Towards Efficient and Effective Transformers for Sequential Recommendation.
DASFAA (2)(2023)
摘要
Transformer and its variants have been intensively applied for sequential recommender systems nowadays as they take advantage of the self-attention mechanism, feed-forward network (FFN) and parallel computing capability to generate the high-quality sequence representation. Recently, a wide range of fast, efficient Transformers have been proposed to facilitate sequence modeling, however, the lack of a well-established benchmark might lead to the non-reproducible and even inconsistent results across different works, making it hard to gain rigorous assessments. In this paper, We provide a benchmark for reproducibility and present a comprehensive empirical study on various Transformer-based recommendation approaches, and key techniques or components in Transformers. Based on this study, we propose a hybrid effective and Efficient Transformer variant for sequential Recommendation (ETRec), which incorporates the scalable long- and short-term preference learning, blocks of items aggregating as interests, and parameter-efficient cross-layer sharing FFN. Extensive experiments on six public benchmark datasets demonstrate the advanced efficacy of the proposed approach.
更多查看译文
关键词
effective transformers,efficient
AI 理解论文
溯源树
样例
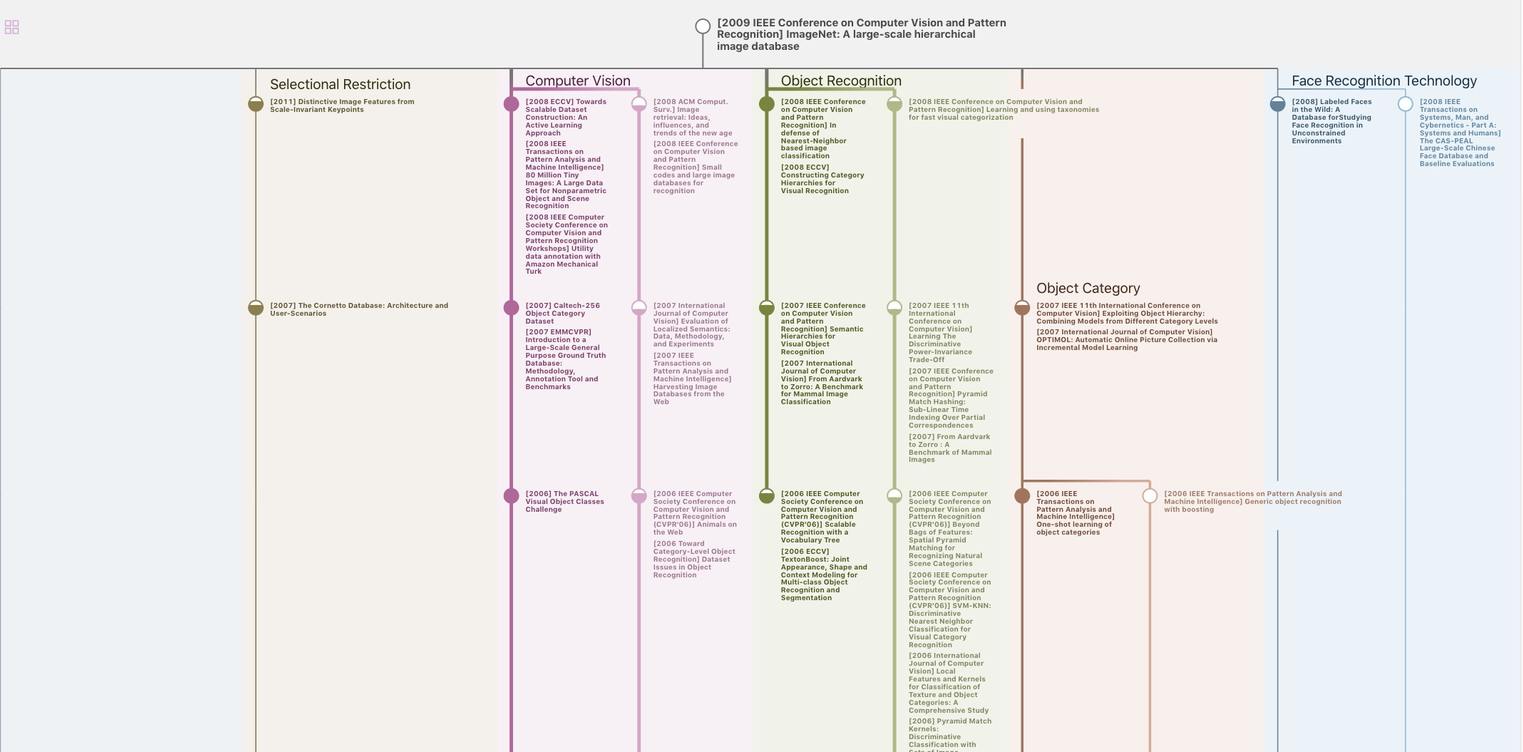
生成溯源树,研究论文发展脉络
Chat Paper
正在生成论文摘要