SNN-AAD: Active Anomaly Detection Method for Multivariate Time Series with Sparse Neural Network.
DASFAA (1)(2023)
摘要
Anomaly detection of time series data is an important and popular problem in both research and application fields. Kinds of solutions have been developed to uncover the anomaly instances from data. However, the labelled data is always limited and costly for real applications, which adds to the difficulty of identifying various anomalies in multivariate time series. In this paper, we propose a novel active anomaly detection method with sparse neural network (SNN-AAD) to improve the accuracy and efficiency in anomaly detection for time series with limited labels. SNN-AAD is designed for two objectives: (1) to achieve sufficient generalization capacity of the model with small-size labels, and (2) to effectively reduce the human cost in active learning process. We introduce sparse neural network in training which minimizes the detection loss caused by the sparsity of labels. We improve the active anomaly detection with the design of sample selection strategy and abnormal feature order generation algorithm, which extracts the important features of instances and reduce the cost of human intelligence. Experimental results on four real-life datasets show SNN-AAD has good detection performance with limited labels, and improves the detection accuracy by 10.2%–18.2% from benchmark methods, while it achieves 12.1% human cost reduction.
更多查看译文
关键词
active anomaly detection method,anomaly detection,multivariate time series,neural network,snn-aad
AI 理解论文
溯源树
样例
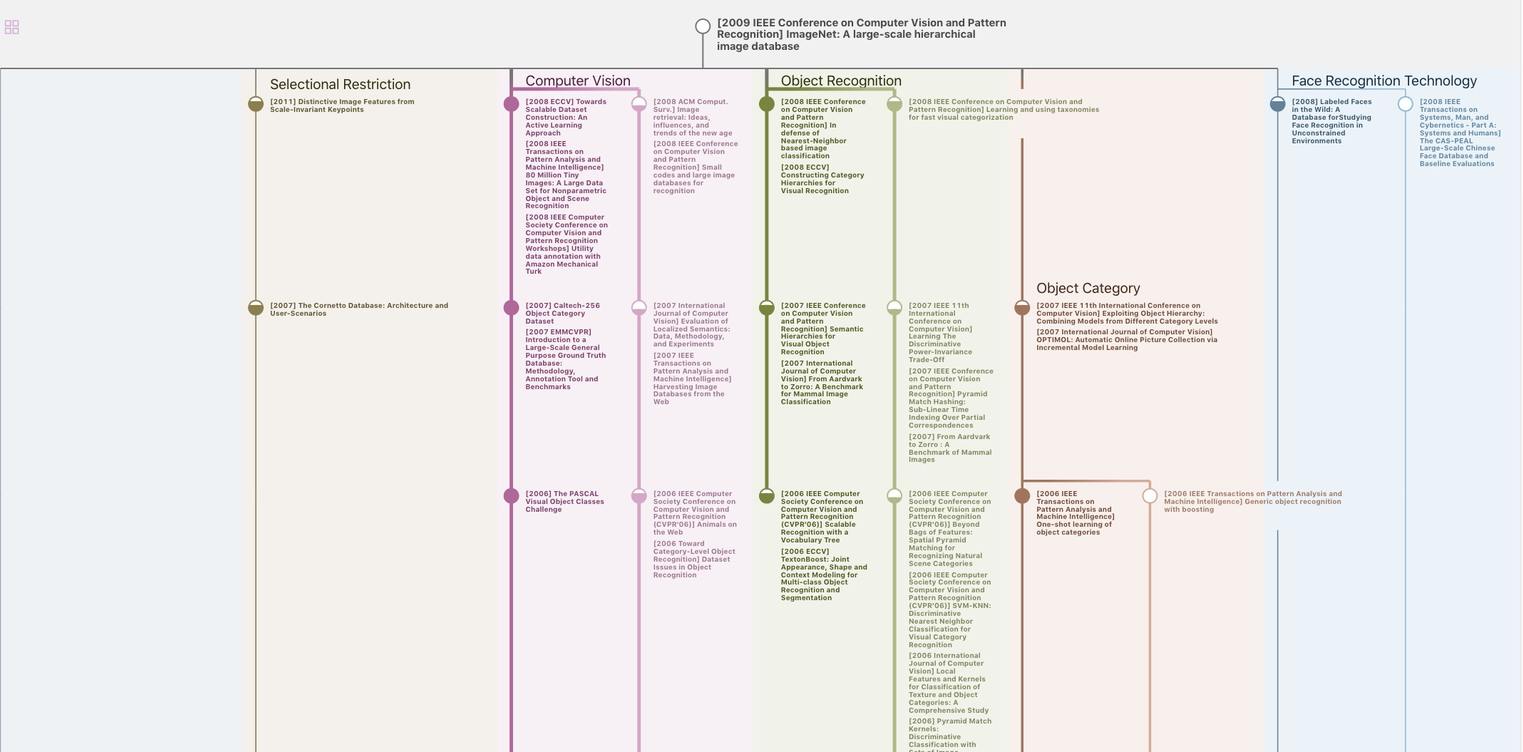
生成溯源树,研究论文发展脉络
Chat Paper
正在生成论文摘要