FLASH: Towards a High-performance Hardware Acceleration Architecture for Cross-silo Federated Learning.
NSDI(2023)
摘要
Cross-silo federated learning (FL) adopts various cryptographic operations to preserve data privacy, which introduces significant performance overhead. In this paper, we identify nine widely-used cryptographic operations and design an efficient hardware architecture to accelerate them. However, directly offloading them on hardware statically leads to (1) inadequate hardware acceleration due to the limited resources allocated to each operation; (2) insufficient resource utilization, since different operations are used at different times. To address these challenges, we propose FLASH, a high-performance hardware acceleration architecture for cross-silo FL systems. At its heart, FLASH extracts two basic operators-modular exponentiation and multiplication-behind the nine cryptographic operations and implements them as highly-performant engines to achieve adequate acceleration. Furthermore, it leverages a dataflow scheduling scheme to dynamically compose different cryptographic operations based on these basic engines to obtain sufficient resource utilization. We have implemented a fully-functional FLASH prototype with Xilinx VU13P FPGA and integrated it with FATE, the most widely-adopted cross-silo FL framework. Experimental results show that, for the nine cryptographic operations, FLASH achieves up to 14:0x and 3:4x acceleration over CPU and GPU, translating to up to 6:8x and 2:0x speedup for realistic FL applications, respectively. We finally evaluate the FLASH design as an ASIC, and it achieves 23:6x performance improvement upon the FPGA prototype.
更多查看译文
AI 理解论文
溯源树
样例
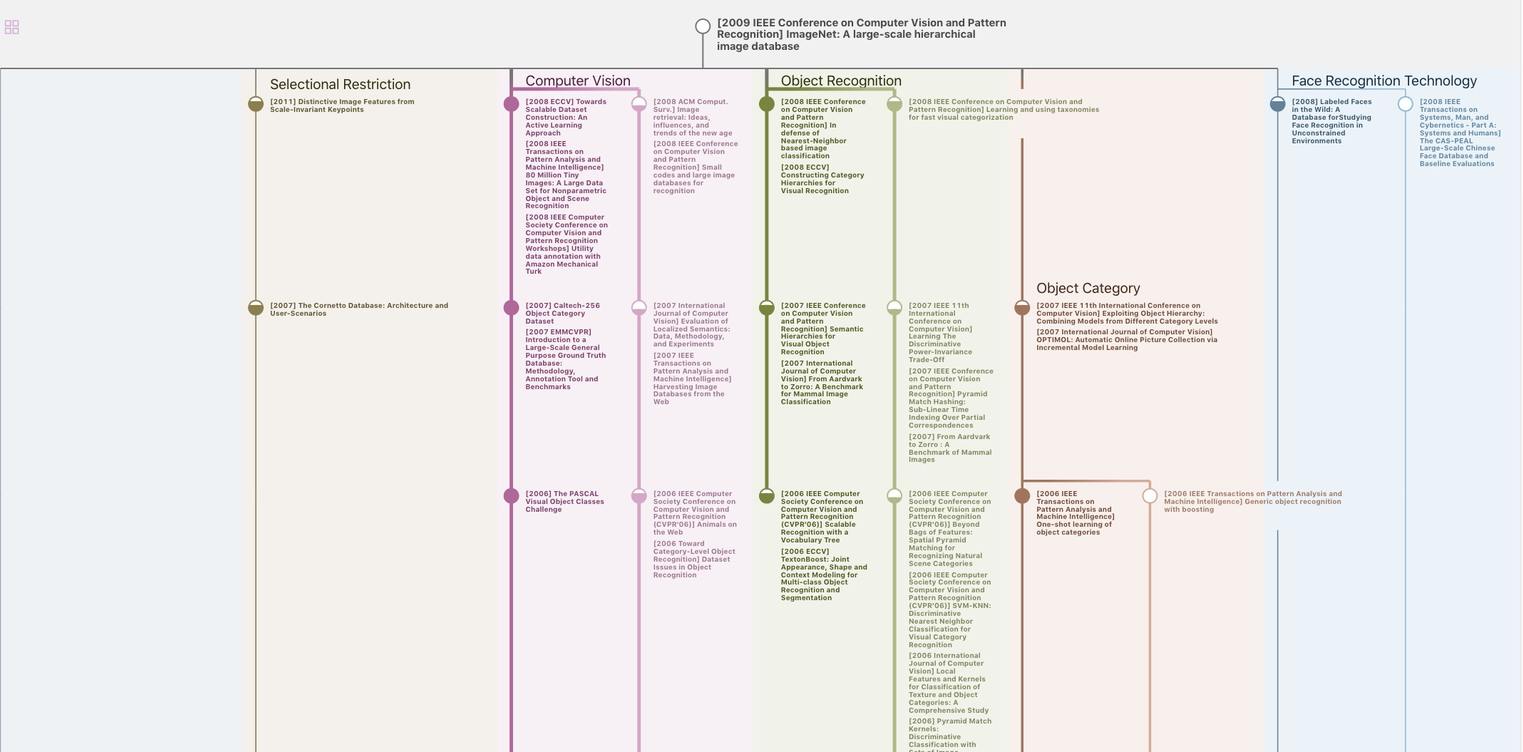
生成溯源树,研究论文发展脉络
Chat Paper
正在生成论文摘要