Meta pseudo labels for anomaly detection via partially observed anomalies
Engineering Applications of Artificial Intelligence(2023)
摘要
General anomaly detection has been an important research due to its broad and significant applications. Those algorithms that are based on weakly supervised or partially observed anomalies have attracted particular interest currently. However, most such algorithms treat the unlabeled set as a substitute for normal samples and ignore the potential anomalies in it, which on the one hand causes the noise of the normal set, and on the other hand, fails make full use of the abnormal supervision information. To address this issue, we propose a meta-pseudo-label based framework for anomaly detection (MPAD). The framework strives to obtain effective pseudo anomalies from the unlabeled samples to supplement the observed anomaly set. Specifically, a teacher network is improved based on the feedback of a student network on a validation set, thereby generating more conducive pseudo anomalies to assist the student network while incurring less confirmation bias. In addition, we also design a model roll-back scheme to guarantee the performance of the student network. Extensive experiments show that the proposed MPAD algorithm outperforms current popular algorithms on five real datasets, and the framework can effectively improve the utilization of unlabeled samples while maintaining an advanced degree of robustness. In addition, the results of ablation experiments confirm the effectiveness of each module of our MPAD. Parameter experiments provide sufficient reference for parameter selecting.
更多查看译文
关键词
0000,1111
AI 理解论文
溯源树
样例
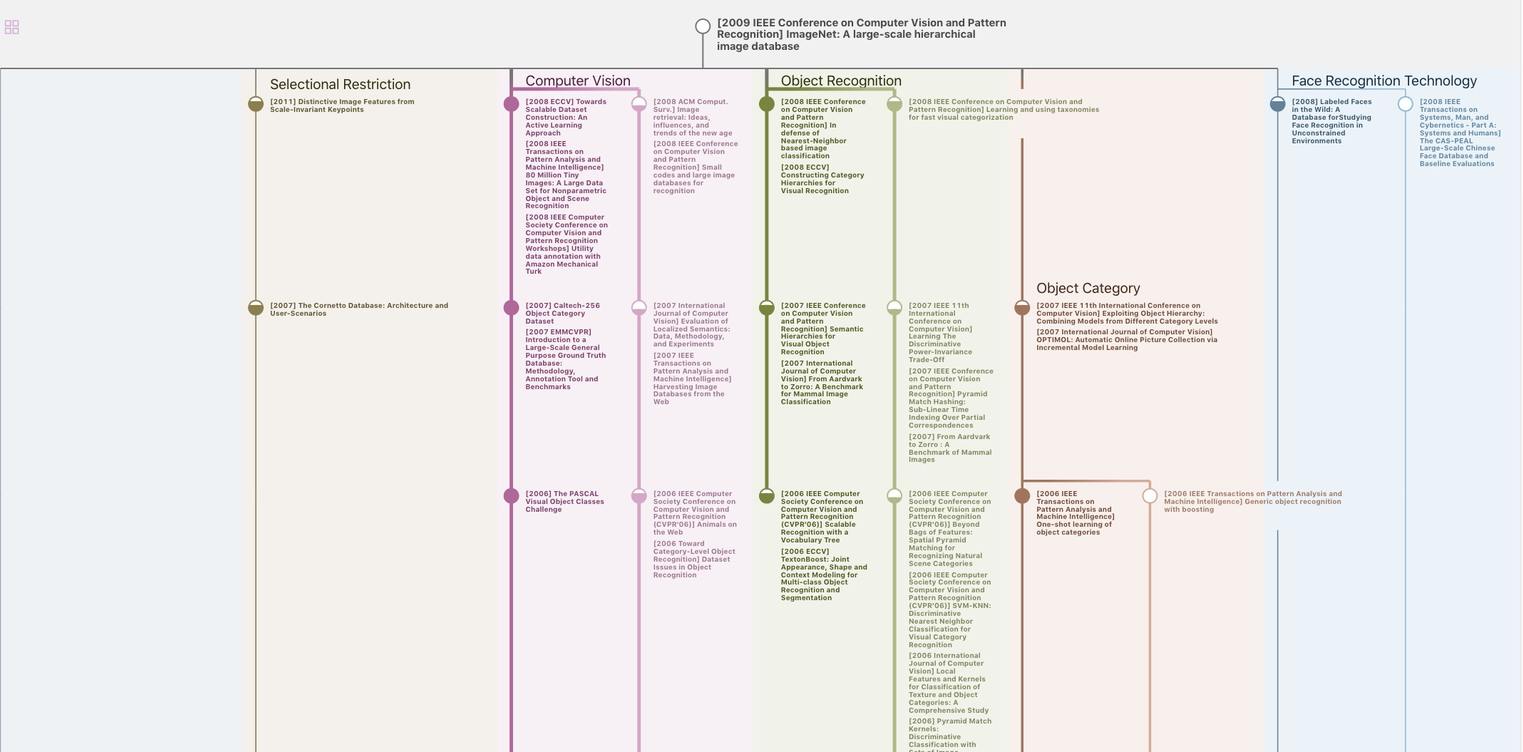
生成溯源树,研究论文发展脉络
Chat Paper
正在生成论文摘要