Maximizing mutual information inside intra- and inter-modality for audio-visual event retrieval
Int. J. Multim. Inf. Retr.(2023)
摘要
The human brain can process sound and visual information in overlapping areas of the cerebral cortex, which means that audio and visual information are deeply correlated with each other when we explore the world. To simulate this function of the human brain, audio-visual event retrieval (AVER) has been proposed. AVER is about using data from one modality (e.g., audio data) to query data from another. In this work, we aim to improve the performance of audio-visual event retrieval. To achieve this goal, first, we propose a novel network, InfoIIM, which enhance the accuracy of intra-model feature representation and inter-model feature alignment. The backbone of this network is a parallel connection of two VAE models with two different encoders and a shared decoder. Secondly, to enable the VAE to learn better feature representations and to improve intra-modal retrieval performance, we have used InfoMax-VAE instead of the vanilla VAE model. Additionally, we study the influence of modality-shared features on the effectiveness of audio-visual event retrieval. To verify the effectiveness of our proposed method, we validate our model on the AVE dataset, and the results show that our model outperforms several existing algorithms in most of the metrics. Finally, we present our future research directions, hoping to inspire relevant researchers.
更多查看译文
关键词
Audio-visual retrieval, Variational autoencoder, Mutual information, InfoMax-VAE
AI 理解论文
溯源树
样例
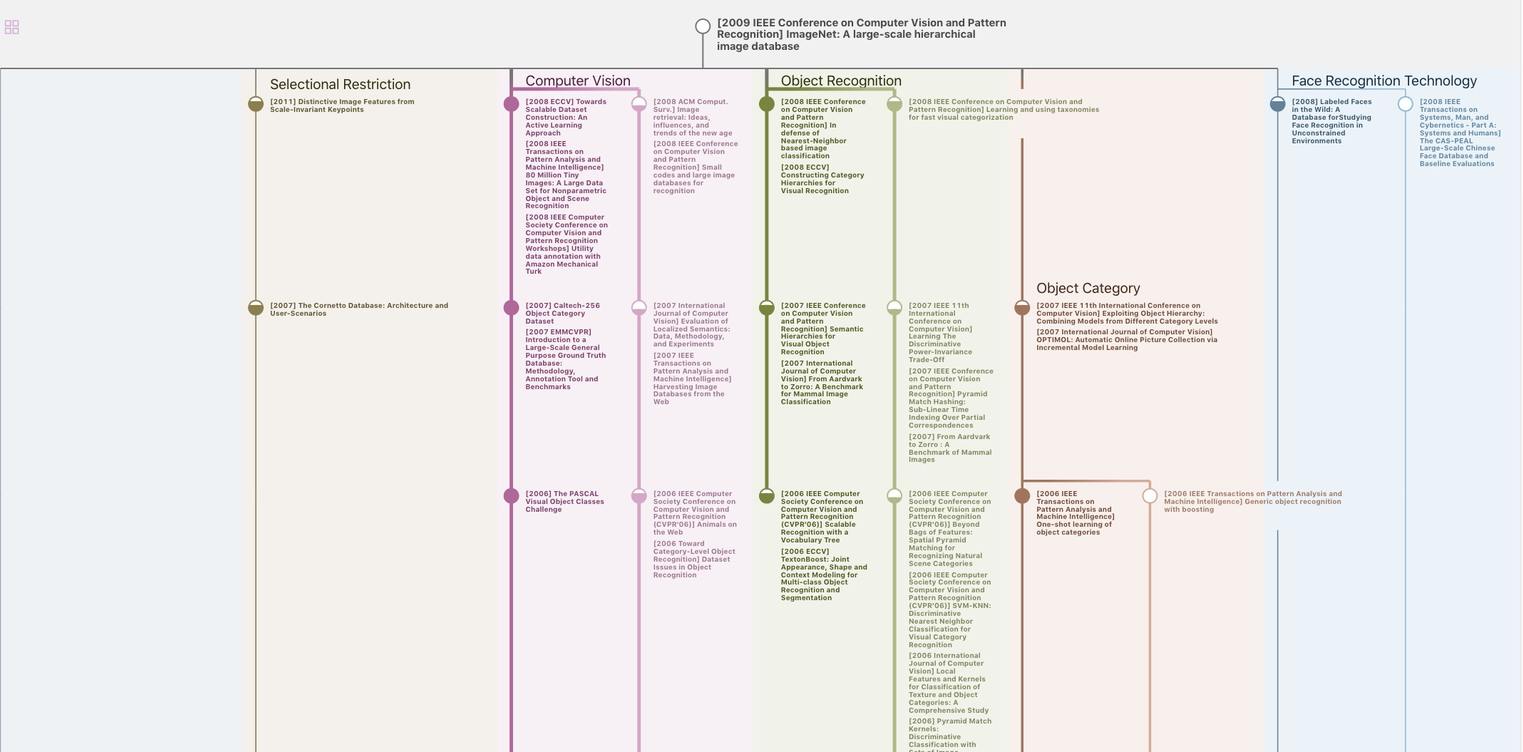
生成溯源树,研究论文发展脉络
Chat Paper
正在生成论文摘要