Novelty detection for multi-label stream classification under extreme verification latency.
Appl. Soft Comput.(2023)
摘要
Multi-Label Stream Classification (MLSC) is the classification streaming examples into multiple classes simultaneously. Since new classes may emerge during the streaming process (concept evolution) and known classes may change over time (concept drift) it is challenging task. In real situations, concept drift and concept evolution occur in scenarios where the actual labels of arriving examples are never available; hence it is impractical to update decision models in a supervised fashion. This is known as Extreme Verification Latency, a topic that has not been well investigated in MLSC literature. This paper proposes a new method called MultI-label learNing Algorithm for Data Streams with Binary Relevance transformation (MINAS-BR), integrated with a Novelty Detection (ND) procedure for detecting concept evolution and concept drift, updating the model in an unsupervised fashion. Furthermore, since the label space is not static, we propose a new evaluation methodology for MLSC under extreme verification latency. Experiments over synthetic and real-world data sets with different concept drift and concept evolution scenarios confirmed the strategies employed in the MINAS-BR and presented relevant advances for handling streaming multi-label data.
更多查看译文
关键词
Concept evolution,Extreme verification latency,Multi-label stream classification,Novelty detection
AI 理解论文
溯源树
样例
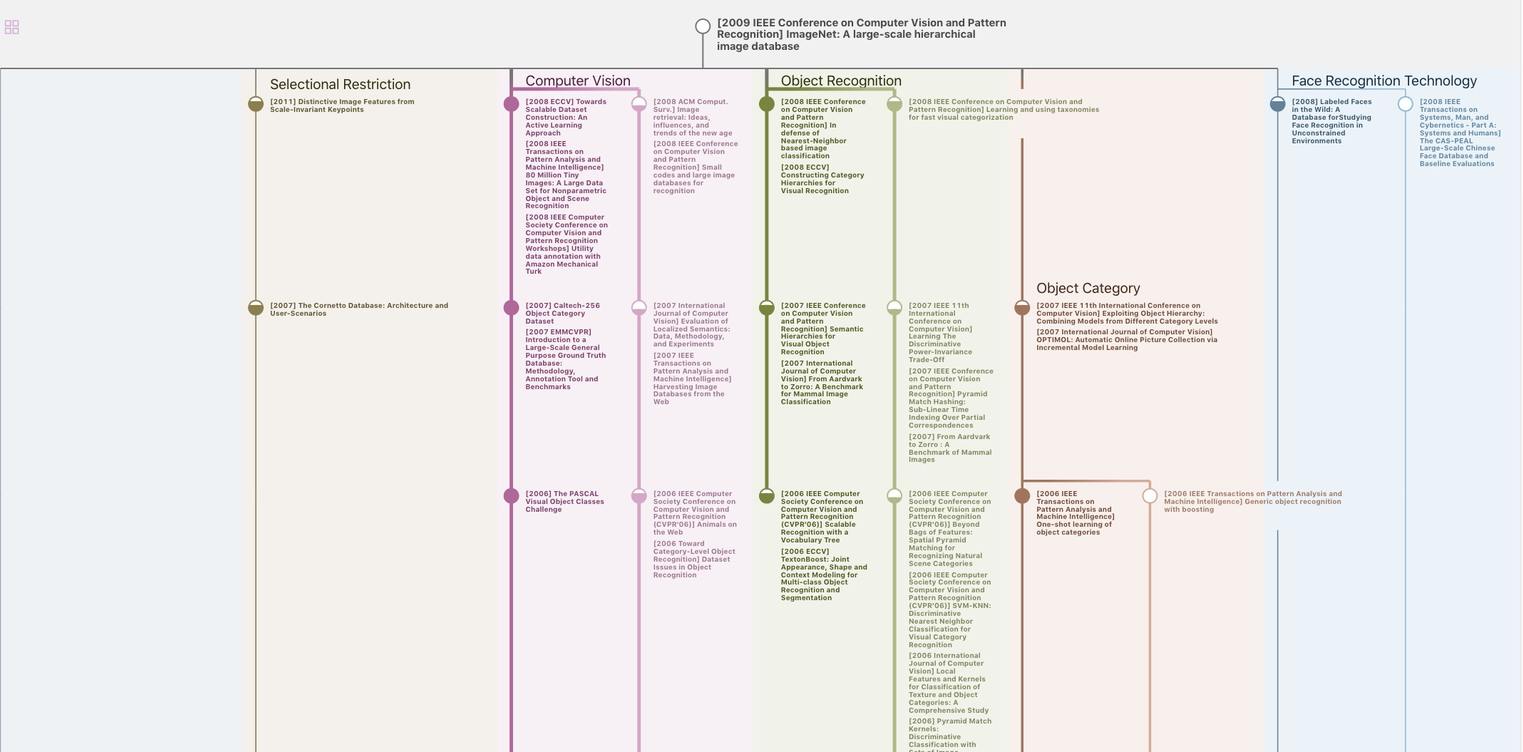
生成溯源树,研究论文发展脉络
Chat Paper
正在生成论文摘要