Anti-Money Laundering in Cryptocurrency via Multi-Relational Graph Neural Network.
PAKDD (2)(2023)
摘要
The cryptocurrency market has been growing exponentially. However, due to their anonymity, cryptocurrencies are frequently abused for laundering money obtained from illegal activities. Although recent approaches based on Graph Neural Networks (GNNs) have shown remarkable achievements in fraud detection, investigating cryptocurrency transaction networks is subject to the following challenges: 1) There is a lack of useful node features as cryptocurrencies block access to user information in principle. 2) The classification tasks are extremely disproportionate since fraudsters are very few compared to benign addresses. 3) Lastly, the computational cost must be considered for large-scale analysis in real-world scenarios. This study presents a novel approach for examining financial transactions to detect anomalies in cryptocurrency networks. We design a multi-relational GNN that incorporates the orientation and characteristics of edges, such as the amount or frequency of transactions. In addition, an adaptive neighbor sampler is designed to improve spotting performance while effectively containing computational costs. Experiments on real-world datasets demonstrate that our proposed method outperforms state-of-the-art GNN-based fraud detectors.
更多查看译文
关键词
cryptocurrency,graph,anti-money,multi-relational
AI 理解论文
溯源树
样例
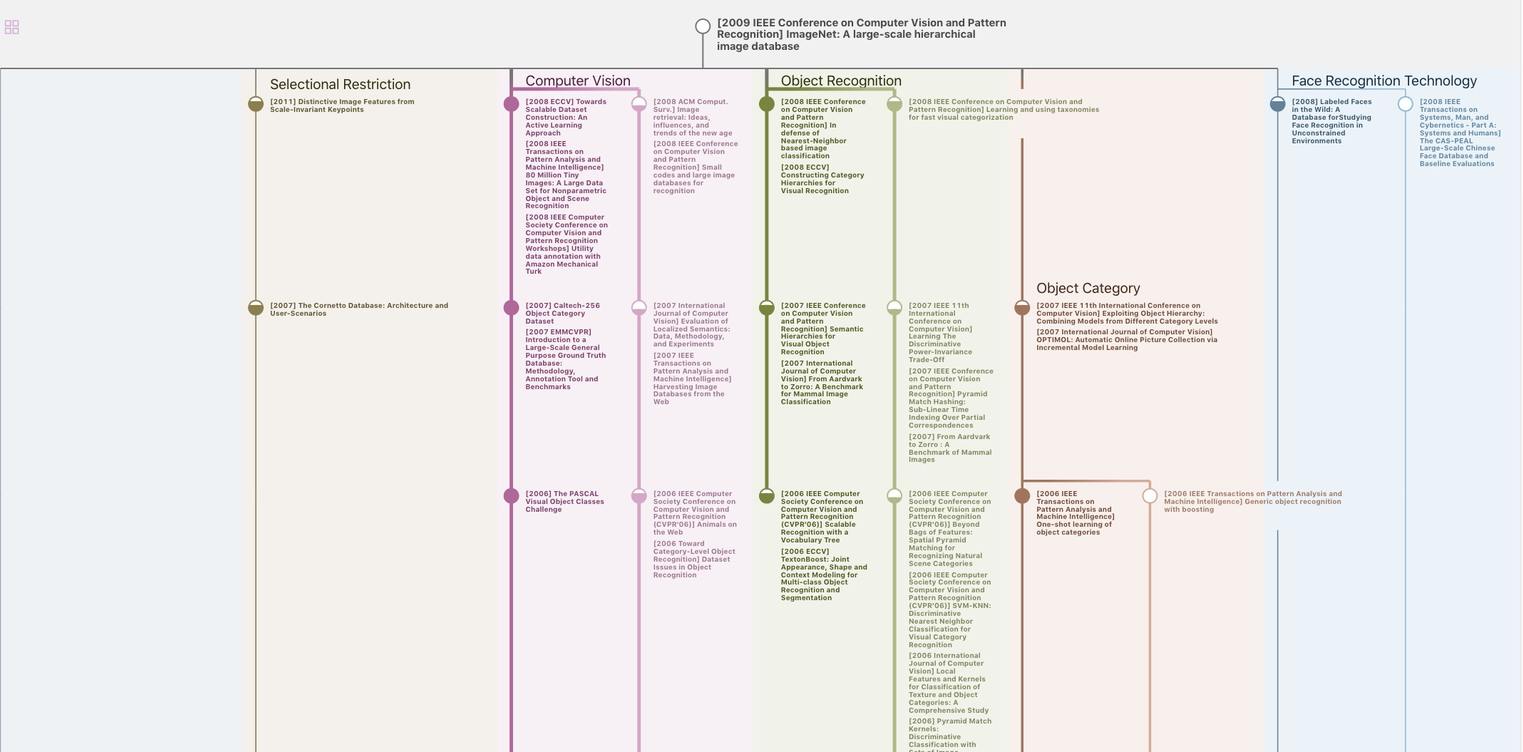
生成溯源树,研究论文发展脉络
Chat Paper
正在生成论文摘要