DNN based Adaptive User Pairing and Power Allocation to achieve α-Fairness in NOMA Systems with Imperfections in SIC.
AIMLSystems(2022)
摘要
Non-orthogonal multiple access (NOMA) technology aided with successive interference cancellation (SIC) is expected to achieve multi-fold improvements in the network capacity. However, the SIC in practice is prone to imperfections and this degrades the achievable gains with NOMA. Additionally, inappropriate user pairing and power allocation in NOMA can adversely affect the fairness between paired users. Hence, the impact of imperfections in SIC and fairness should be considered for user pairing and power allocation in NOMA. Motivated by this, we formulate the user pairing and power allocation to achieve α-fairness among the paired users as an optimization problem. To obtain a feasible solution in practice, we then propose a two-step machine learning-based approach to solve the problem. We use a random forest classifier (RFC) to establish a pairing criterion and a deep neural network (DNN) to allocate the power factors to the NOMA pair. The performance of the proposed supervised learning (SL) models is extensively evaluated and compared with other pre-existing algorithms. We analyze the performance of DNN for varying number of neurons in the hidden layer by considering different activation functions. We show that with 4 neurons in the hidden layer and sigmoid activation function, the trained DNN network outperforms the existing SL algorithms. We then use the trained network and perform Monte-Carlo simulations to quantify the achievable gains. We show that the proposed approach achieves an excellent solution that maximizes fairness and also ensures minimum required data rates for each user. Through extensive numerical evaluations, we show that our proposed two-step machine learning approach outperforms various state-of-the-art algorithms.
更多查看译文
AI 理解论文
溯源树
样例
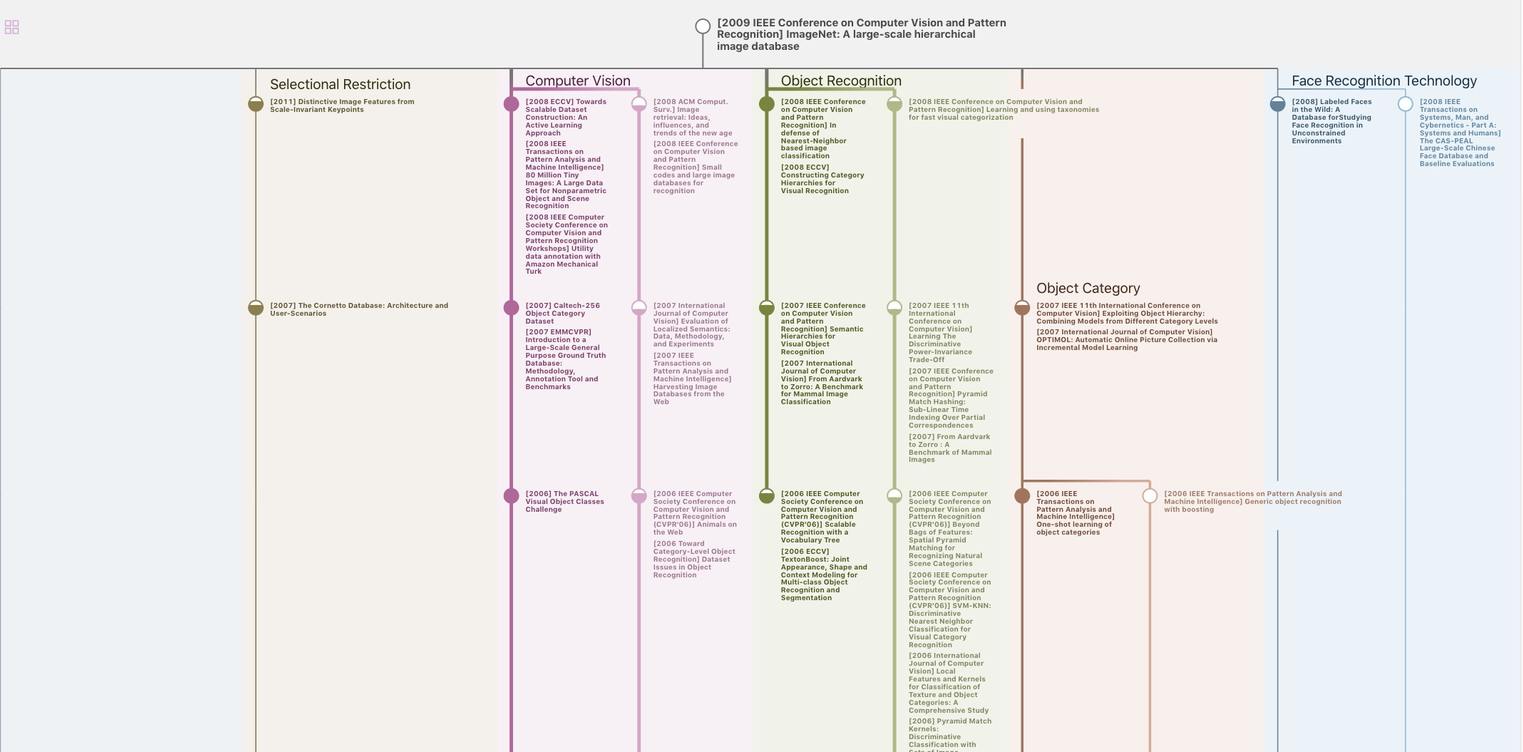
生成溯源树,研究论文发展脉络
Chat Paper
正在生成论文摘要