Face deidentification with controllable privacy protection.
Image Vis. Comput.(2023)
摘要
Privacy protection has become a crucial concern in today's digital age. Particularly sensitive here are facial images, which typically not only reveal a person's identity, but also other sensitive personal information. To address this problem, various face deidentification techniques have been presented in the literature. These techniques try to remove or obscure personal information from facial images while still preserving their usefulness for further analysis. While a considerable amount of work has been proposed on face deidentification, most state-of-theart solutions still suffer from various drawbacks, and (a) deidentify only a narrow facial area, leaving potentially important contextual information unprotected, (b) modify facial images to such degrees, that image naturalness and facial diversity is suffering in the deidentify images, (c) offer no flexibility in the level of privacy protection ensured, leading to suboptimal deployment in various applications, and (d) often offer an unsatisfactory tradeoff between the ability to obscure identity information, quality and naturalness of the deidentified images, and sufficient utility preservation. In this paper, we address these shortcomings with a novel controllable face deidentification technique that balances image quality, identity protection, and data utility for further analysis. The proposed approach utilizes a powerful generative model (StyleGAN2), multiple auxiliary classification models, and carefully designed constraints to guide the deidentification process. The approach is validated across four diverse datasets (CelebA-HQ, RaFD, XM2VTS, AffectNet) and in comparison to 7 state-of-the-art competitors. The results of the experiments demonstrate that the proposed solution leads to: (a) a considerable level of identity protection, (b) valuable preservation of data utility, (c) sufficient diversity among the deidentified faces, and (d) encouraging overall performance. (c) 2023 The Author(s). Published by Elsevier B.V. This is an open access article under the CC BY license (http:// creativecommons.org/licenses/by/4.0/).
更多查看译文
关键词
Face deidentification,Privacy protection,Data utility,Privacy-enhancing technologies,Face biometrics,Deep learning
AI 理解论文
溯源树
样例
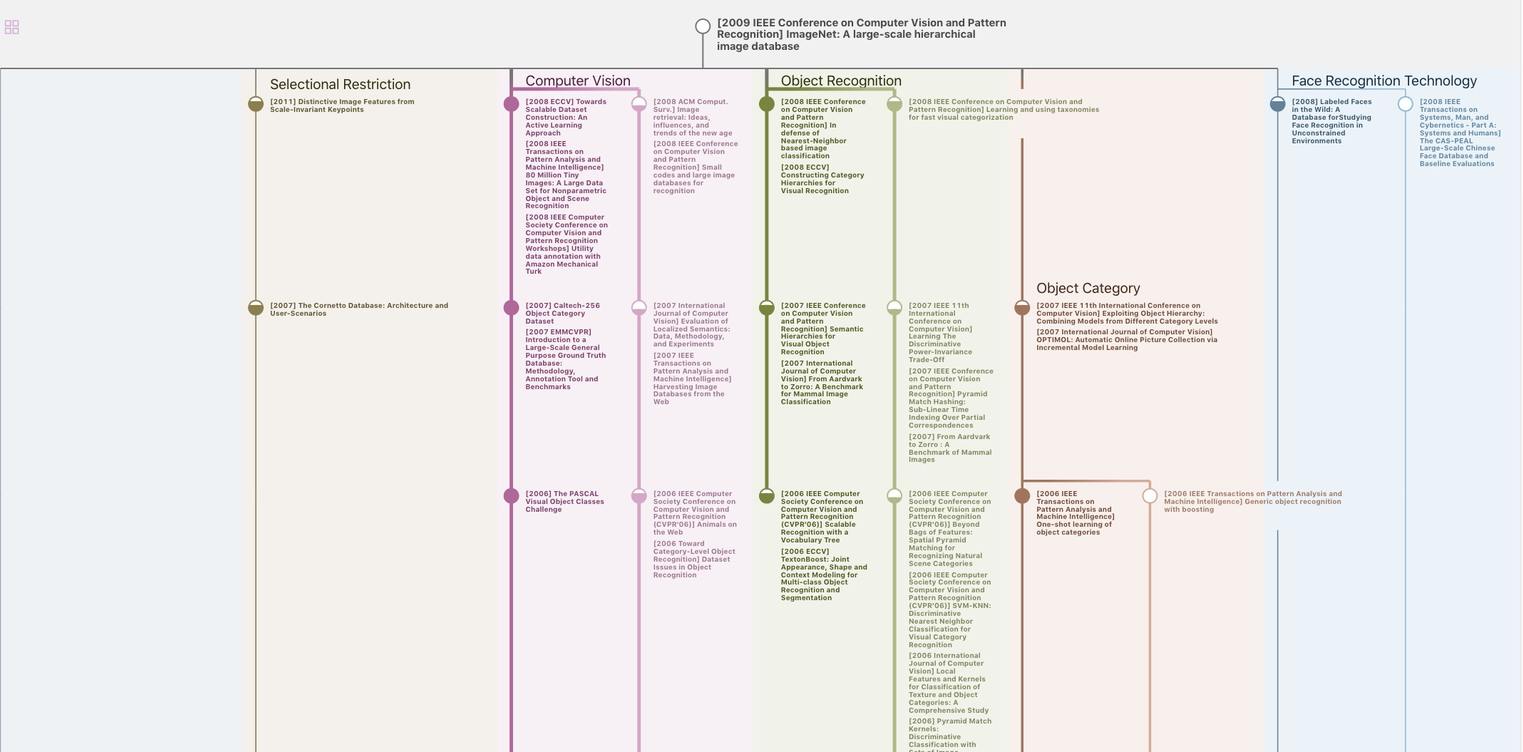
生成溯源树,研究论文发展脉络
Chat Paper
正在生成论文摘要