LabanFormer: Multi-scale graph attention network and transformer with gated recurrent positional encoding for labanotation generation.
Neurocomputing(2023)
摘要
Labanotation is a widely-used notation system for recording human dance movements. Automatically generating Labanotation scores from motion capture data can save significant manual effort and help the preservation of old folk dances in protecting intangible cultural heritages. Existing Labanotation gen-eration methods have limited ability to capture the flexible limb movements as well as the rich periodic, symmetric, or repeated dance steps. In this paper, we present a novel LabanFormer model including a Multi-Scale Graph Attention network (MS-GAT) and a transformer model with Gated Recurrent Positional Encoding (GRPE) to achieve more effective Labanotation generation. First, the proposed MS-GAT can capture flexible limb movements by learning feature correlations between every two joints and aggregating features of neighboring joints over multiple scales. Second, we propose a new GRPE-based transformer to learn global temporal dependencies in the output feature sequences of MS-GAT. The novel GRPE module can encode position information with learnable parameters while handling var-ious sequence lengths. As such, the periodic, symmetric, or repeated steps in dances can be accurately captured. Finally, the corresponding Laban symbols are generated by the decoder of the GRPE-based transformer. Extensive experiments on two real-world datasets show that the proposed LabanFormer model obtains remarkable performance compared with state-of-the-art approaches on the automatic Labanotation generation task. (c) 2023 Elsevier B.V. All rights reserved.
更多查看译文
关键词
labanotation generation,recurrent positional encoding,attention,graph,multi-scale
AI 理解论文
溯源树
样例
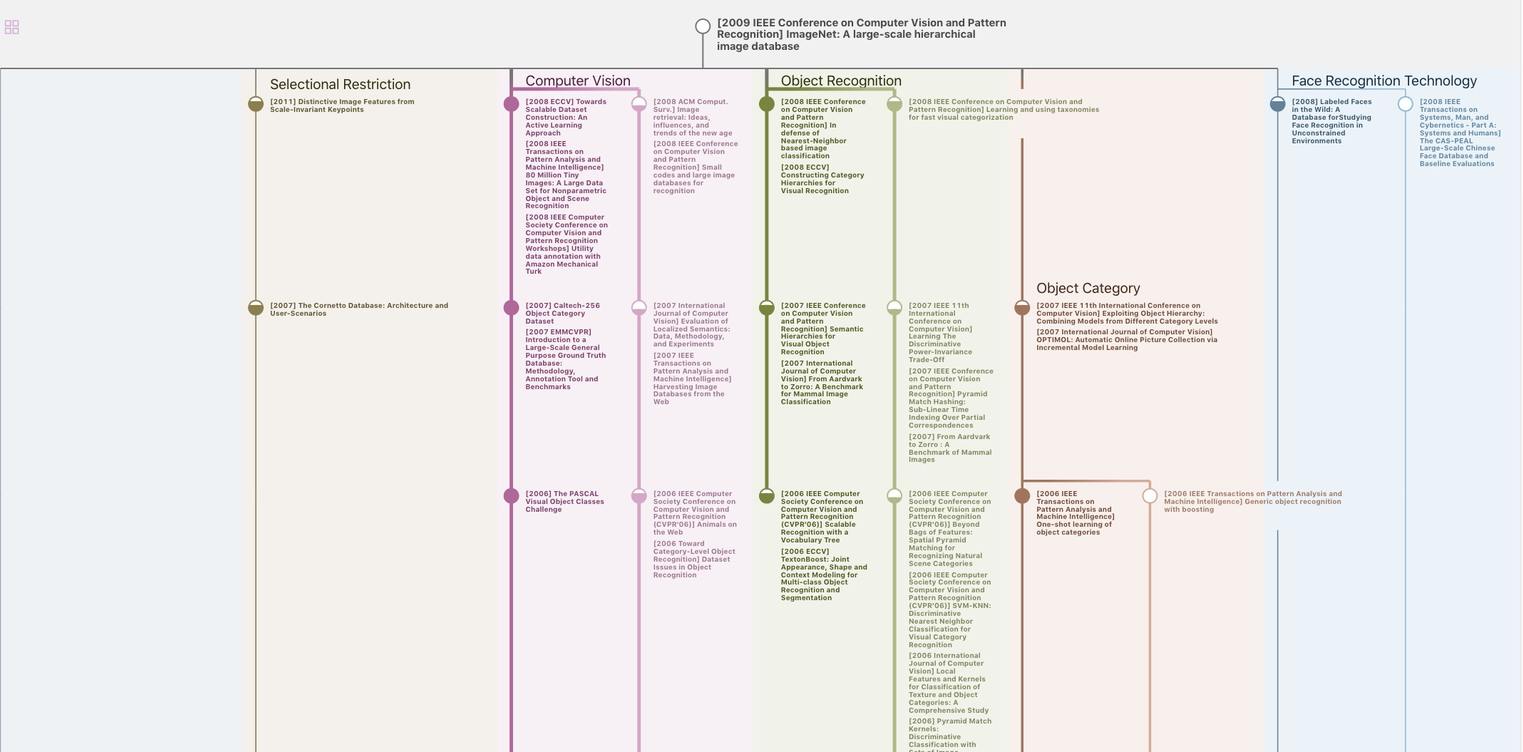
生成溯源树,研究论文发展脉络
Chat Paper
正在生成论文摘要