Network Intrusion Detection in Internet of Blended Environment Using Ensemble of Heterogeneous Autoencoders (E-HAE).
Comput. Syst. Sci. Eng.(2023)
摘要
Contemporary attackers, mainly motivated by financial gain, consistently devise sophisticated penetration techniques to access important information or data. The growing use of Internet of Things (IoT) technology in the contemporary convergence environment to connect to corporate networks and cloud-based applications only worsens this situation, as it facilitates multiple new attack vectors to emerge effortlessly. As such, existing intrusion detection systems suffer from performance degradation mainly because of insufficient considerations and poorly modeled detection systems. To address this problem, we designed a blended threat detection approach, considering the possible impact and dimensionality of new attack surfaces due to the aforementioned convergence. We collectively refer to the convergence of different technology sectors as the internet of blended environment. The proposed approach encompasses an ensemble of heterogeneous probabilistic autoencoders that leverage the corresponding advantages of a convolutional variational autoencoder and long short-term memory variational autoencoder. An extensive experimental analysis conducted on the TON_IoT dataset demonstrated 96.02% detection accuracy. Furthermore, performance of the proposed approach was compared with various single model (autoencoder)-based network intrusion detection approaches: autoencoder, variational autoencoder, convolutional variational autoencoder, and long short-term memory variational autoencoder. The proposed model outperformed all compared models, demonstrating F1-score improvements of 4.99%, 2.25%, 1.92%, and 3.69%, respectively.
更多查看译文
关键词
intrusion detection,heterogeneous autoencoders,ensemble,blended environment,e-hae
AI 理解论文
溯源树
样例
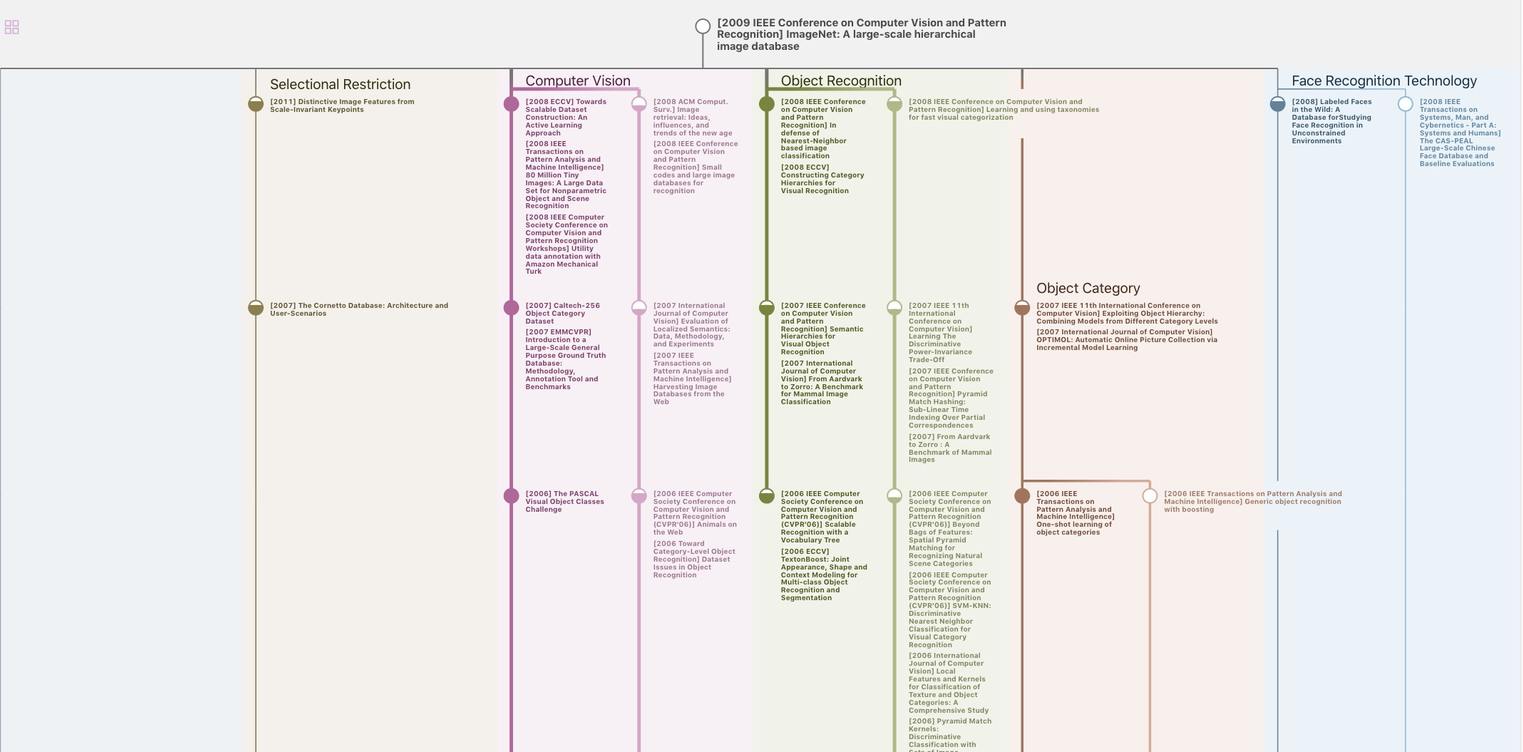
生成溯源树,研究论文发展脉络
Chat Paper
正在生成论文摘要