Percolate: An Exponential Family JIVE Model to Design DNA-Based Predictors of Drug Response.
RECOMB(2023)
摘要
Motivation: Anti-cancer drugs may elicit resistance or sensitivity through mechanisms which involve several genomic layers. Nevertheless, we have demonstrated that gene expression contains most of the predictive capacity compared to the remaining omic data types. Unfortunately, this comes at a price: gene expression biomarkers are often hard to interpret and show poor robustness. Results: To capture the best of both worlds, i.e. the accuracy of gene expression and the robustness of other genomic levels, such as mutations, copy-number or methylation, we developed Percolate, a computational approach which extracts the joint signal between gene expression and the other omic data types. We developed an out-of-sample extension of Percolate which allows predictions on unseen samples without the necessity to recompute the joint signal on all data. We employed Percolate to extract the joint signal between gene expression and either mutations, copy-number or methylation, and used the out-of sample extension to perform response prediction on unseen samples. We showed that the joint signal recapitulates, and sometimes exceeds, the predictive performance achieved with each data type individually. Importantly, molecular signatures created by Percolate do not require gene expression to be evaluated, rendering them suitable to clinical applications where only one data type is available. Availability: Percolate is available as a Python 3.7 package and the scripts to reproduce the results are available here .
更多查看译文
关键词
exponential family jive model,drug response,predictors,dna-based
AI 理解论文
溯源树
样例
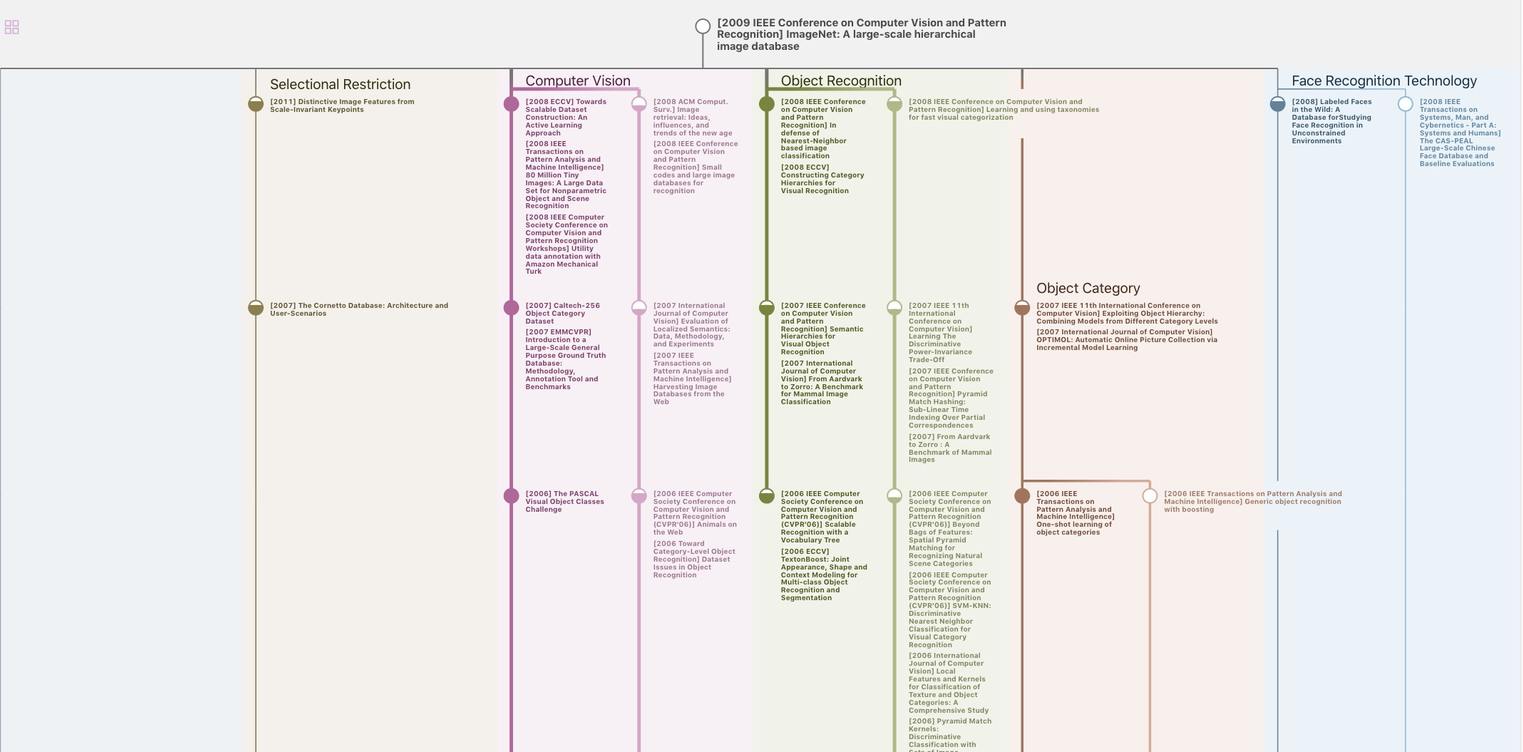
生成溯源树,研究论文发展脉络
Chat Paper
正在生成论文摘要