels while enlarging feature discrepancy between heterogeneous ones. The SARI loss is thus designed with three subparts, including superpixel variance loss, intraclass similarity loss and interclass distance loss. We also develop semantic superpixels to assist in the network training with SARI loss while overcoming the limitations of general superpixels. Extensive experiments on two challenging datasets demonstrate that the SARI loss can facilitate regional feature learning, achieving state-of-the-art performance with mIoU scores of around 97.11% and 73.99% on Gaofen Image dataset and DeepGlobe dataset, respectively.
更多查看译文
关键词
Semantics,Remote sensing,Training,Feature extraction,Semantic segmentation,Task analysis,Deep learning,land-cover classification,region loss,remote sensing
AI 理解论文
溯源树
样例
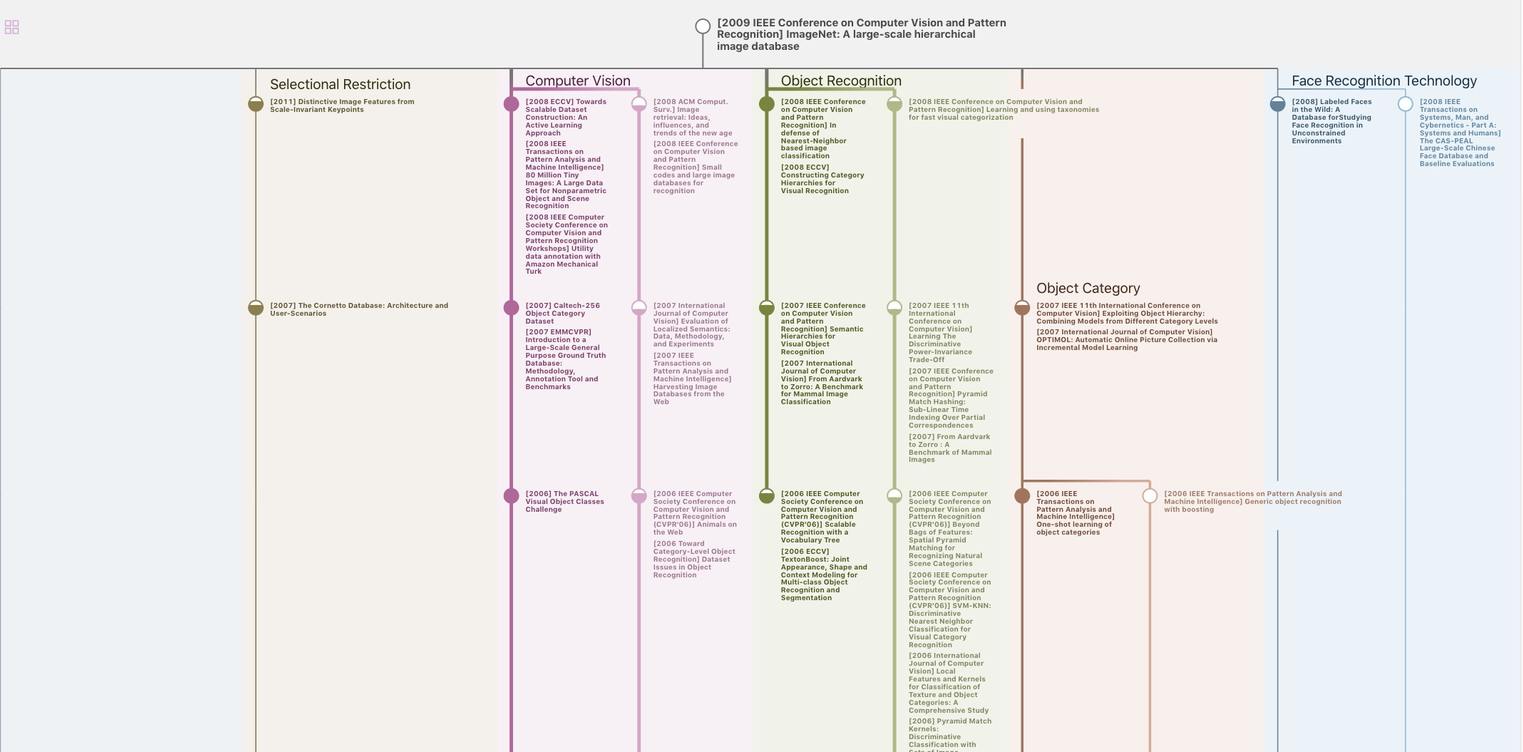
生成溯源树,研究论文发展脉络
Chat Paper
正在生成论文摘要