Noise-Tolerant Radio Frequency Fingerprinting With Data Augmentation and Contrastive Learning.
WCNC(2023)
摘要
Deep learning (DL) based identification systems are deemed as the scalable, accurate and lightweight authentication mechanisms to handle the security provisioning of massive Internet of Things (IoT) systems by leveraging the hardwarelevel radio frequency fingerprints. However, the conventional DL-based methods perform poor generalization in the practical time-varying signal-to-noise ratio (SNR) scenarios. In this paper, we propose a data augmentation and contrastive learning based radio frequency fingerprinting (DACL-RFF) with the joint optimization of samples agreement and labels agreement. First, we expand the SNR variations of training dataset with data augmentation, and then we propose a novel framework of contrastive learning. Specifically, we employ the original samples as the supervisory information of augmented samples and the label information of original samples is leveraged to guide the training process. Experimental results demonstrate that our proposal can increase the average accuracy by up to 51.74% in comparison with the case of none augmentation as the conventional DLbased methods. Additionally, we show that our framework of contrastive learning yields 5.27% improvement compared to the case of data augmentation with supervised learning.
更多查看译文
关键词
Radio frequency fingerprinting, data augmentation, contrastive learning, signal-to-noise ratio
AI 理解论文
溯源树
样例
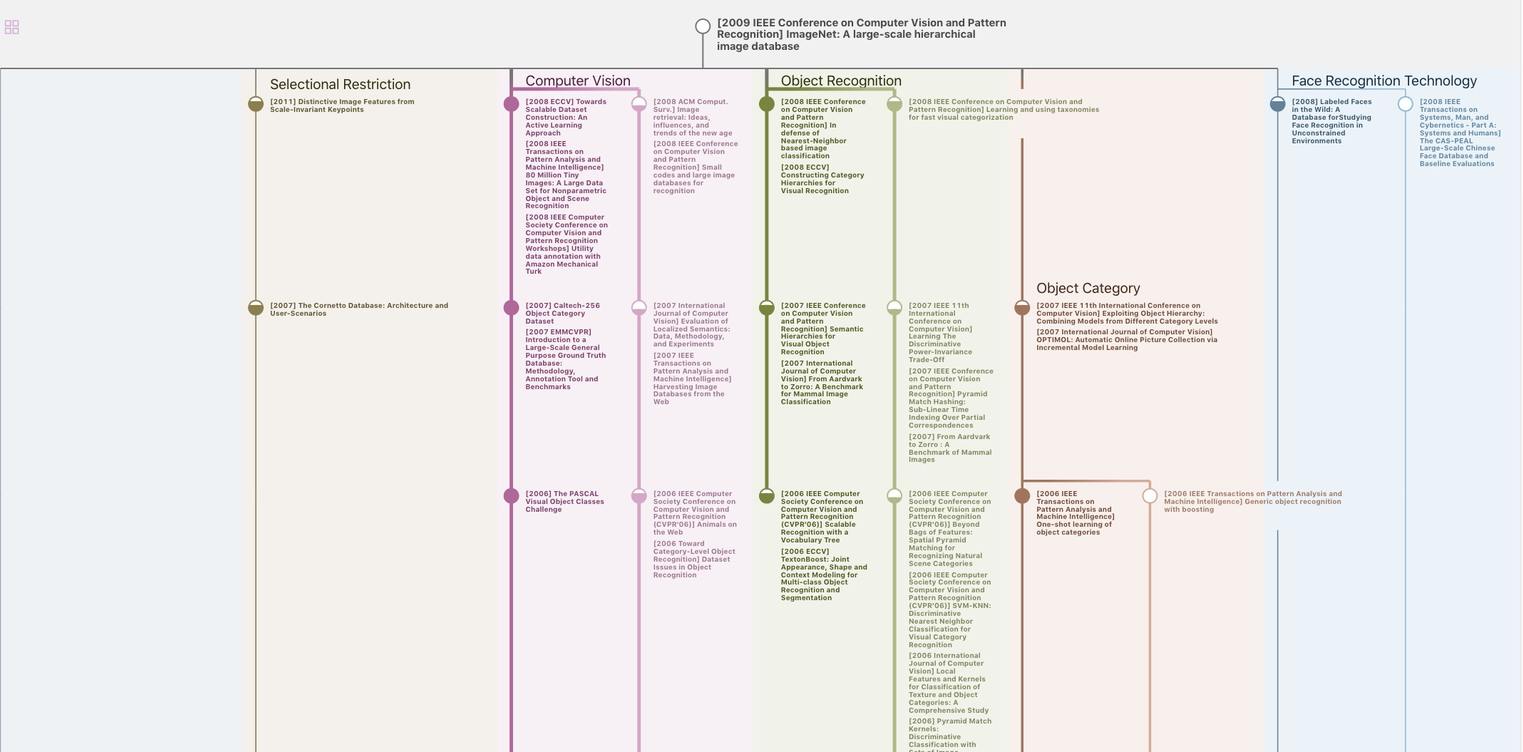
生成溯源树,研究论文发展脉络
Chat Paper
正在生成论文摘要