Remaining useful life prediction combined dynamic model with transfer learning under insufficient degradation data.
Reliab. Eng. Syst. Saf.(2023)
摘要
The remaining useful life (RUL) prediction is critically involved in machinery to ensure safe and reliable operation. Nevertheless, acquiring the full-cycle degradation data is difficult and time-consuming, which hinders the application of the prognostic methods. In view of this problem, this paper proposes an RUL prediction method that combines the dynamic model with transfer learning. Firstly, the dynamic mechanism and the generative adversarial network based on deep autoencoder structure (GAN-DAE) are used to build the simulation model to achieve the accurate simulation of the physical asset state. Then, the defect evolution laws based on multiple nonlinear functions guide the simulation model to generate various types of full-cycle degradation data. Finally, the multiple source-and-target domain joint adaption network (MDJAN) is utilized to build the RUL prediction model, which can apply the generated information to the actual space by eliminating the local distribution discrepancy among individuals. The validity of the method is supported by a case study of bearing with outer race fault under the same- and cross-working conditions. The experimental results indicate that the method presented here can perform more accurate RUL prediction without full-cycle degradation data compared to the state-of-the-art approaches.
更多查看译文
关键词
Remaining useful life prediction, Dynamic model, Generative adversarial network, Deep transfer learning, Insufficient degradation data
AI 理解论文
溯源树
样例
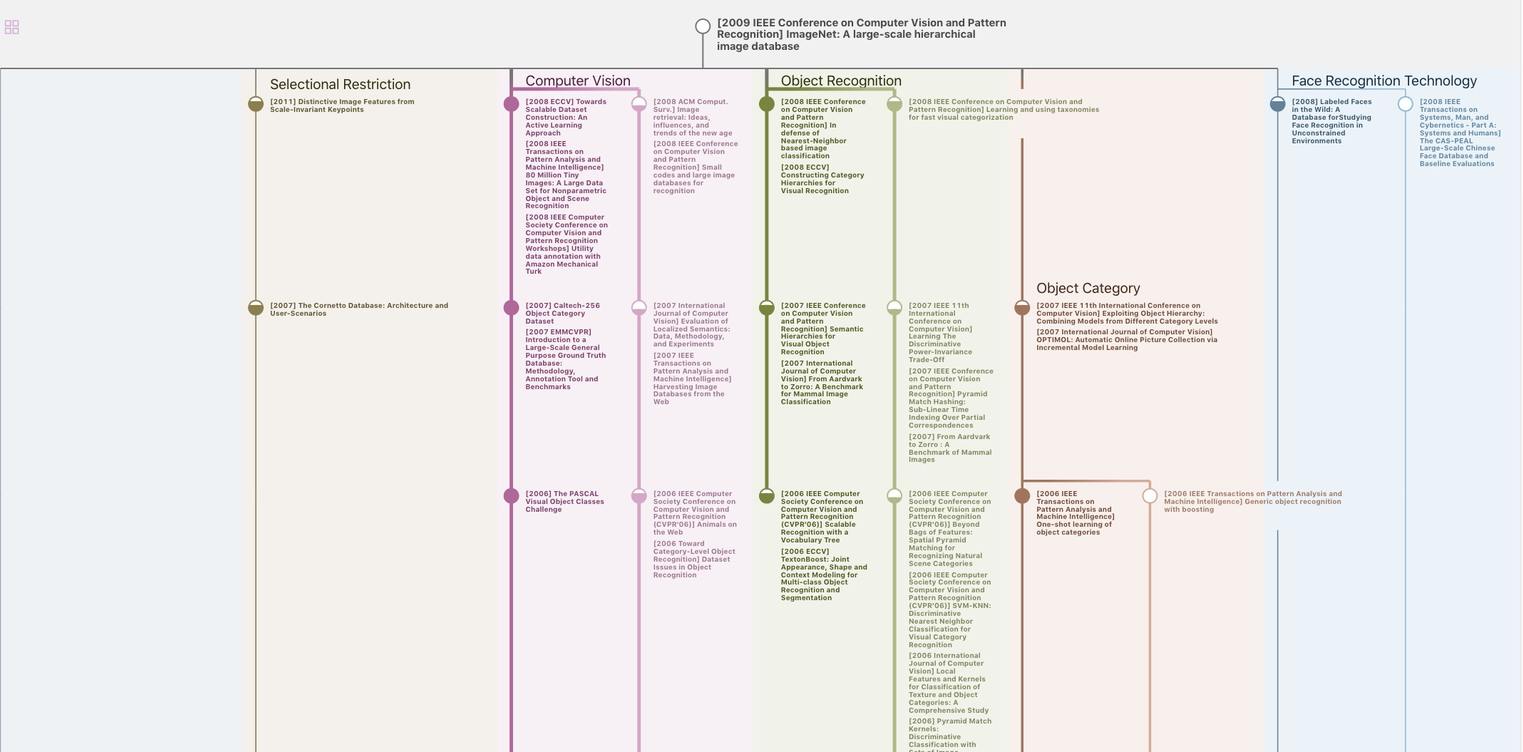
生成溯源树,研究论文发展脉络
Chat Paper
正在生成论文摘要