Fragment Linker Prediction Using the Deep Encoder-Decoder Network for PROTACs Drug Design.
J. Chem. Inf. Model.(2023)
摘要
A drug discovery and development pipeline is a prolonged and complex process that remains challenging for both computational methods and medicinal chemists and has not been able to be resolved using computational methods. Deep learning has been utilized in various fields and achieved tremendous success in designing novel molecules in the pharmaceutical industry. Herein, we use state-of-the-art techniques to propose a deep neural network, AIMLinker, to rapidly design and generate meaningful drug-like proteolysis targeting chimeras (PROTACs) analogs. The model extracts the structural information from the input fragments and generates linkers to incorporate them. We integrate filters in the model to exclude nondruggable structures guided via protein-protein complexes while retaining molecules with potent chemical properties. The novel PROTACs subsequently pass through molecular docking, taking root-mean-square deviation (RMSD), relative Gibbs free energy (), molecular dynamics (MD) simulation, and free energy perturbation (FEP) calculations as the measurement criteria for testing the robustness and feasibility of the model. The generated novel PROTACs molecules possess similar structural information with superior binding affinity to the binding pockets compared to the existing CRBN-dBET6-BRD4 ternary complexes. We demonstrate the effectiveness of the methodology of leveraging AIMLinker to design novel compounds for PROTACs molecules exhibiting better chemical properties compared to the dBET6 crystal pose.
更多查看译文
关键词
drug,fragment,prediction,encoder-decoder
AI 理解论文
溯源树
样例
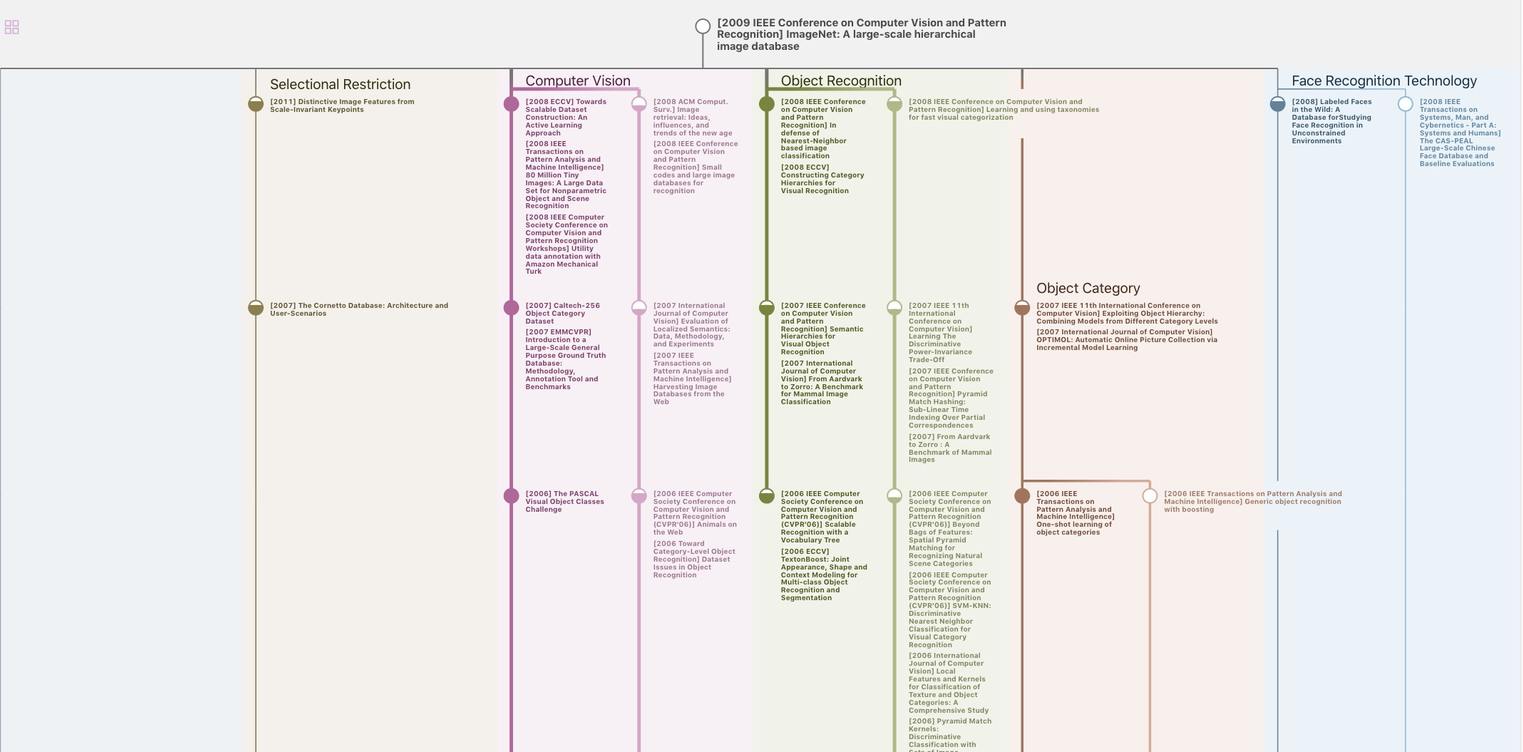
生成溯源树,研究论文发展脉络
Chat Paper
正在生成论文摘要