Modeling High-Order Relation to Explore User Intent with Parallel Collaboration Views.
DASFAA (2)(2023)
摘要
As an emerging paradigm, session-based recommendation (SBR) aims to predict the next item by exploiting user behaviors within a short yet anonymous session. Existing works focus on how to effectively model the information based on graph neural networks, which may be insufficient to capture the high-order relation for short-term interest. To this end, we propose a novel framework, named PacoHGNN, which models high-order relations based on HyperGraph Neural Network with Parallel Collaboration views. Specifically, PacoHGNN learns two embedding views for the SBR task, respectively: (i) item-internal graph view, which is to learn the item embedding by modeling pairwise item connectivities among corresponding items; and (ii) session-external hypergraph view, which targets session embedding by learning beyond pairwise information from high-order relations across all sessions. These two types of graph modeling with data-driven can provide complementary information for each other while exhibiting collaboration to some degree. Additionally, we further propose Hyperedge-to-Node (H2N) to enhance supervised signals against the data sparsity problem for better graph representation. Extensive experiments on multiple real-world datasets demonstrate the superiority of the proposed model over state-of-the-art methods.
更多查看译文
关键词
parallel collaboration views,explore user intent,relation,high-order
AI 理解论文
溯源树
样例
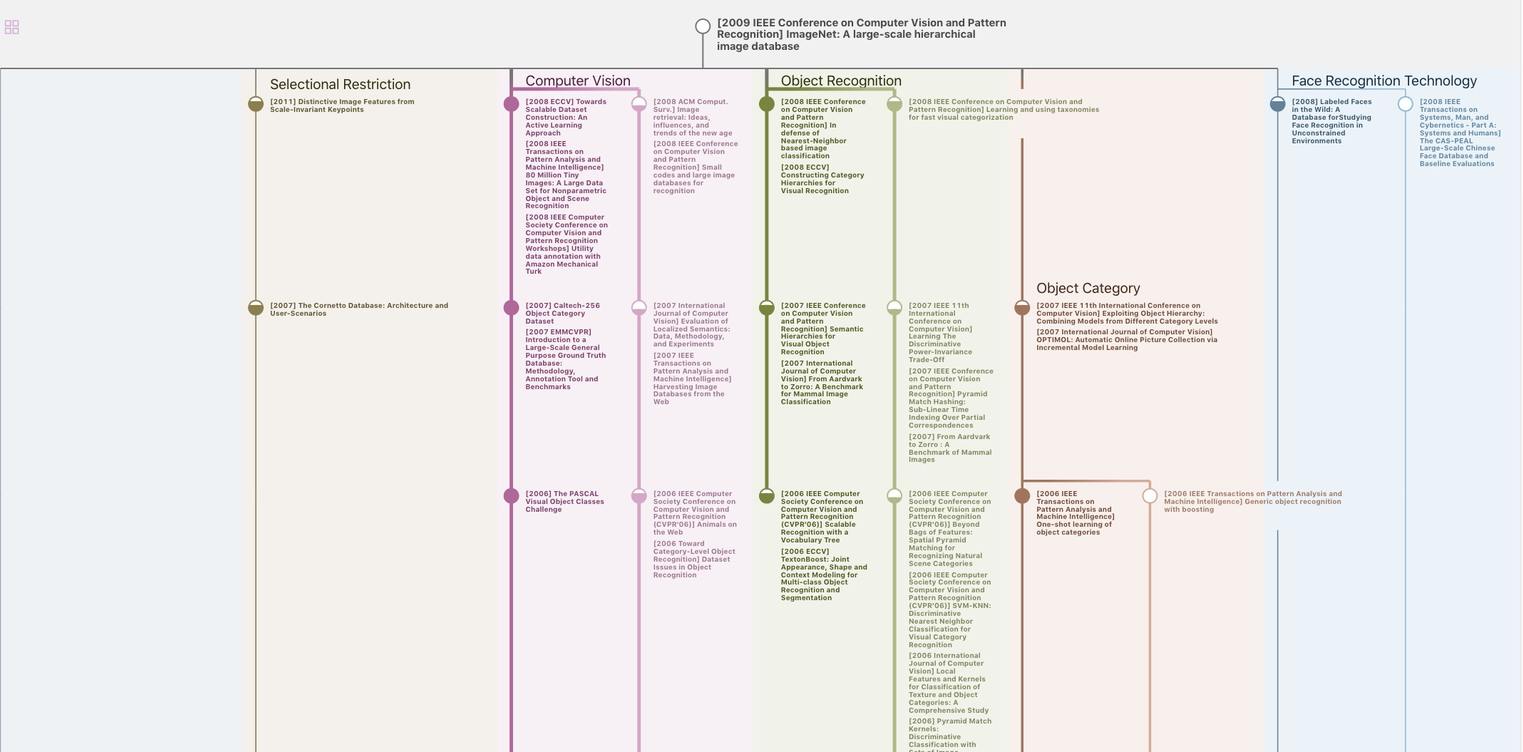
生成溯源树,研究论文发展脉络
Chat Paper
正在生成论文摘要