Structural Bias in Knowledge Graphs for the Entity Alignment Task.
ESWC(2023)
摘要
Knowledge Graphs (KGs) have recently gained attention for representing knowledge about a particular domain and play a central role in a multitude of AI tasks like recommendations and query answering. Recent works have revealed that KG embedding methods used to implement these tasks often exhibit direct forms of bias (e.g., related to gender, nationality, etc.) leading to discrimination. In this work, we are interested in the impact of indirect forms of bias related to the structural diversity of KGs in entity alignment (EA) tasks. In this respect, we propose an exploration-based sampling algorithm, SUSIE, that generates challenging benchmark data for EA methods, with respect to structural diversity. SUSIE requires setting the value of a single hyperparameter, which affects the connectivity of the generated KGs. The generated samples exhibit similar characteristics to some of the most challenging real-world KGs for EA tasks. Using our sampling, we demonstrate that state-of-the-art EA methods, like RREA, RDGCN, MultiKE and PARIS, exhibit different robustness to structurally diverse input KGs.
更多查看译文
关键词
entity alignment task,knowledge graphs,bias
AI 理解论文
溯源树
样例
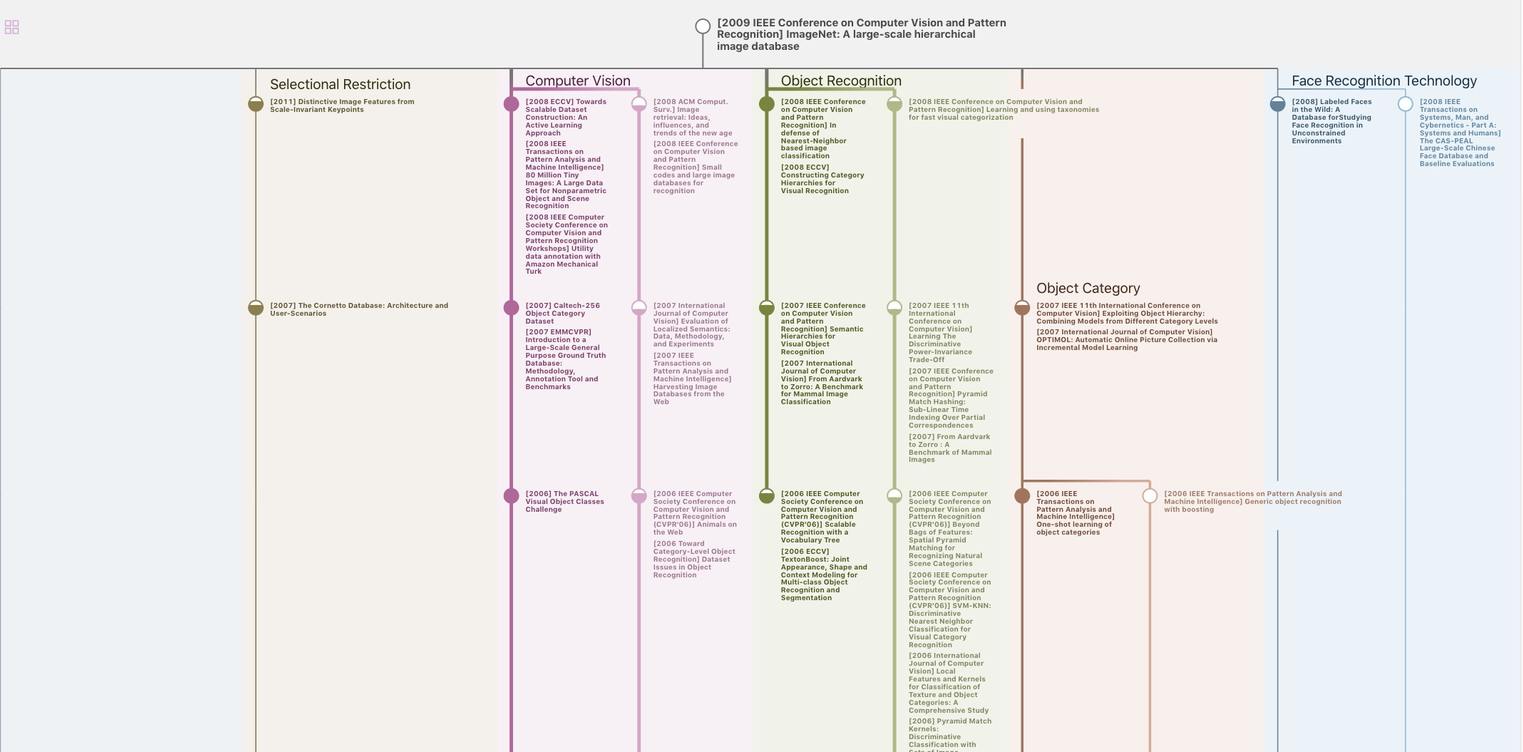
生成溯源树,研究论文发展脉络
Chat Paper
正在生成论文摘要