Semantic-Driven Instance Generation for Table Question Answering.
DASFAA (1)(2023)
摘要
Recent studies exhibit that generating sufficient samples can improve the performance of Table QA, especially in complex cross-domain applications. However, most existing data augmentation approaches for Table QA adopt a top-down paradigm and rely on predefined rules designed by human experts. In this paper, we aim to generate training instances for Table QA while mitigating the dependence on the human experience. We propose an approach coined SIG-TQA in which an off-shelf parsing tool is utilized to extract semantic patterns of SQL queries from text-to-SQL corpus. Then, these semantic patterns are underpinned to generate question/SQL pair with a SQL generator and a natural language question generator, respectively. Both the semantic pattern extraction and question/SQL pair generation are performed based on the original text-to-SQL corpus with few manual efforts injected, our proposed SIG-TQA is making a different bottom-up paradigm. Extensive experiments on a widely-used benchmark and online experiments on a practical industry system demonstrate the superiority of SIG-TQA. Currently, our SIG-TQA has been applied to a real-world Table QA system, and its code is available on https://github.com/DHms2020/SIG-TQA .
更多查看译文
关键词
instance generation,table,semantic-driven
AI 理解论文
溯源树
样例
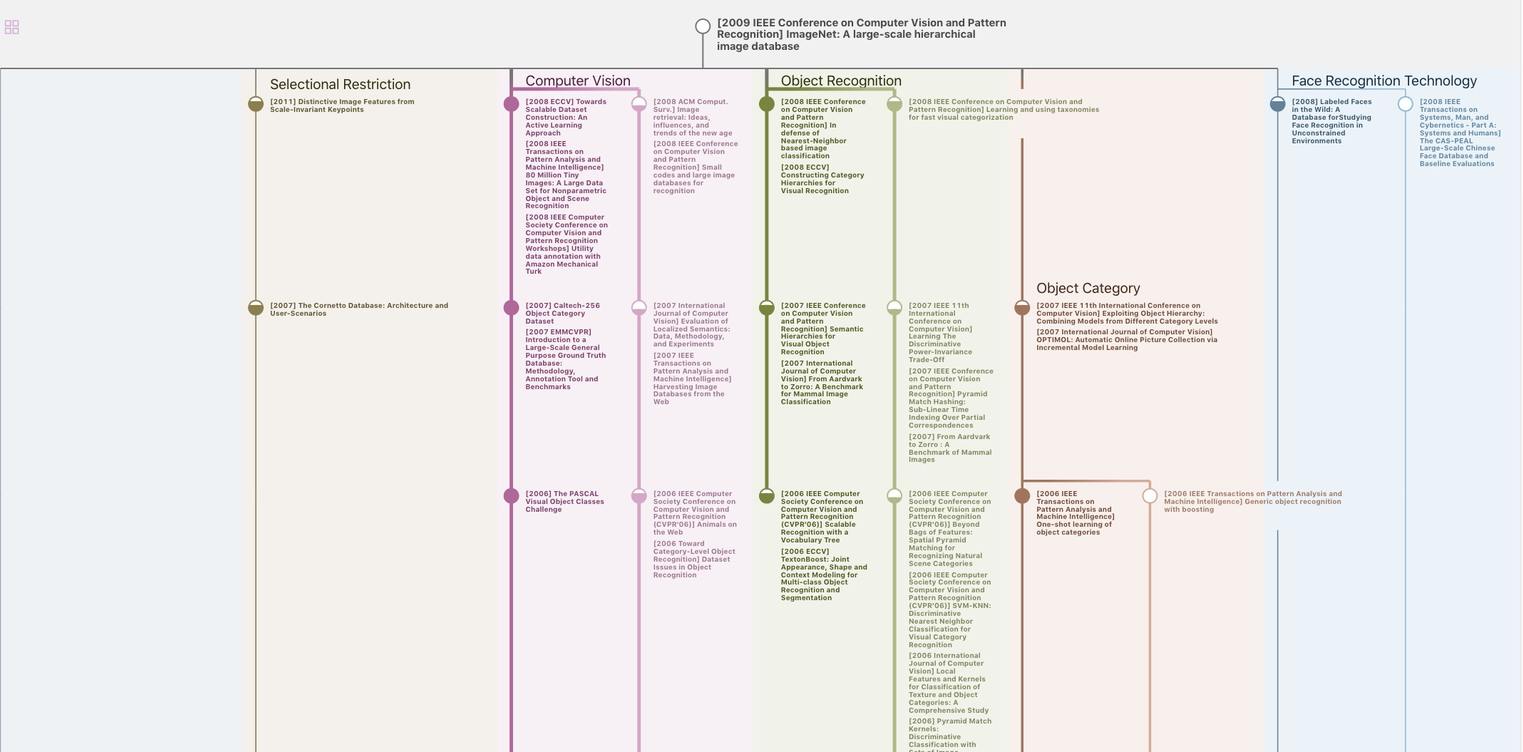
生成溯源树,研究论文发展脉络
Chat Paper
正在生成论文摘要