Federated Learning Under Statistical Heterogeneity on Riemannian Manifolds.
PAKDD (1)(2023)
摘要
Federated learning (FL) is a collaborative machine learning paradigm in which clients with limited data collaborate to train a single “best” global model based on consensus. One major challenge facing FL is the statistical heterogeneity among the data for each of the local clients. Clients trained with non-IID or imbalanced data whose models are aggregated using averaging schemes such as FedAvg may result in a biased global model with a slow training convergence. To address this challenge, we propose a novel and robust aggregation scheme, FedMan, which assigns each client a weighting factor based on its statistical consistency with other clients. Such statistical consistency is measured on a Riemannian manifold spanned by the covariance of the local client output logits. We demonstrate the superior performance of FedMAN over several FL baselines (FedAvg, FedProx, and Fedcurv) as applied to various benchmark datasets (MNIST, Fashion-MNIST, and CIFAR-10) under a wide variety of degrees of statistical heterogeneity.
更多查看译文
关键词
federated learning,riemannian manifolds,statistical heterogeneity
AI 理解论文
溯源树
样例
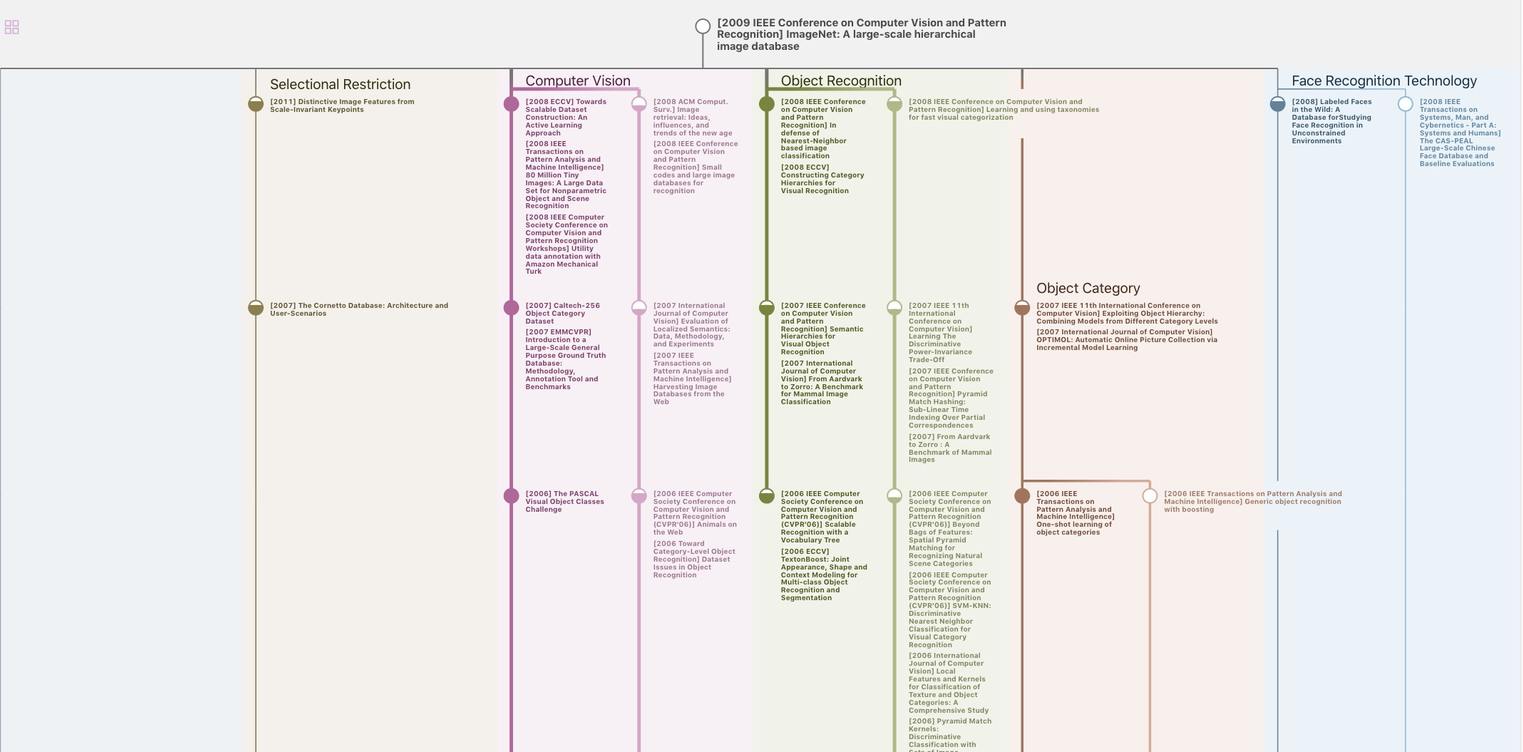
生成溯源树,研究论文发展脉络
Chat Paper
正在生成论文摘要