A Dynamic and Task-Independent Reward Shaping Approach for Discrete Partially Observable Markov Decision Processes.
PAKDD (2)(2023)
摘要
Agents often need a long time to explore state-action space in order to learn how to act expectedly in Partially Observable Markov Decision Processes (POMDPs). With the reward shaping method, real-time POMDP planning can be guided both in terms of reliability and speed. In this paper, we propose Low Dimensional Policy Graph (LDPG), a new reward shaping method for reducing the dimension of the value function to extract the best state-action pairs. The reward function is then shaped using these key pairs. For accelerating learning speed, we analyze the Transition Function graph to discover significant paths to the learning agent’s goal. Direct comparison on five standard testbeds indicates LDPG brings about the deterministic finding of optimal actions faster regardless of the task type. Our method is shown to reach the goals more quickly (by 41.48 % improvement) and performed 61.57 % better in receiving rewards in the
$$ 4 \times 5 \times 2 $$
domain.
更多查看译文
关键词
dynamic,decision,discrete partially,task-independent
AI 理解论文
溯源树
样例
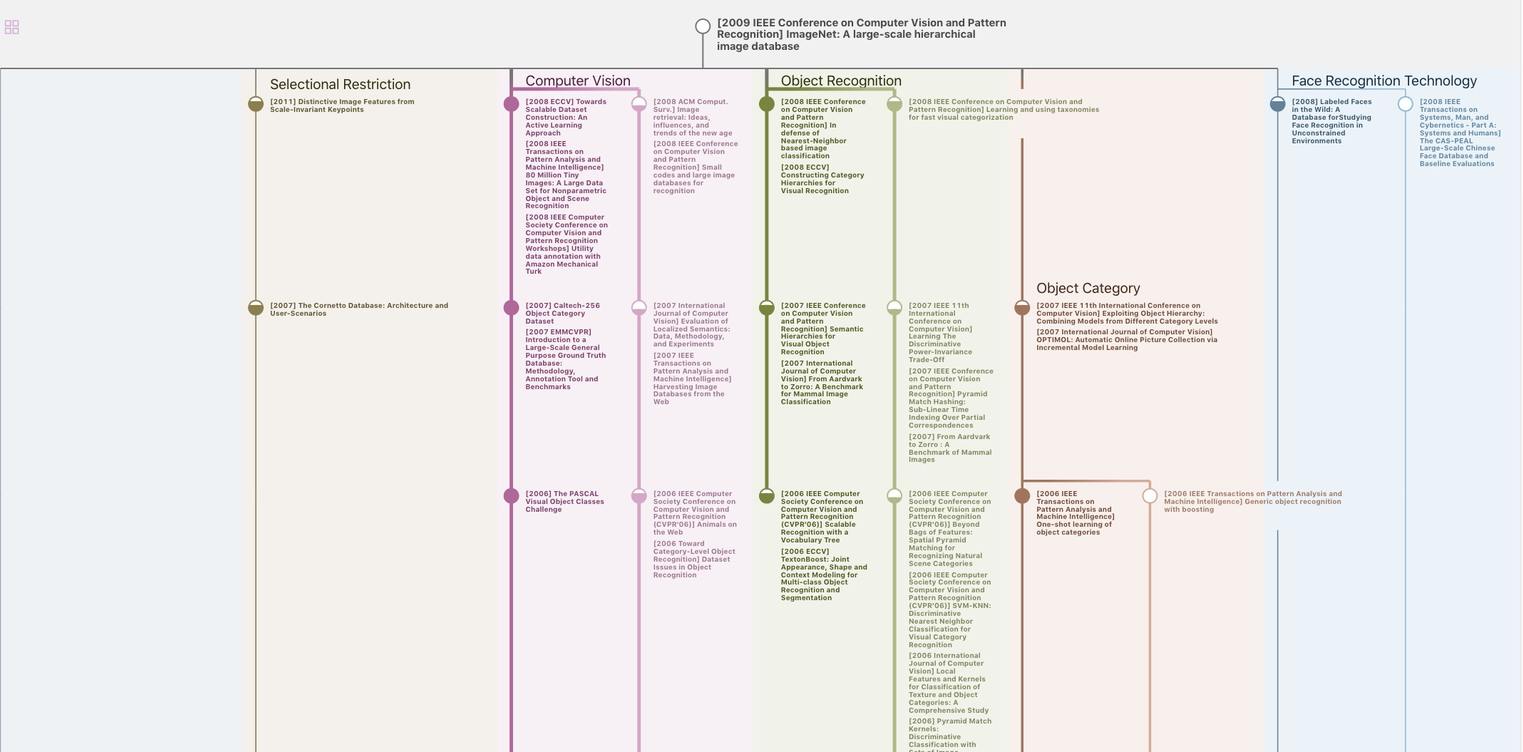
生成溯源树,研究论文发展脉络
Chat Paper
正在生成论文摘要