Incentivizing Information Gain in Hidden Information Multi-Action Games.
CG(2022)
摘要
Wargames often include fog of war, i.e. hidden information, and multi-action turns, where each turn requires making multiple, sequential action choices. These properties provide unique challenges for Artificial Intelligence agents. Extensions to Monte-Carlo Tree Search (MCTS) allow it to perform well in games with hidden information as well as multi-action games. However, these extensions do not specifically consider both properties simultaneously nor how information-gaining actions could improve agent performance. Information-gaining actions are important in multi-action turns where initial actions can reveal state information, thus improving later action decisions. This paper presents enhancements to MCTS that add an information gain incentive and a risk determinization to balance locating opponent pieces while minimizing exposure to enemy fire. The information gain incentive and risk functions are implemented in Perfect Information-MCTS (PIMCTS) and Information Set-MCTS (ISMCTS) and evaluated on the multi-action hidden information game TUBSTAP. Results show that these additions improve performance over the baseline algorithms and against a Cheating MCTS implementation.
更多查看译文
关键词
information gain,multi-action
AI 理解论文
溯源树
样例
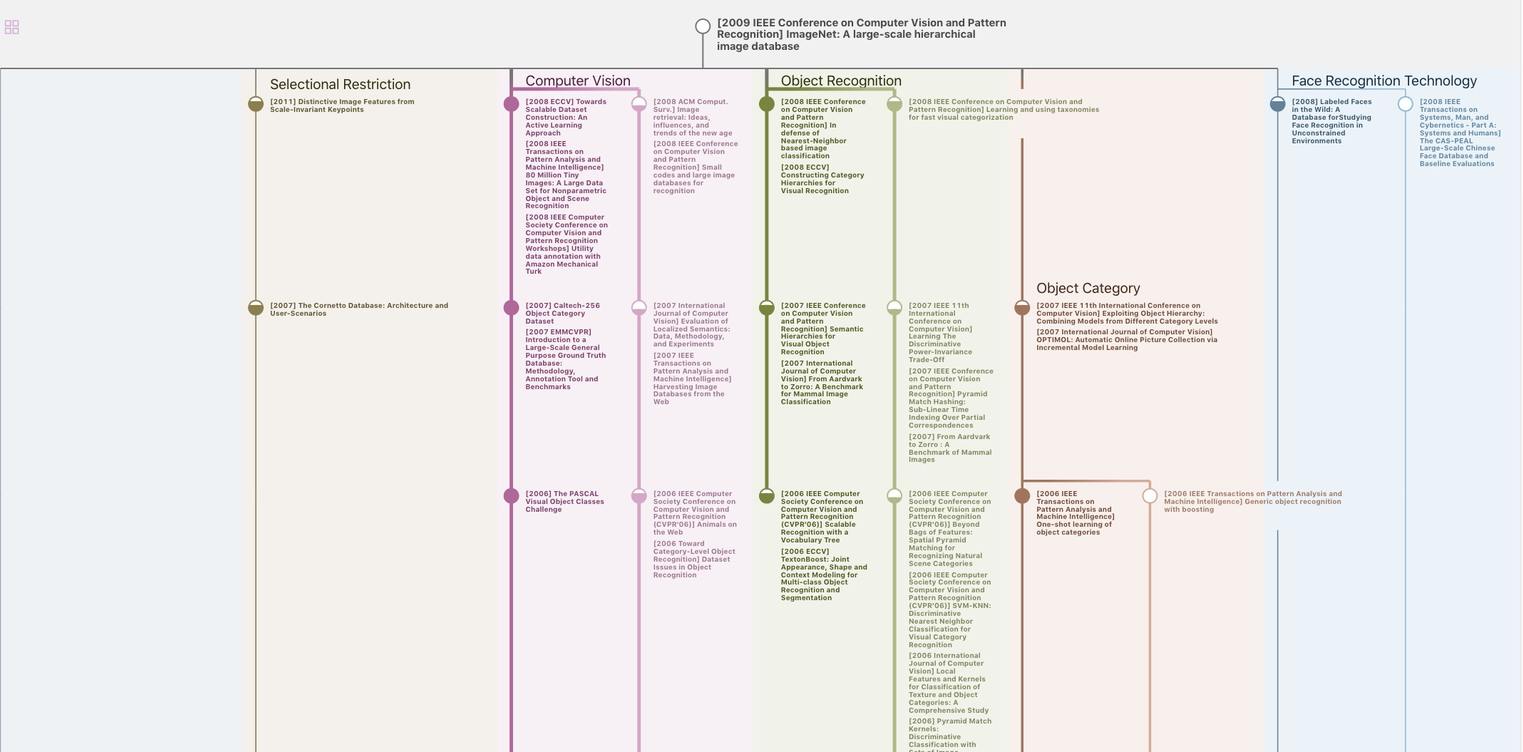
生成溯源树,研究论文发展脉络
Chat Paper
正在生成论文摘要