LHP: Logical hypergraph link prediction.
Expert Syst. Appl.(2023)
摘要
Logical knowledge mining has become increasingly important as it is the foundation of precise reasoning, such as for clinical diagnosis or chemical reaction discovery. Knowledge hypergraphs provide a natural format for expressing inherently high-order relationships beyond pairwise associations. Although logical knowledge (the conjunct operator in first-order logic) is apparently a higher-order relation that can be represented by a hypergraph, methods of representing logical knowledge in this form and completing knowledge by hyperlink prediction have not yet been explored. In this study, logical knowledge is represented by directed hyperedges and effectively quantified by geometric operations in a neural network. The proposed logical hyperlink predictor (LHP) leverages logical knowledge features including permutation invariant and information aggregation of the logical 'conjunct' operation. LHP captures logical representation as a whole unit containing the different rela-tionship types embedded within separate hyperedges, making it the novel method to integrate logical knowledge contained within hyperedges. We conduct extensive experiments on the iAF1260b, iJO1366, USPTO, and our Chinese Medical High-order Relational (CMHR) dataset. LHP achieved best performance with linear complexity compared to the state-of-the-art hyperlink prediction methods on a mean area under the receiver operating characteristic curve (AUC), Macro_F1 and accuracy. It was particularly effective on the CMHR demonstrating the importance of logical knowledge representation in the medical field. LHP also achieved a mean AUC of 0.924 in performing hyperedge relationship classification on the CMHR.
更多查看译文
关键词
logical hypergraph link prediction
AI 理解论文
溯源树
样例
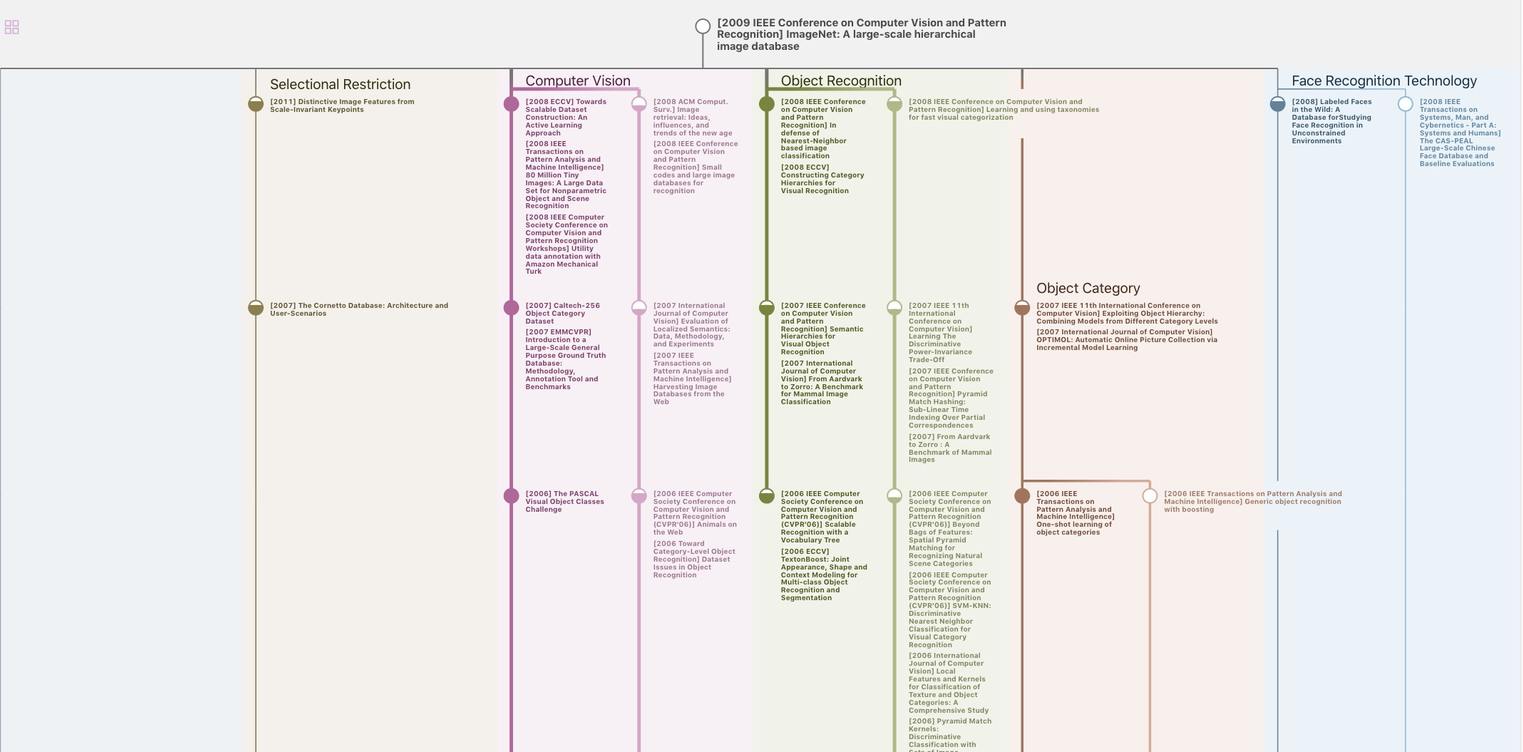
生成溯源树,研究论文发展脉络
Chat Paper
正在生成论文摘要