Building energy performance prediction: A reliability analysis and evaluation of feature selection methods.
Expert Syst. Appl.(2023)
摘要
The advancement of smart meters using evolving technologies such as the Internet of Things (IoT) is producing more data for the training of energy prediction models. Since many machine learning techniques were not premeditated to handle a large number of irrelevant features, it has engendered the search for optimal techniques to decrease the generated features and potentially identify the most relevant features that have an impact on building energy efficiency. Feature selection is considered one of the most suitable methods of pinpointing the best features combination. However, the fraction of studies that deliver comprehensive insights on the incor-poration of feature selection with machine learning is still limited, notwithstanding the capabilities of feature selection to produce a good result in terms of accuracy and speed. To address this gap, this study investigates feature selection methods centred on building energy consumption prediction using machine learning. This study conducted a comparative analysis of 14 machine learning algorithms on 5 different data sizes and explored the effect of 7 feature selection methods on model performance for predicting energy consumption in buildings. Furthermore, this study identifies the most effective feature selection methods and machine learning models for energy use prediction. The experimental results demonstrate that feature selection can affect model's perfor-mance positively or negatively, depending on the algorithm employed. Nevertheless, the filter method was noted as the most appropriate method for most Machine Learning (ML) classification algorithms. Moreover, Gradient Boosting (GB) was identified as the most effective model for predicting energy performance in buildings. Additionally, the reliability analysis confirms the hypothesis that "the larger the data, the more accurate the result" but only for specific algorithms such as Deep Neural Networks (DNN). This study also presents the theoretical and practical implications of this research.
更多查看译文
关键词
energy performance prediction,reliability analysis,building
AI 理解论文
溯源树
样例
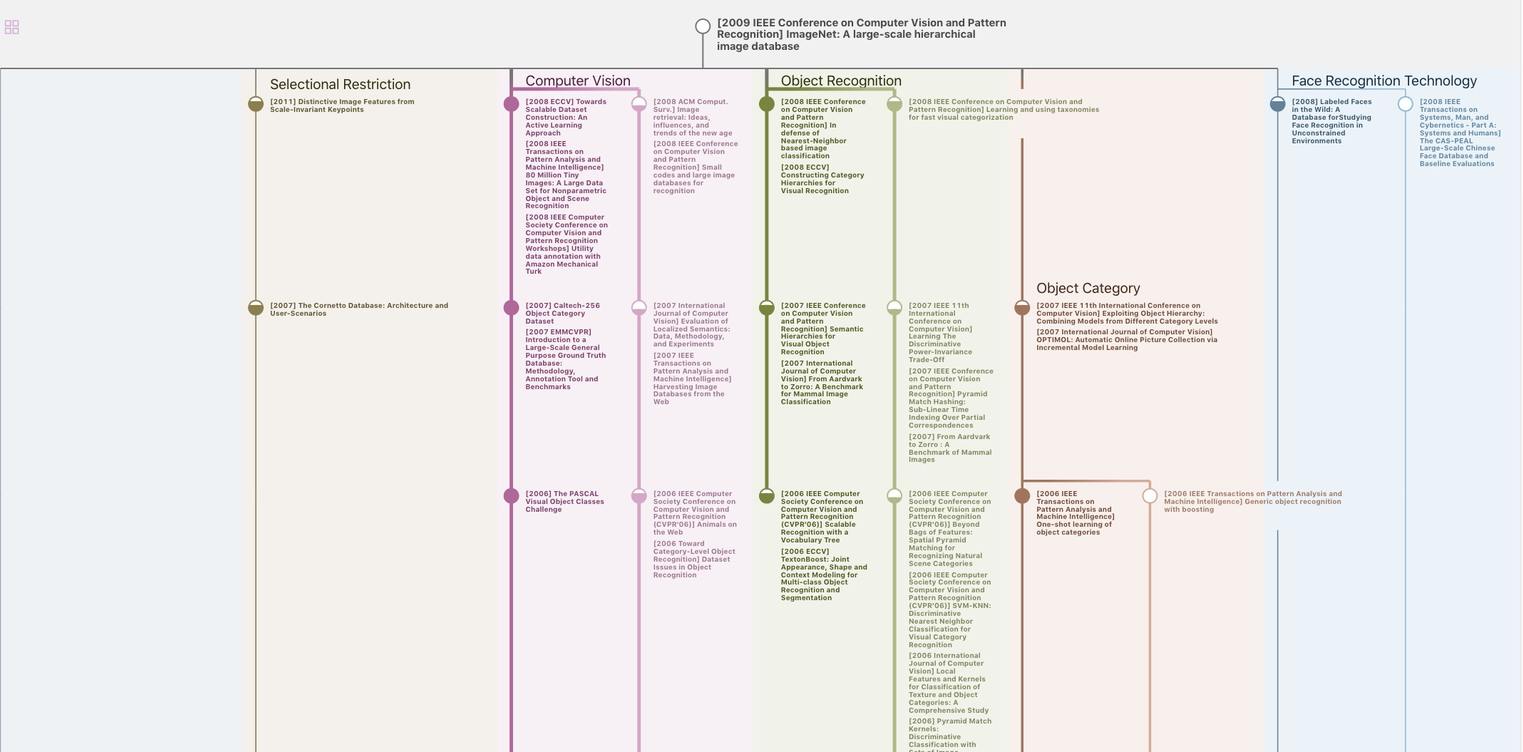
生成溯源树,研究论文发展脉络
Chat Paper
正在生成论文摘要