Few-shot semantic segmentation for industrial defect recognition.
Comput. Ind.(2023)
摘要
In modern manufacturing, vision-based defect recognition is an important technology to guarantee product quality. Deep learning-based vision recognition methods have made great progress in accuracy and generality than traditional vision methods. Training vision-based deep learning models requires a large amount of labeled data. However, data annotation is a laborious task and there is not enough defect data for annotation in many real productions, which becomes a bottleneck for deep learning in industrial applications. In this paper, we constructed a comparison dataset Industrial-5i, which is based on public datasets. This dataset can be used for defect detection methods by comparing images of normal and abnormal products. In addition, we propose a generic defect detection algorithm that not only learns how to compare positive and negative samples to segment defects but also generalizes well to new products. Compared with available few-shot segmentation methods, our method achieves the best defect detection results on the Industrial-5i dataset. Under 1-shot tasks, our method outperforms the baseline by 8.92% in mIoU and 7.68% in FB-IoU. Under 5-shot tasks, our method outperforms the baseline by 9.84% in mIoU and 8.46% in FB-IoU. Our code is available at https://github.com/Alex-ShiLei/IndustrialNet.
更多查看译文
关键词
industrial defect recognition,semantic segmentation,few-shot
AI 理解论文
溯源树
样例
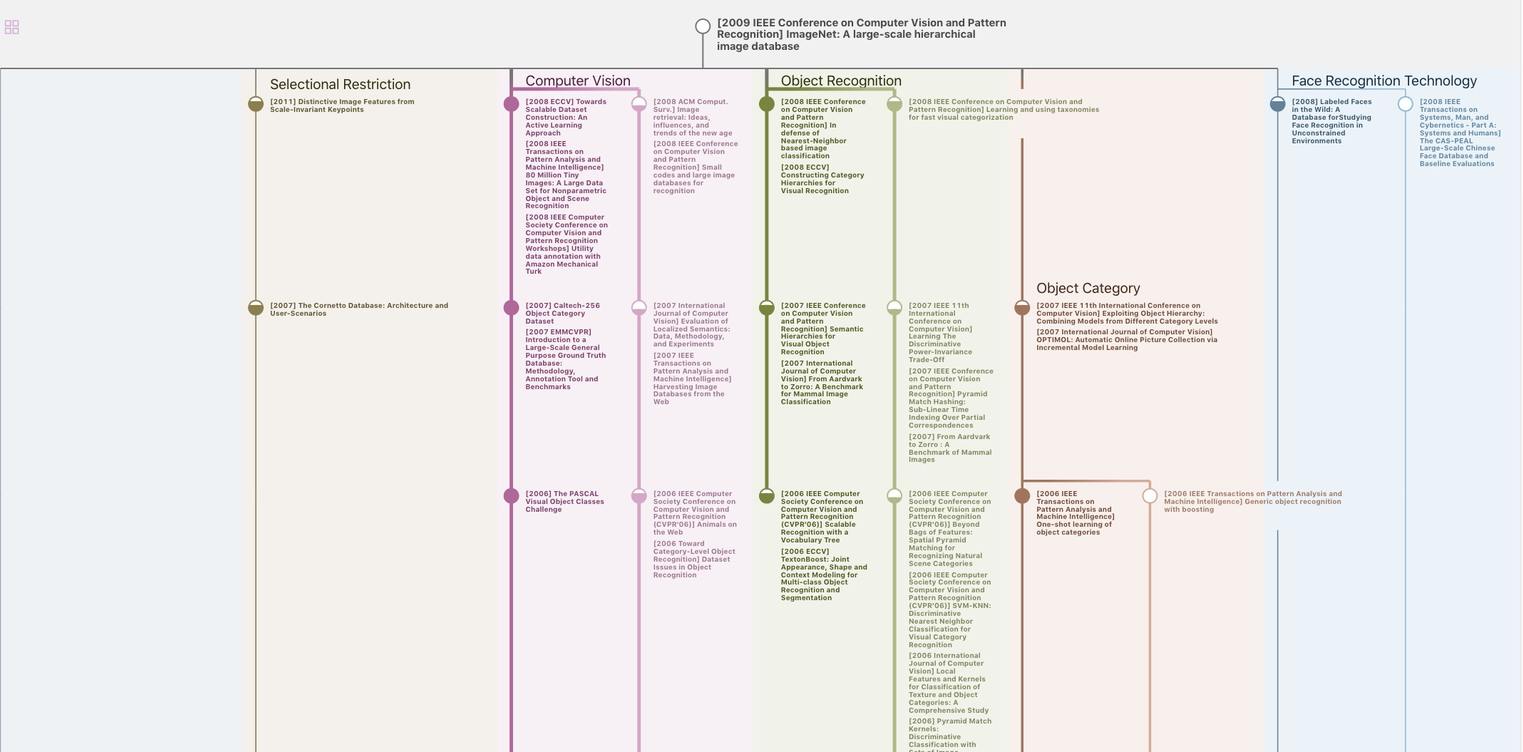
生成溯源树,研究论文发展脉络
Chat Paper
正在生成论文摘要