Federated learning based driver recommendation for next generation transportation system
Expert Syst. Appl.(2023)
摘要
Driving behavior analysis benefits the transportation system in terms of road safety, maintenance costs, vehicle's off-road time, fuel consumption, and enhanced driving experiences. In this paper, we present FedSafe, a driver stress and behavior-based recommendation system using federated learning (FL). The proposed system recognizes the drivers' stressed conditions and driving behavior using vehicle telematics and physiological data to recommend the driver for the next trip, ensuring stress-free and safe driving behavior. Here, federated learning enables collaborative learning from a large amount of data belonging to different vehicles without sharing the raw data among the drivers, ensuring reduced data transmission overhead by allowing computation on the end devices, which helps to meet the increased demand for computing on the edge of the vehicular network. The extensive human-in-the-loop study on three publicly available natural driving datasets (i.e., UAH DriveSet, HCI Lab, PhysioNet) shows that the proposed technique can predict driver stress and identifies the behavior with an accuracy of 97% and 98%, respectively, in terms of AUC and F-measure with approximately 25 times lower transmission overhead using FL.
更多查看译文
关键词
driver recommendation,transportation
AI 理解论文
溯源树
样例
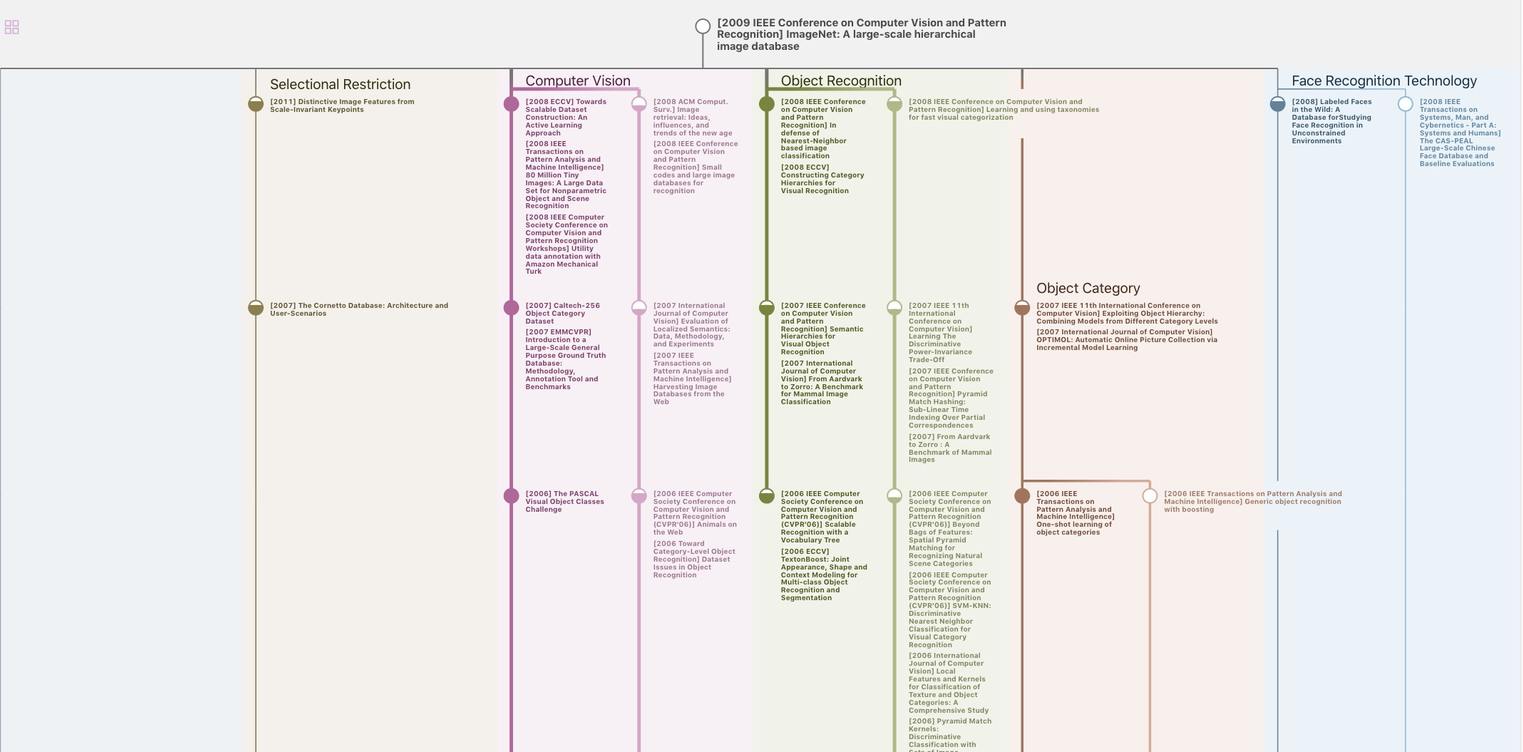
生成溯源树,研究论文发展脉络
Chat Paper
正在生成论文摘要