Toward Interpretable Machine Learning: Constructing Polynomial Models Based on Feature Interaction Trees.
PAKDD (2)(2023)
摘要
As AI has been applied in many decision-making processes, ranging from loan application approval to predictive policing, the interpretability of machine learning models is increasingly important. Interpretable models and post-hoc explainability are two approaches in eXplainable AI (XAI). We follow the argument that transparent models should be used instead of black-box ones in real-world applications, especially regarding high-stakes decisions. In this paper, we propose PolyFIT to address two major issues in XAI: (1) bridging the gap between black-box and interpretable models and (2) experimentally validating the trade-off relationship between model performance and explainability. PolyFIT is a novel polynomial model construction method assisted by the knowledge of feature interactions in black-box models. PolyFIT uses extracted feature interaction knowledge to build interaction trees, which are then transformed into polynomial models. We evaluate the predictive performance of PolyFIT with baselines using four publicly available data sets, Titanic survival, Adult income, Boston house price, and California house price. Our method outperforms linear models by 5% and 56% in classification and regression tasks on average, respectively. We also conducted usability studies to derive the trade-off relationship between model performance and explainability. The studies validate our hypotheses about the actual relationship between model performance and explainability.
更多查看译文
关键词
interpretable machine learning,polynomial models,machine learning,trees
AI 理解论文
溯源树
样例
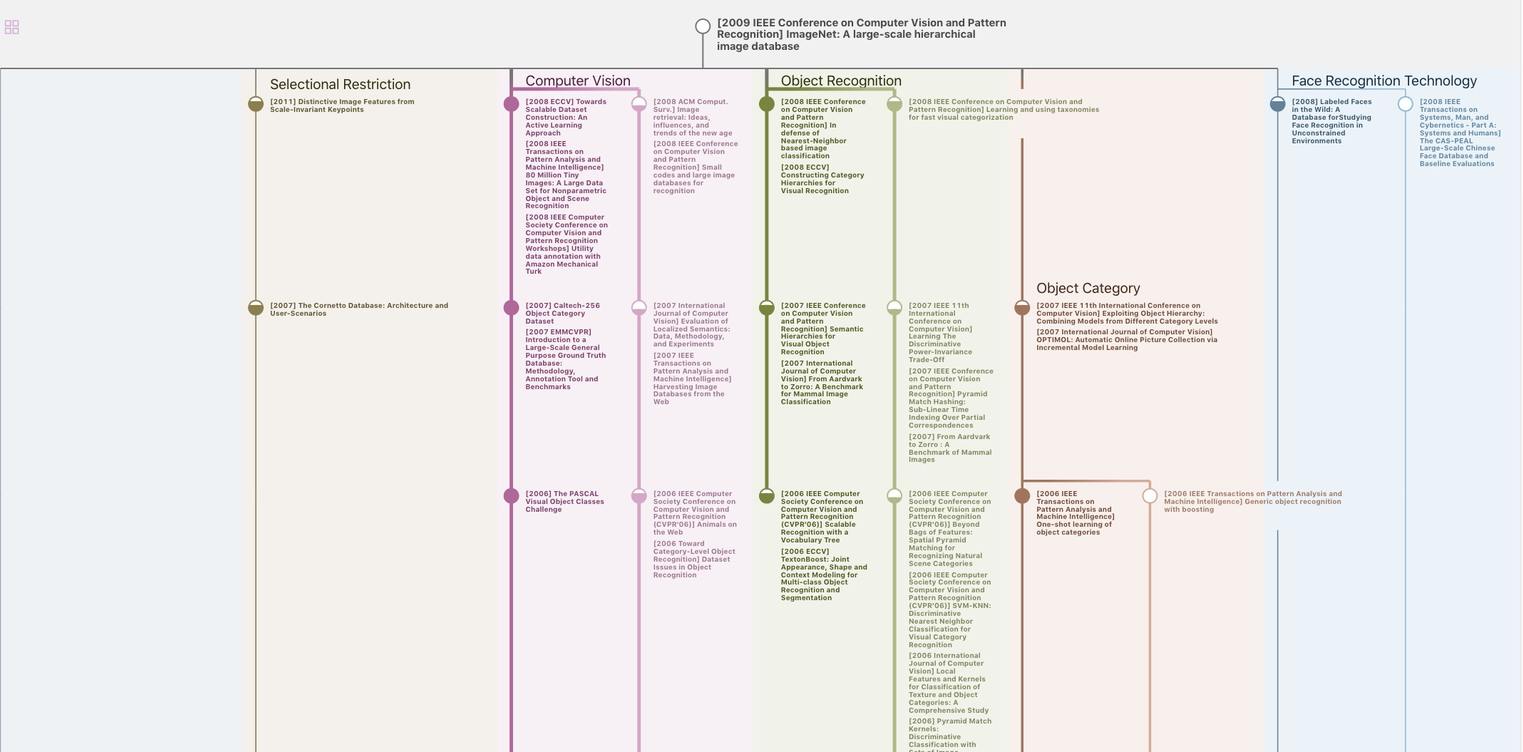
生成溯源树,研究论文发展脉络
Chat Paper
正在生成论文摘要