Enhanced Autoencoders With Attention-Embedded Degradation Learning for Unsupervised Hyperspectral Image Super-Resolution
IEEE Trans. Geosci. Remote. Sens.(2023)
摘要
Recently, unmixing-based networks have shown significant potential in unsupervised multispectral-aided hyperspectral image super-resolution (MS-aided HS-SR) task. Nevertheless, the representation ability of unsupervised networks and the design of loss functions still have not been fully explored, leaving large room for further improvement. To this end, we propose an enhanced unmixing-inspired unsupervised network with attention-embedded degradation learning (EU2ADL) to realize MS-aided HS-SR. First, two coupled autoencoders serve as the backbone of EU2ADL network to simultaneously decompose input modalities into abundances and corresponding endmembers, whose encoder part is composed of a spatial–spectral two-stream subnetwork for modality-salient representation learning and a parameter-shared one-stream subnetwork for modality-interacted representation enhancement. More importantly, a hybrid model-constrained loss containing a perceptual abundance term and a degradation-guided term is introduced to further eliminate the latent distortions. Since the hybrid loss is built on the degradation model, we additionally present an attention-embedded degradation learning network to adaptively estimate the unknown degradation parameters. Extensive experimental results on four datasets demonstrate the effectiveness of our proposed methods when compared with state of the arts.
更多查看译文
关键词
degradation learning,attention-embedded,super-resolution
AI 理解论文
溯源树
样例
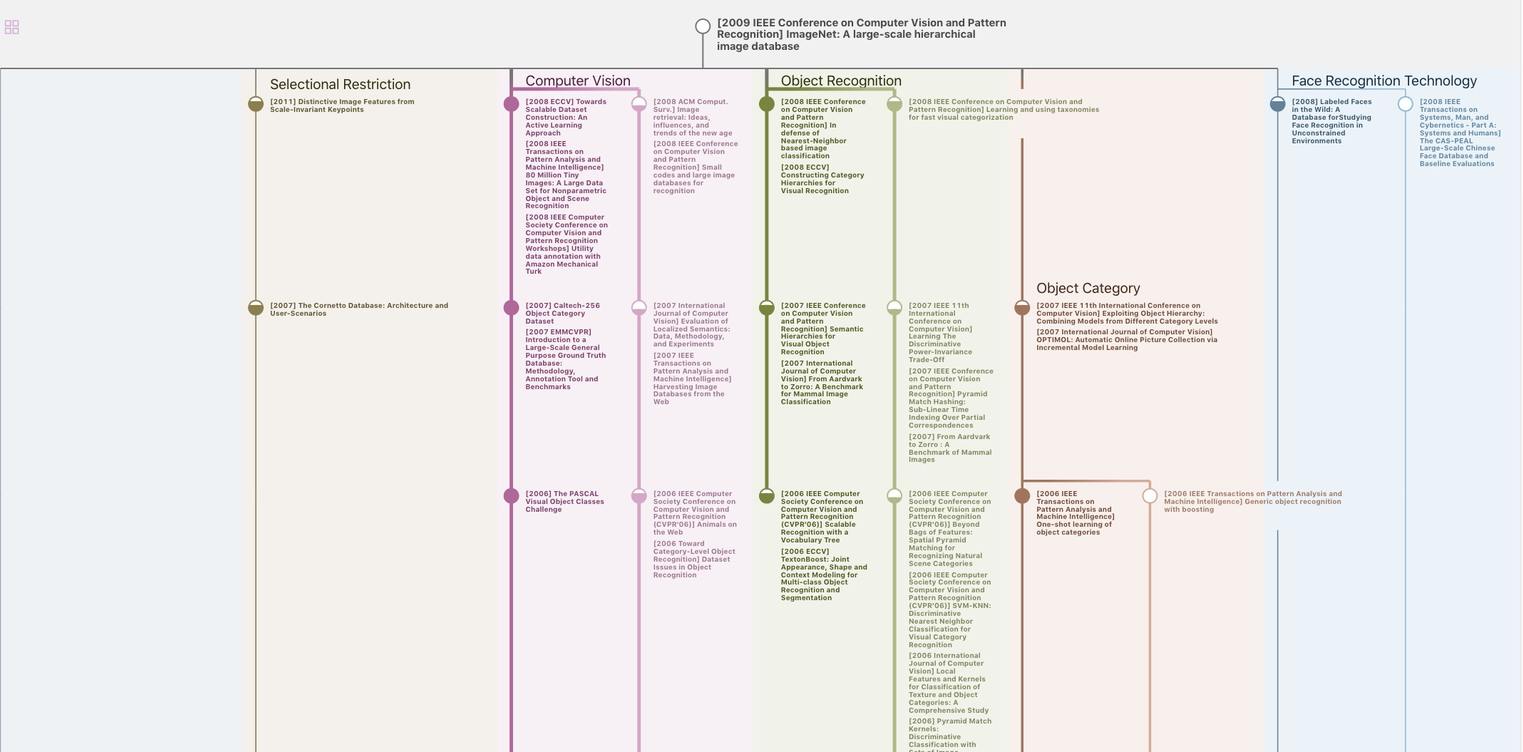
生成溯源树,研究论文发展脉络
Chat Paper
正在生成论文摘要