Fair Training of Multiple Federated Learning Models on Resource Constrained Network Devices
PROCEEDINGS OF THE 2023 THE 22ND INTERNATIONAL CONFERENCE ON INFORMATION PROCESSING IN SENSOR NETWORKS, IPSN 2023(2023)
摘要
Federated learning (FL) is an increasingly popular form of distributed learning across devices such as sensors and smartphones. To amortize the effort and cost of setting up FL training in real world systems, in practice multiple machine learning tasks may be trained during one FL execution. However, given that the tasks have varying complexities, naive methods of allocating resource-constrained devices to work on each task may lead to highly variable performance across the tasks. We instead propose an alpha-fair based allocation algorithm that dynamically allocates tasks to users during multi-model FL training, based on the prevailing loss levels.
更多查看译文
关键词
Federated Learning,Resource Allocation
AI 理解论文
溯源树
样例
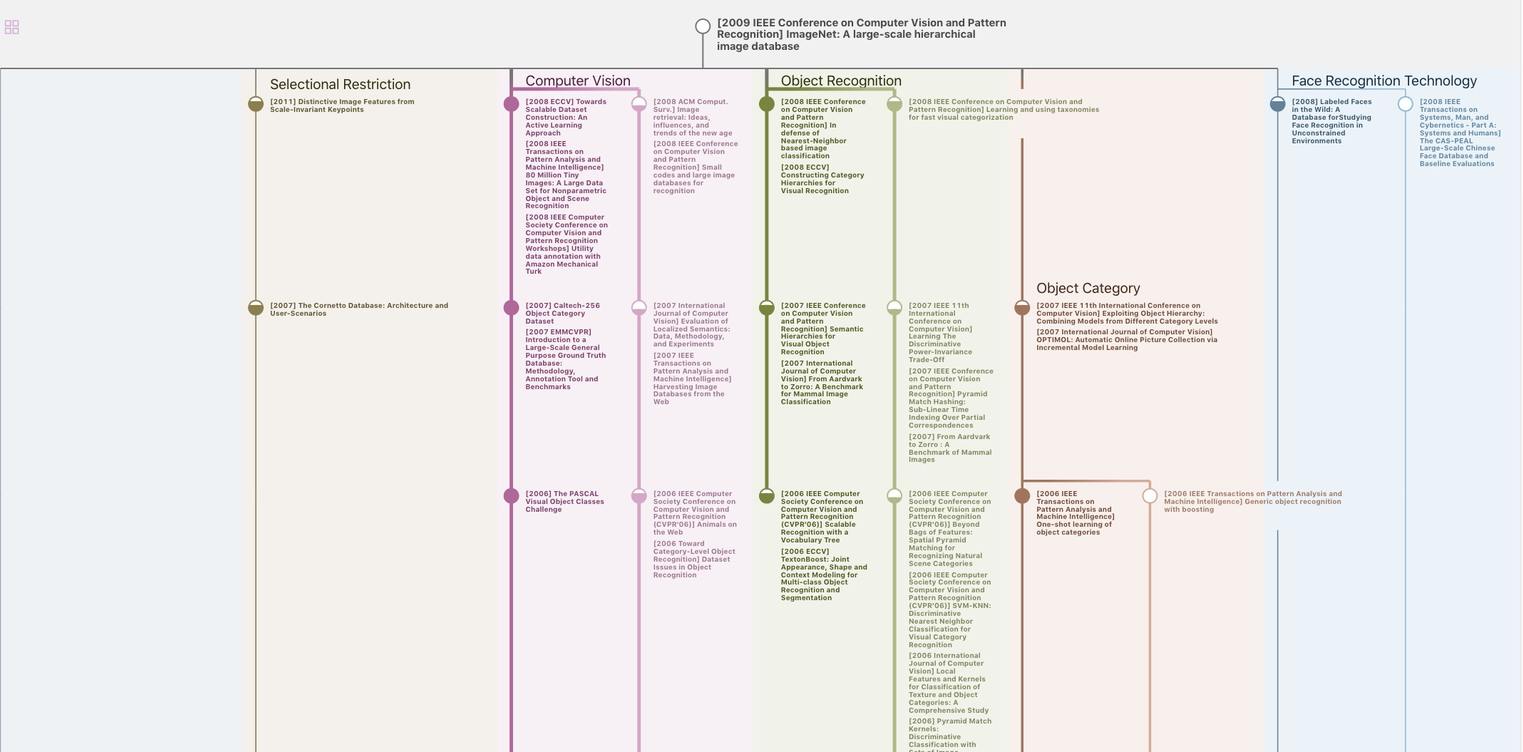
生成溯源树,研究论文发展脉络
Chat Paper
正在生成论文摘要