On Skin Lesion Recognition Using Deep Learning: 50 Ways to Choose Your Model.
PSIVT(2022)
摘要
Skin cancer is a highly relevant health problem around the world. The World Health Organization (WHO) reports that one-third of the diagnosed cancers are skin cancer. It is well known that early detection of skin cancer significantly increases the prognosis of patients. In many cases, however, the absence of clinical devices and qualified experts makes this task very difficult. To overcome this problem, in the last years advanced deep learning techniques have been proposed to recognize skin cancer automatically showing promising results. In this work, we evaluate 50 deep learning approaches on the well-known HAM10000 dataset of dermatoscopic images from seven different skin lesion categories. The approaches have been evaluated using the same experimental protocol. In our experiments, the performance of each approach in terms of accuracy and computational time are measured. In addition, the number of trainable parameters and the number of features of the last layer of the deep learning architecture are given. Thus, comparison between the approaches can be easily established. The results showed that the best performance has been achieved by a deep learning approach based on visual transformers with 84.29% of accuracy on the testing subset. We know that these results may vary significantly on other datasets. For this reason, rather than establish which method is the best, the main contribution of this work is to make the 50 deep learning approaches available in a simple way for future research in the area. We believe that this methodology, i.e. , training, testing and evaluating many deep learning methods, can be very helpful to establish which is the best architecture and what is the highest performance that can be achieved on new datasets.
更多查看译文
关键词
skin lesion recognition,deep learning
AI 理解论文
溯源树
样例
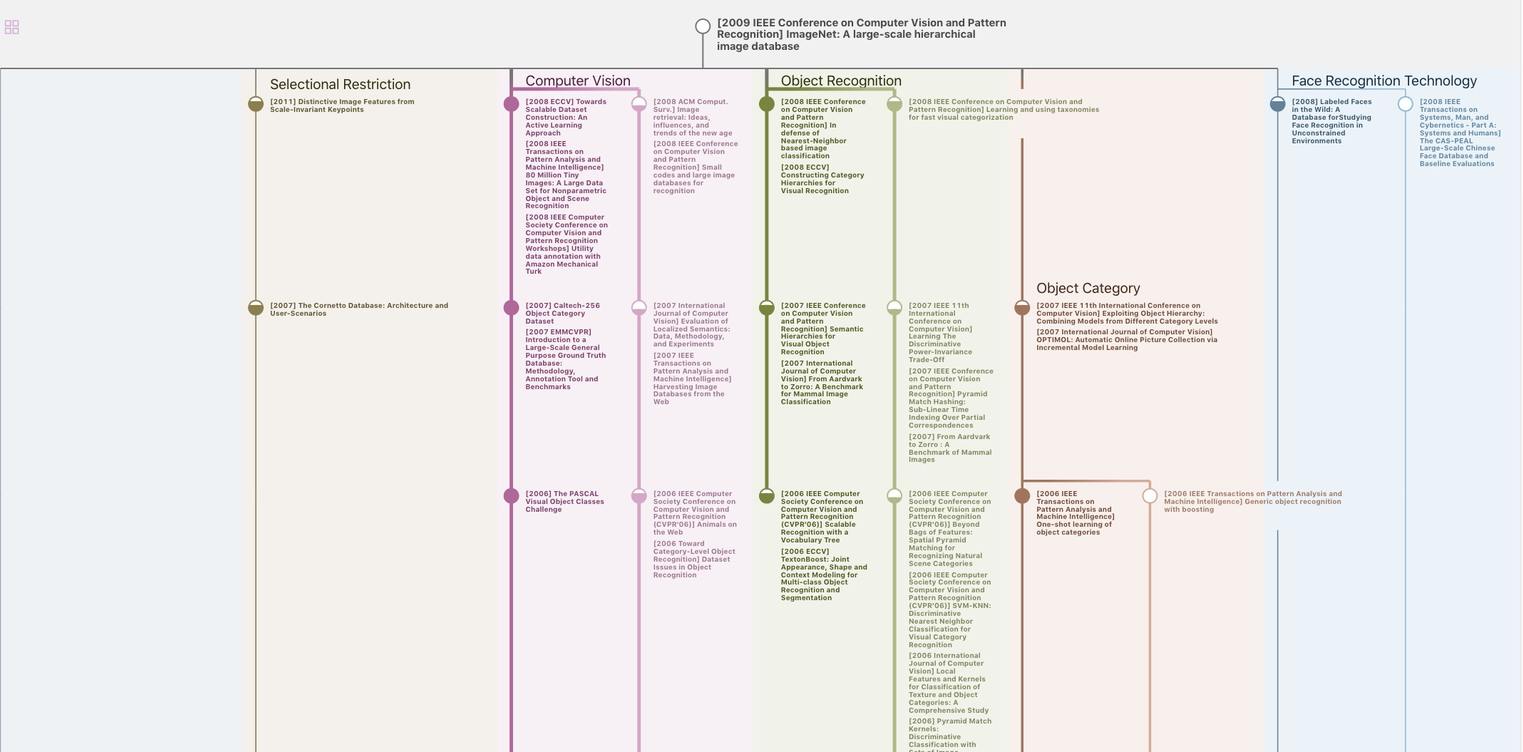
生成溯源树,研究论文发展脉络
Chat Paper
正在生成论文摘要