Topological Graph Convolutional Networks Solutions for Power Distribution Grid Planning.
PAKDD (1)(2023)
摘要
With ever rising energy demands along with continuing proliferation of clean energy sources, the expanding analytic needs of the modern power sector can no longer be met by prevailing physical-based models and require new automatic solutions for planning, monitoring, and controlling tasks. In turn, artificial intelligence (AI) offers many necessary tools to develop such novel solutions. In this paper we take the first step towards bringing the utility of Topological Graph Neural Networks to power distribution grid planning and resilience quantification. We develop new Graph Convolutional Networks coupled with a zigzag topological layer for classification of distribution grid expansion plans. We also introduce bootstrap over the extracted zigzag persistence representations of the distribution grids which allows us to learn the most characteristic, or hereditary topological signatures over multiple graphs from the same family and, as a result, to improve classification performance both in terms of accuracy and stability. Our numerical experiments show that the new Bootstrapped Zigzag Persistence Based Graph Convolutional Networks (BZP-GCN) yields substantial gains in computational efficiency compared to the traditional methodology to assess the quality of investment and planning of distribution grids. Furthermore, BZP-GCN outperforms by a significant margin 6 state-of-the-art models in terms of classification accuracy.
更多查看译文
关键词
power distribution grid planning,networks
AI 理解论文
溯源树
样例
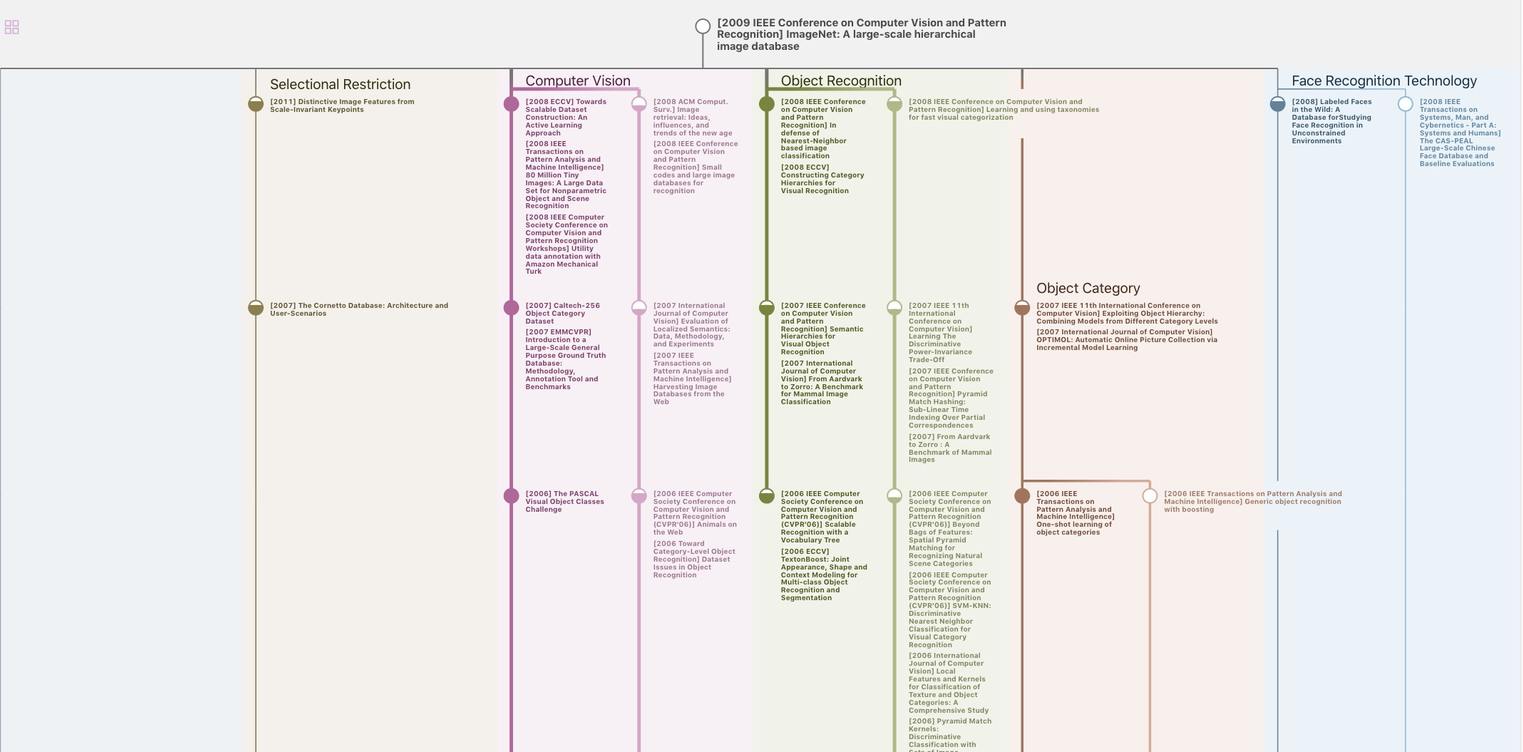
生成溯源树,研究论文发展脉络
Chat Paper
正在生成论文摘要