Surface stress monitoring of laser shock peening using AE time-scale texture image and multi-scale blueprint separable convolutional networks with attention mechanism.
Expert Syst. Appl.(2023)
摘要
The quality monitoring of the laser shock peening (LSP) process is of great significance for improving the in-telligence of precision manufacturing. At present, the monitoring method combining acoustic emission (AE) and machine learning inevitably faces the problems of large data samples, lack of artificial feature experience, large parameter redundancy and slow recognition speed. To overcome these limitations, this paper proposes a new method of LSP monitoring combining AE time-scale texture images and multi-scale blueprint separable con-volutional networks with attention mechanism (MBSCN-AM). Firstly, this method discards the tedious and lossy manual feature extraction process, and integrates the time scale information into the texture image. Secondly, a feature extraction module of multi-scale convolution and a feature selection module of the attention mechanism are designed using advanced blueprint separable convolution as the underlying architecture. The multi-scale convolution kernel optimizes the balance between details and overall information through different receptive fields. The adjustment of high-level features is made possible by the attention mechanism, which evaluates and adaptively assigns weights. To verify the validity of the proposed method, LSP experiments were designed to collect AE signals at six different laser energies. The experimental results show that the proposed method can effectively distinguish between different stress states on the material surface compared to other existing moni-toring methods. On the premise of faster calculation and lower storage requirements, the proposed method has higher recognition accuracy and stability. Furthermore, the operation mechanism of the proposed method is elaborated through ablation experiments and visual analysis.
更多查看译文
关键词
Laser shock peening,Acoustic emission,Deep learning,Quality monitoring,Blueprint separable convolutions
AI 理解论文
溯源树
样例
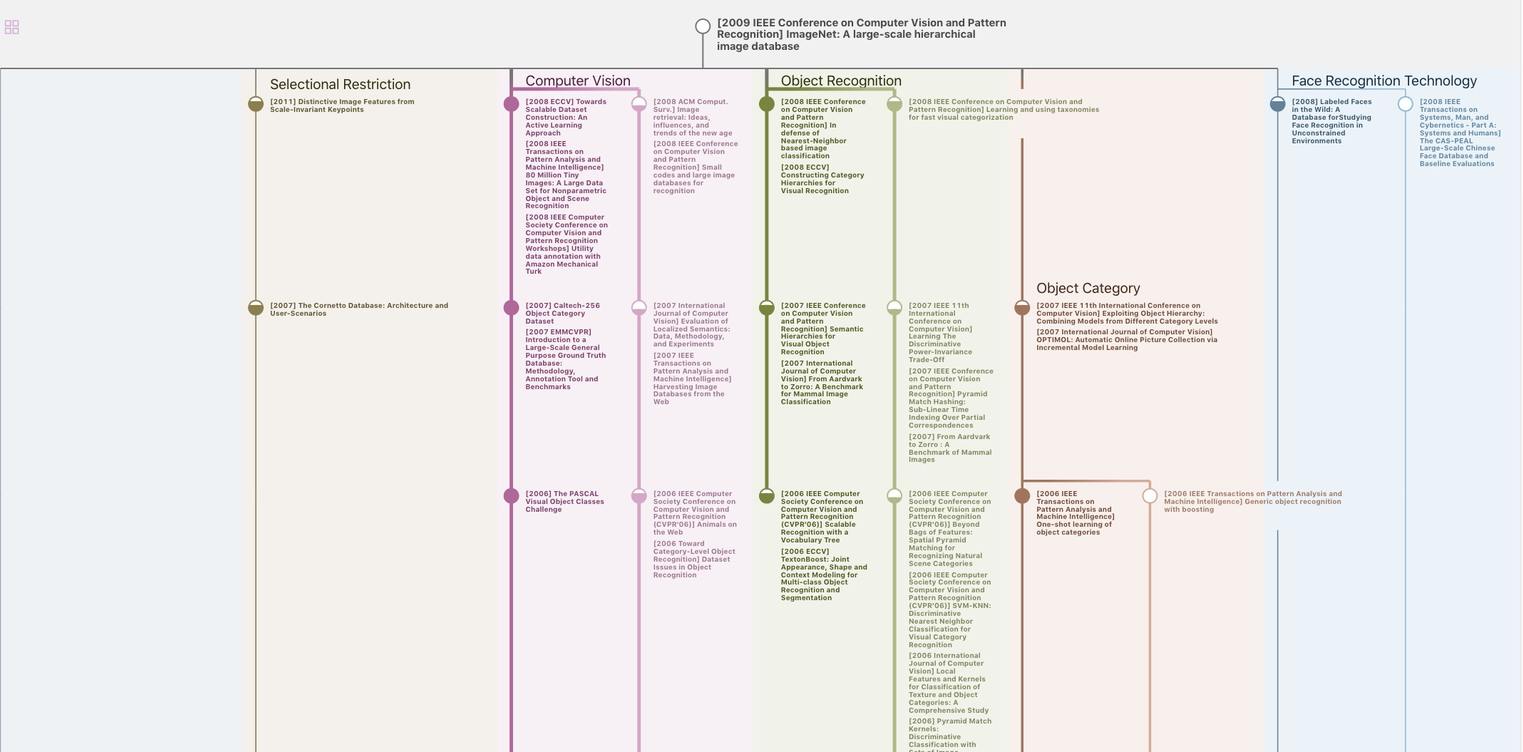
生成溯源树,研究论文发展脉络
Chat Paper
正在生成论文摘要