Deep probability multi-view feature learning for data clustering.
Expert Syst. Appl.(2023)
摘要
Data in the real world is often represented by multi-view or multi-modality. Single view data of the sample is usually not comprehensive enough, yet multi-view data can favorably describe the characteristics of the samples through complementing to each other. Thus, many researchers pay more attention to the field of multi-view data clustering. Some researchers explore non-negative matrix factorization (NMF) based techniques and subspace learning ideas for multi-view clustering. However, these methods usually have difficulty to tackle noise within data, meanwhile most of them are not robust enough without considering the distribution of the original data. Thus, in this paper, we propose a novel deep probability multi-view feature learning (DPMFL) method to tackle these problems. Specifically, we design a probabilistic matrix factorization (PMF) algorithm, which assumes the data obey Gaussian distribution during noise and dimension reduction, for data preprocessing. Moreover, considering the success of Deep Neural Network (DNN) in the field of machine learning, we integrate DNN with PMF and subspace self-representation for effective consistent and specific multi-view feature learning. A new objective function is thus obtained and the solution processes are presented. Experiments on five popular datasets demonstrate the effectiveness of our proposed method compared with nine state-of-the-art approaches in terms of four evaluation metrics.
更多查看译文
关键词
Multi-view clustering, Subspace learning, Auto-encoder, Probability non-negative matrix factorization
AI 理解论文
溯源树
样例
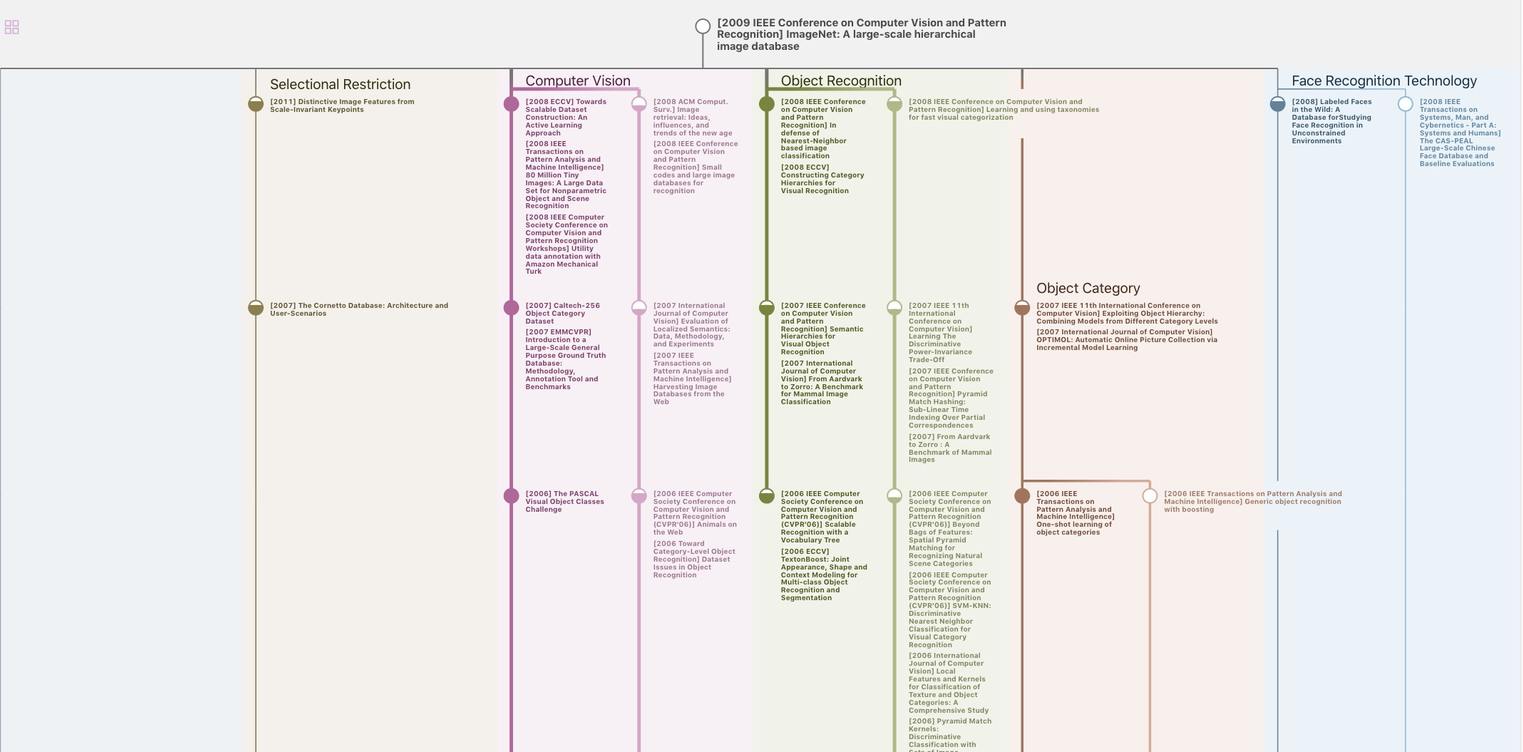
生成溯源树,研究论文发展脉络
Chat Paper
正在生成论文摘要