BTRPP: A Rapid PGA Prediction Model Based on Machine Learning.
IEEE Trans. Geosci. Remote. Sens.(2023)
摘要
Peak ground acceleration (PGA) is a critical parameter in the postearthquake intensity snapshot. In this article, we propose a Bagged Tree for Rapid PGA Prediction (BTRPP) based on velocity waveforms recorded at stations around the epicenter, as well as a feature pruning algorithm based on BTRPP to predict PGA and analyze the relationship between PGA and manually predefined features. The research not only aims to discover the best model for forecasting PGA but also examines the impact of features using the interpretability of some models. As inputs to the model, BTRPP uses and mixes features in the time and frequency domains. Bayesian optimization (BO) is used to find the optimum hyperparameters of BTRPP. The results demonstrate that, using the data within 5 s after the first arrival of the P-wave, the BTRPP with BO can reduce the RMSE to 0.2076 and enhance the R-square to 0.91. It is, finally, found that the PGA is most closely related to the average positive amplitude, average negative amplitude, and spectral slope through the feature pruning algorithm in this article, which indicates that the results are plausible.
更多查看译文
关键词
Machine learning algorithms, Fuses, Frequency-domain analysis, Machine learning, Predictive models, Prediction algorithms, Time-domain analysis, Bagged tree, feature pruning, hyperparameter optimization, machine learning, multifeature fusion, peak ground acceleration (PGA)
AI 理解论文
溯源树
样例
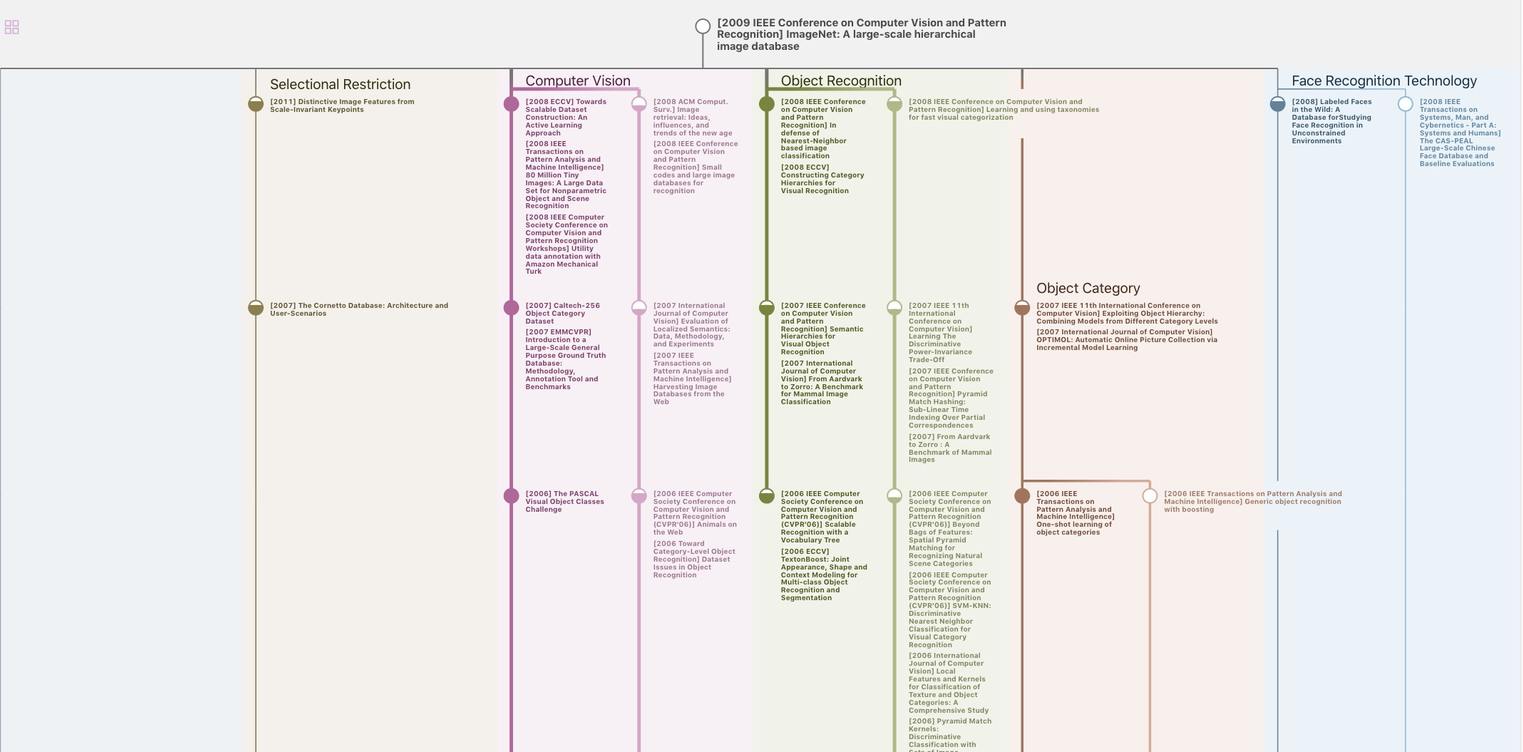
生成溯源树,研究论文发展脉络
Chat Paper
正在生成论文摘要