A Guessing Entropy-Based Framework for Deep Learning-Assisted Side-Channel Analysis
IEEE Trans. Inf. Forensics Secur.(2023)
摘要
Recently deep-learning (DL) techniques have been widely adopted in side-channel power analysis. A DL-assisted SCA generally consists of two phases: a deep neural network (DNN) training phase and a follow-on attack phase using the trained DNN. However, currently the two phases are not well aligned, as there is no conclusion on what metric used in the training can result in the most effective attack in the second phase. When traditional loss functions such as negative log-likelihood (NLL) are used in training a DNN, the trained model does not yield optimal follow-on attack. Recently some information theoretical SCA leakage metrics are proposed, either as the validation metric to stop the DNN training with traditional loss functions, or as both the validation metric and the training loss function. None of those proposed metrics, however, directly measures the SCA effectiveness. We propose to conduct DNN training directly with a common SCA effectiveness metric, Guessing Entropy (GE). We overcome the prior practical difficulty of using GE in DNN training by utilizing the GEEA estimation algorithm introduced in CHES 2020. We show that using GEEA as either the validation metric or the loss function produces DNN models that lead to much more effective follow-on attacks. Our work consolidates the DL-assisted SCA framework with a consistent metric, which shows great potential to be adopted as the universal SCA-oriented DNN training framework.
更多查看译文
关键词
Training,Measurement,Germanium,Deep learning,Loss measurement,Predictive models,Entropy,Side-channel analysis,deep learning,guessing entropy,evaluation metric
AI 理解论文
溯源树
样例
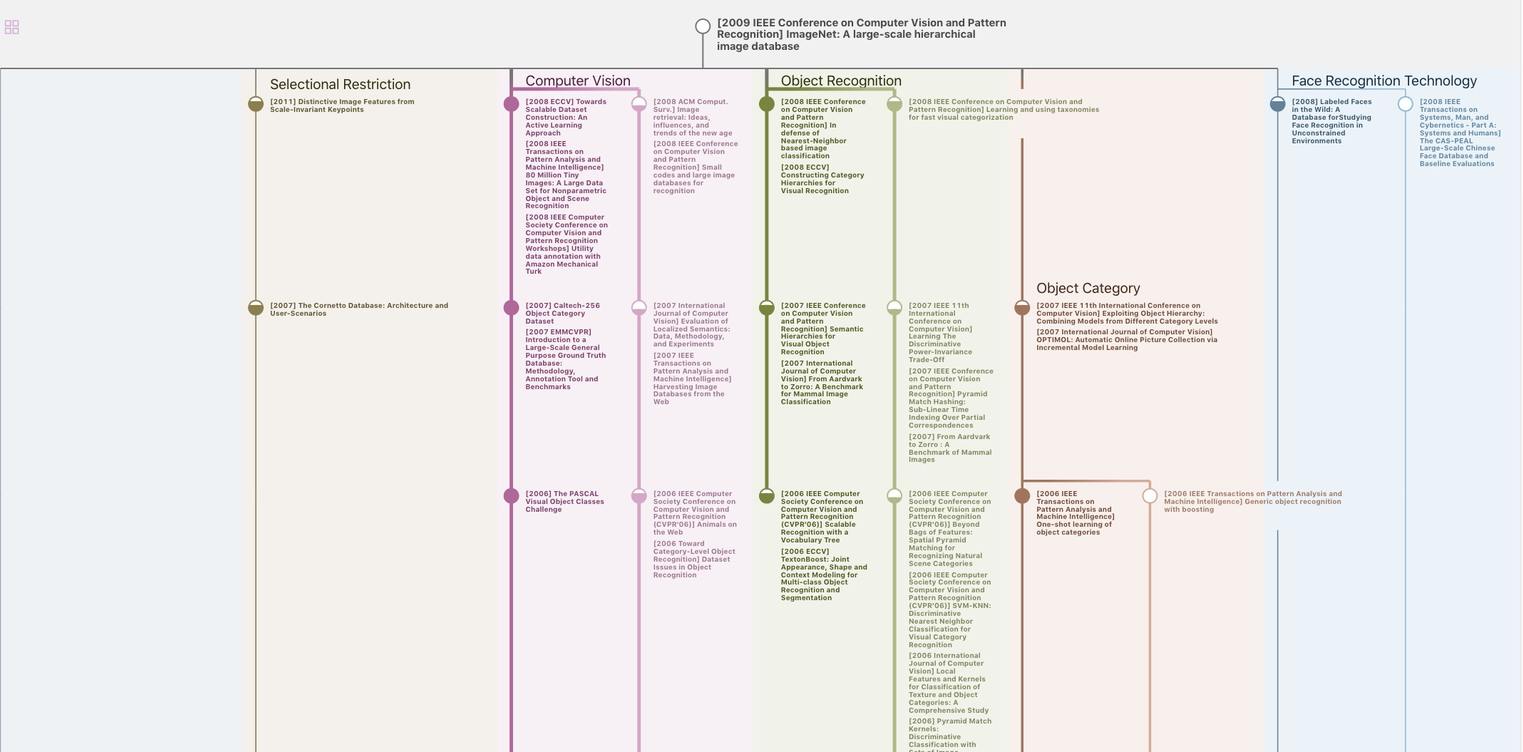
生成溯源树,研究论文发展脉络
Chat Paper
正在生成论文摘要