Reinforcement Learning for Enhancing Human Security Resource Allocation in Protecting Assets with Heterogeneous Losses
PROCEEDINGS OF THE 2023 ACM WORKSHOP ON SECURE AND TRUSTWORTHY CYBER-PHYSICAL SYSTEMS, SAT-CPS 2023(2023)
摘要
Given the rising cyber threats on today's cyber-physical systems (CPS), enhancing security resource allocation is highly desirable. We consider a security resource allocation setting that involves a human defender who is required to invest her (limited) security in protecting a given set of assets against attacks. Each asset has a certain loss valuation to the defender, along with a probability of being successfully compromised. The success probability of each asset is a function of the resource allocation in that asset by the defender. In this setting, we first model the impacts of behavioral probability weighting that is identified by behavioral economics to be a common feature of human decision-making on the security resource allocation strategies. We then propose a reinforcement learning (RL) technique for enhancing security resource allocation in multi-round setups. We illustrate the effect of probability weighting on the security investments and the benefits of our RL algorithm via numerical simulations. In particular, we show that RL can guide human behavioral defender in enhancing her security investments to make the system more secure.
更多查看译文
关键词
Reinforcement Learning,Risk Assessment,Security Resource Allocation,Cyber-Physical Systems,Prospect Theory
AI 理解论文
溯源树
样例
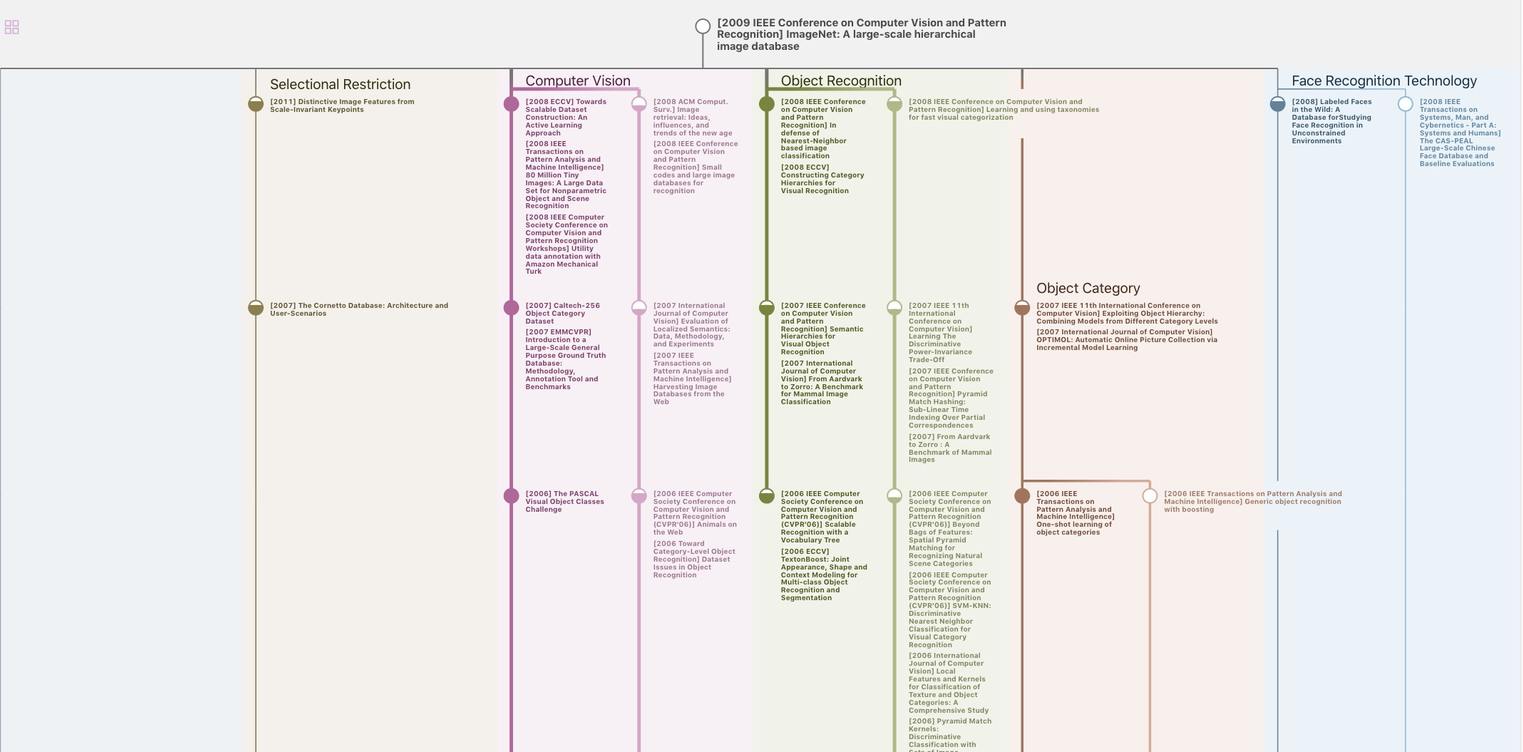
生成溯源树,研究论文发展脉络
Chat Paper
正在生成论文摘要