Rigorous non-disjoint discretization for naive Bayes
Pattern Recognit.(2023)
摘要
Naive Bayes is a classical machine learning algorithm for which discretization is commonly used to trans-form quantitative attributes into qualitative attributes. Of numerous discretization methods, Non-Disjoint Discretization (NDD) proposes a novel perspective by forming overlapping intervals and always locating a value toward the middle of an interval. However, existing approaches to NDD fail to adequately consider the effect of multiple occurrences of a single value - a commonly occurring circumstance in practice. By necessity, all occurrences of a single value fall within the same interval. As a result, it is often not possible to discretize an attribute into intervals containing equal numbers of training instances. Current methods address this issue in an ad hoc manner, reducing the specificity of the resulting atomic intervals. In this study, we propose a non-disjoint discretization method for NB, called Rigorous Non-Disjoint Discretiza-tion (RNDD), that handles multiple occurrences of a single value in a systematic manner. Our extensive experimental results suggest that RNDD significantly outperforms NDD along with all other existing state-of-the-art competitors.(c) 2023 The Author(s). Published by Elsevier Ltd. This is an open access article under the CC BY license (http://creativecommons.org/licenses/by/4.0/)
更多查看译文
关键词
naive bayes,non-disjoint
AI 理解论文
溯源树
样例
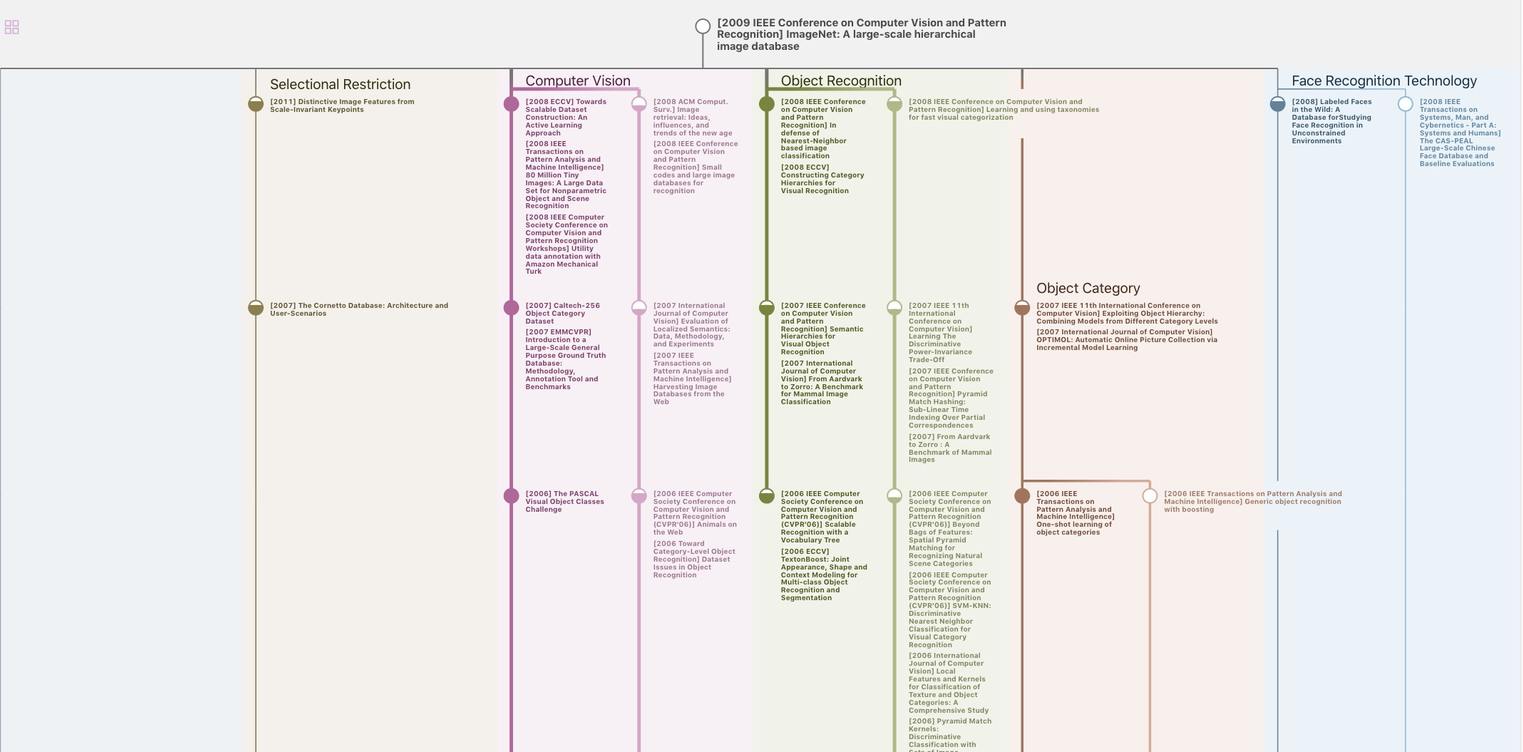
生成溯源树,研究论文发展脉络
Chat Paper
正在生成论文摘要