Contextual combinatorial bandit on portfolio management.
Expert Syst. Appl.(2023)
摘要
Portfolio optimization is a classic problem in finance, and assumptions are generally accepted about the stochastic dynamics of prices and market information. The Linear Upper Confidence Bound (LinUCB) algorithm based on bandit learning is a typical reinforcement learning approach that can be a data-driven approach to the portfolio problem. Most investors may have inconsistent preferences for different assets; therefore, the reward function needs to be designed to balance exploration (allocating part of the investment to searching among other alternative portfolios) and exploitation (concentrating the investment on the historically best portfolio). The study consists of developing a two-stage investing strategy that uses the supervised adaptive decision tree approach to build a pool of candidate portfolios and the reinforcement LinUCB algorithm to derive a portfolio update rule with respect to a specific utility function. The two-stage strategy has been tested on CSI300 constitute stocks listed on the Shanghai Stock Exchange. The accumulated returns, as well as other performance measures (e.g., maximum drawdown, Sharpe ratio, and information ratio) at different testing periods achieved by the model, can well outperform the benchmark index.
更多查看译文
关键词
contextual combinatorial bandit,management
AI 理解论文
溯源树
样例
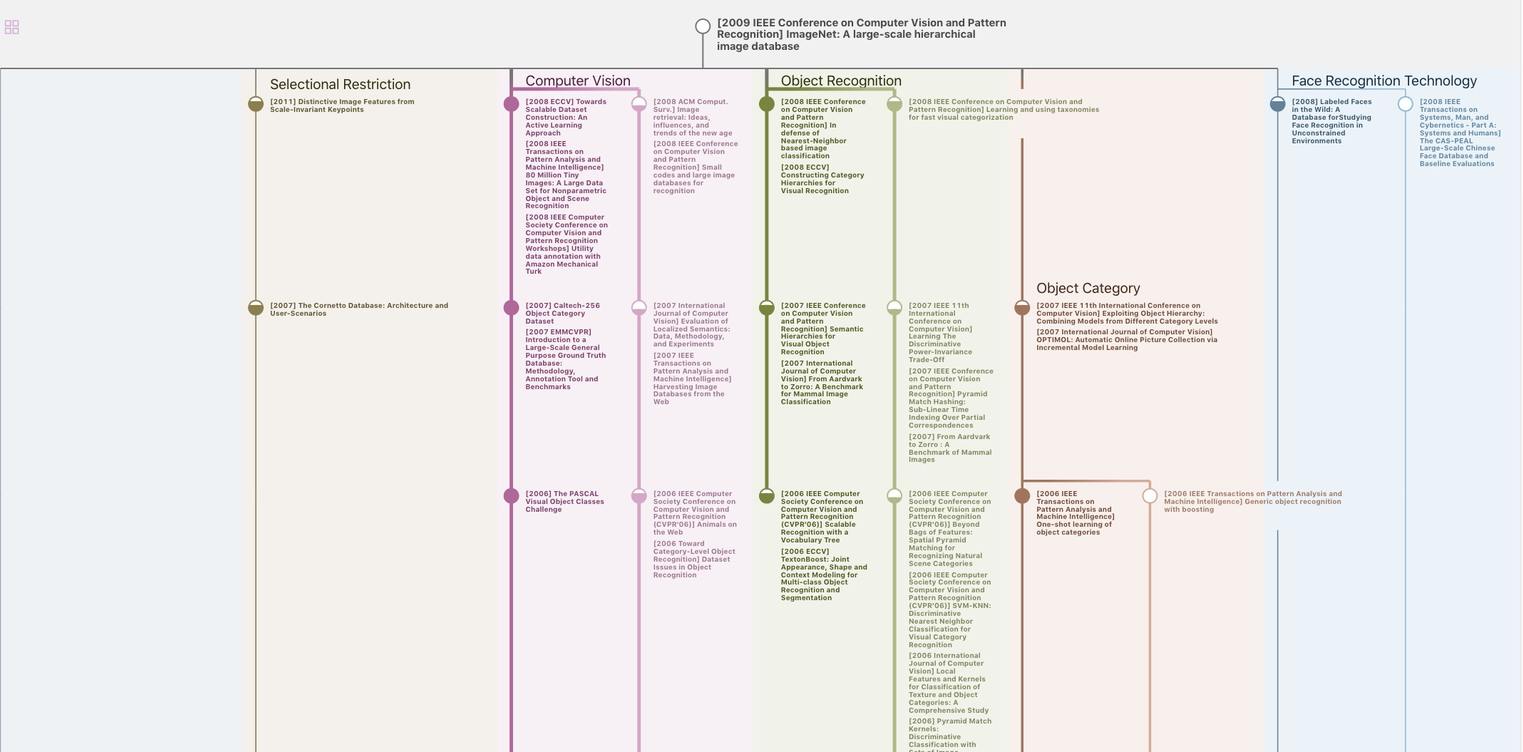
生成溯源树,研究论文发展脉络
Chat Paper
正在生成论文摘要