Throughput optimization for backscatter-and-NOMA-enabled wireless powered cognitive radio network
Telecommun. Syst.(2023)
摘要
In a typical wireless powered cognitive radio network, secondary transmitters (STs) perform energy harvesting when the channel is busy and perform active transmission using the harvested energy when the channel is idle. In order to further enhance the throughput of the network, we enable time-division-multiple-access-based backscatter communication (BackCom) when the channel is busy and non-orthogonal-multiple-access (NOMA)-based active transmission when the channel is idle. We aim to maximize the longterm average sum-throughput of all the STs by allocating each ST’s BackCom time, energy harvesting time and transmit power for the NOMA-based active transmission. With consideration of limited battery capacity and time-varying channel, we formulate the problem as a Markov decision process. Both Q-learning and deep Q-learning (DQL) algorithms are proposed to solve the problem to obtain fully online policies. Simulation results show that the proposed DQL algorithm not only efficiently deals with the dynamics of the environment but also improves the average throughput up to 27.5% compared with Q-learning and up to 4 times compared with random policy.
更多查看译文
关键词
Backscatter communication, Wireless powered cognitive radio network, Nonorthogonal multiple access, Throughput, Deep Q-learning
AI 理解论文
溯源树
样例
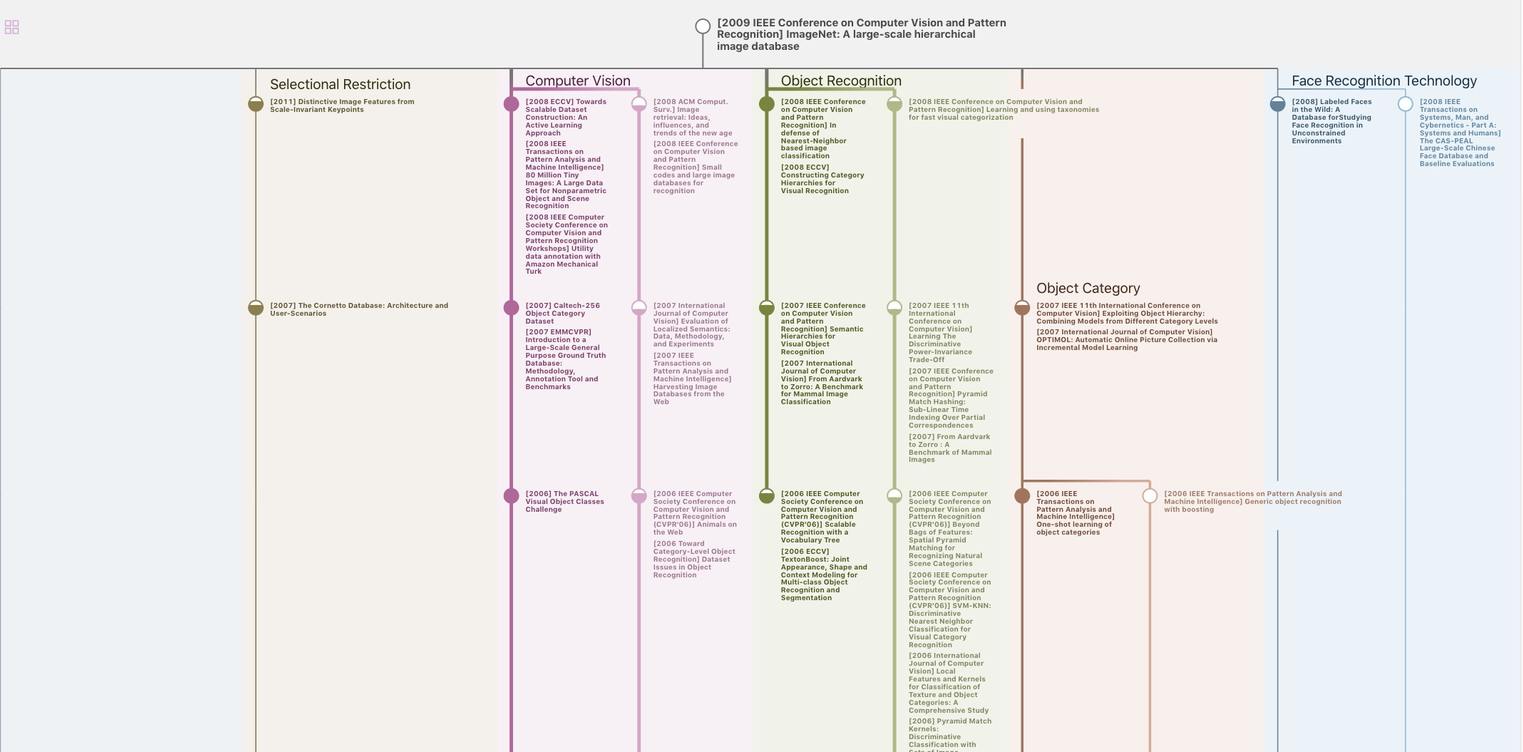
生成溯源树,研究论文发展脉络
Chat Paper
正在生成论文摘要