A Computationally Efficient Robust Cubature Kalman Filter With Multivariate Laplace Distribution.
IEEE Trans. Instrum. Meas.(2023)
摘要
This article investigates the nonlinear state estimation under heavy-tailed process and measurement noises (PAMNs), and the noises may be induced by sensor failures, measurement loss, modeling errors, environmental changes, or malicious cyberattacks. Considering that the multivariate Laplacian (ML) distribution has obvious heavy-tailed characteristic, we employ the distribution to describe heavy-tailed PAMNs. Furthermore, to improve the computational efficiency of the existing variational Bayesian (VB) iteration process, we design an improved VB iteration method, which can separately calculate the posterior distributions of the state vector and unknown noise parameters. Employing the ML distribution and improved VB inference process, a computationally efficient robust cubature Kalman filter (CEMLRCKF) is derived. Simulation and vehicle experimental results illustrate the superiority of the proposed filter.
更多查看译文
关键词
Multivariate Laplacian (ML) distribution, nonlinear filter, outlier-contaminated measurements, variational Bayesian (VB) inference
AI 理解论文
溯源树
样例
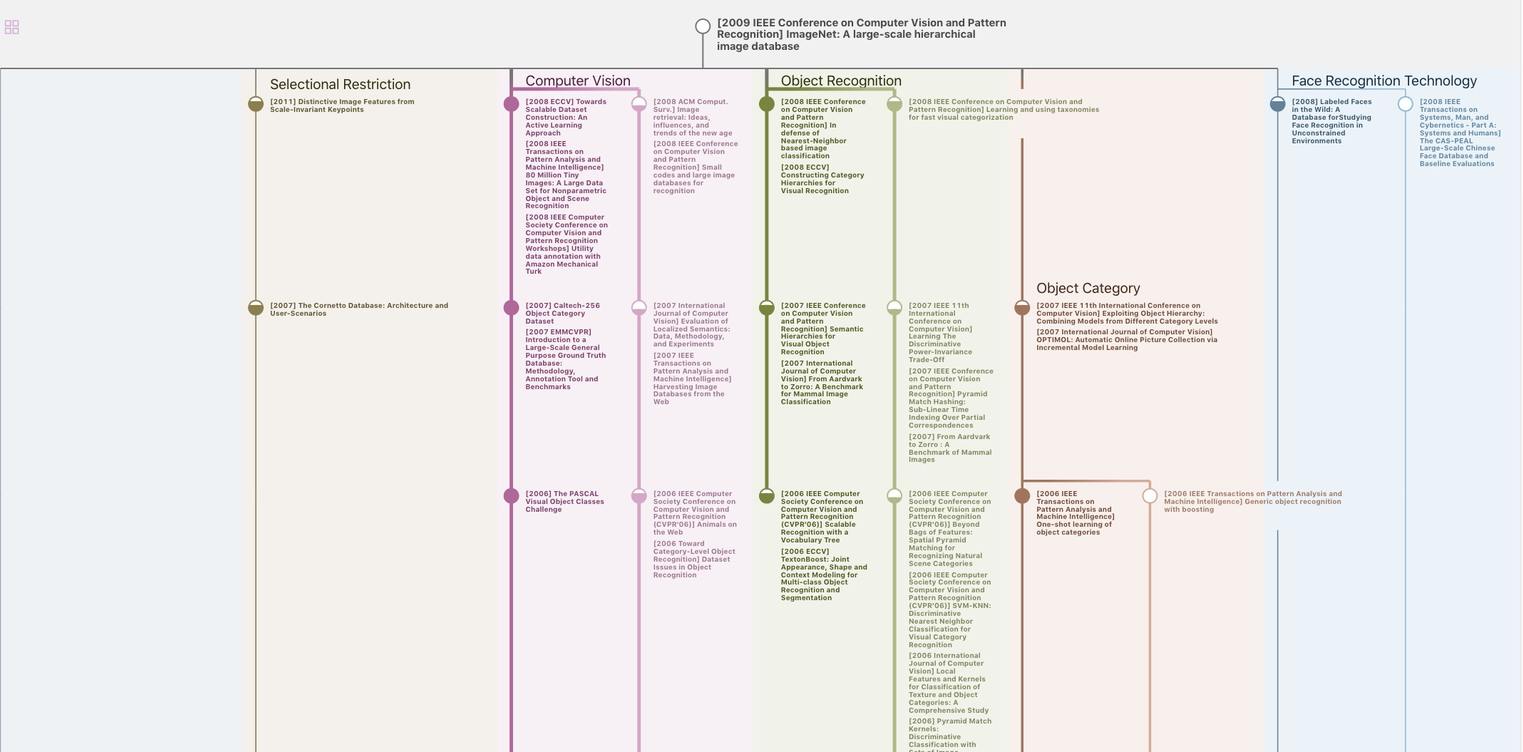
生成溯源树,研究论文发展脉络
Chat Paper
正在生成论文摘要